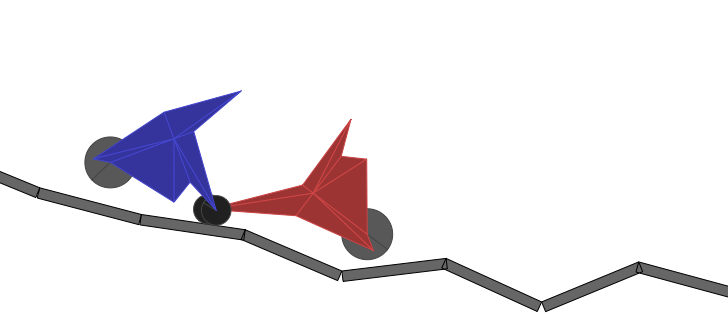
You could be forgiven for thinking that this past week yielded the discovery of some new type of AI, but watching Elon Musk donate US$10 million to the Future of Life Program would lead one to believe that. Musk donated these funds specifically to push for research designed to keep artificial intelligence friendly.
The Future of Life Institute was founded by one of the creators of Skype, who said that building AI is like controlling a rocket: You concentrate on acceleration first, but once that’s accomplished, steering becomes the real focus.
But Musk wasn’t the only one focusing on futuristic AI this week. Deep learning got a big boost from bloggers and developers, as well. Two larger pieces on the state of deep learning went live.
Particularly interesting is the use of AI and data visualization to imagine higher-level dimensional data. And in the latter link, a podcast on machine learning, specifically tackles the ethical questions, again.
Does this mean we need three rules for AI, like Asimov’s robots? 2015 might be the year we finally tart laying some ethical guidelines for AI. But looking more deeply into what machine learning actually entails, Christopher Olah’s blog postings on the topic are truly enlightening. He dives deeply into the ways neural networks function to try and help us understand what, exactly is going on inside these mysterious learning programs.
While reading his pieces on the topic, all of which are magnificent, I was struck by just how much learning algorithms can be understood by outputting visuals of their inner workings. Olah does a great job of this, representing the hidden intermediate steps of a neural network’s reasoning in ways that show how it is going about its rationalizations.
This phenomenon is exemplified in some interesting ways on the Web, though not quite as obliquely. My favorite example is Genetic Cars. While this is an evolutionary algorithm rather than an AI algorithm, it’s still of similar importance. The program generates possible solutions to a simple question in a confined problem space. Here, instead of performing image recognition, it’s just building cars and seeing how far they can get on a random track.
One has to wonder if human reasoning works in a similar way, or in a completely different way. The scientific method would dictate that we’re relatively similar: trial and error, ruling out the inferior, and eventually yielding a solution.