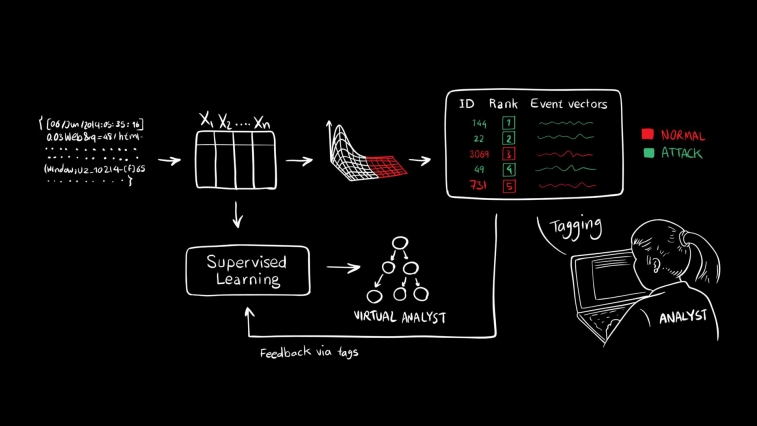
Researchers from MIT’s Computer Science and Artificial Intelligence Laboratory (CSAIL), and a machine-learning startup, PatternEx, have developed a virtual artificial intelligence analyst that can predict 85% of cyber-attacks using input from humans.
As a way to address some of the challenges security analysts face, researchers from CSAIL and PatternEx presented a paper on a new artificial intelligence platform called AI2 They found that their system is able to detect cyberattacks more frequently because it is continuously incorporating input from human analysts.
(Related: Companies team up on e-mail security)
There are several problems that currently exist in today’s state of cybersecurity. One major problem includes the lack of qualified security analysts in the market. It is critical to increase analyst efficiency, but existing tools generate too many false positives that create distrust and need to be investigated by humans in the end, said Ignacio Arnaldo, one of the researchers at PatternEx.
He said that the type of security systems that exist today fall into two categories: human or machine. An “analyst-driven solution” relies on rules created by the human experts, which means the system could miss attacks that do not match the rules they’ve created, he said.
“On the other hand, purely machine learning approaches rely on ‘anomaly detection,’ which tends to trigger false positives that both create distrust of the system and end up having to be investigated by humans anyway,” said Arnaldo. “Our system aims to minimize the number of both false positives and false negatives.”
The team showed that AI2 reduced the number of false positives by a factor of 5. According to the researchers’ paper, they were able to demonstrate the system’s performance by monitoring a Web-scale platform that generated millions of log lines a day over a period of three months, for a total of 3.6 billion log lines.
The system is like a virtual analyst, but the human component is very necessary at this point, said Arnaldo. “The system is aimed at making more efficient use of human expertise and freeing analysts from having to comb through massive amounts of cybersecurity data,” he said.
Reviewing flagged data to determine validity is a time-consuming task for security experts, so AI2 brings in three machine-learning methods to determine events for the analysts to label. Then, using what the team called a “continuous active learning system,” AI2 builds a model that can refine its ability to separate cyberattacks from other activity, reducing the number of events it needs to look at each day.
The three outlier-analysis methods can be broadly described based on density, matrix decomposition and replicator neural networks, and these three methods are considered the system’s “secret weapon.”
The next step for the team is to build a network of organizations that share information on malicious patterns, said Arnaldo. This will improve analyst efficiency since investigation outcomes fed back to the system will benefit many of the organizations.
“Imagine that the moment a malicious pattern is discovered at one organization, other organizations subscribed to the network will automatically be prepared to defend against it,” said Arnaldo.