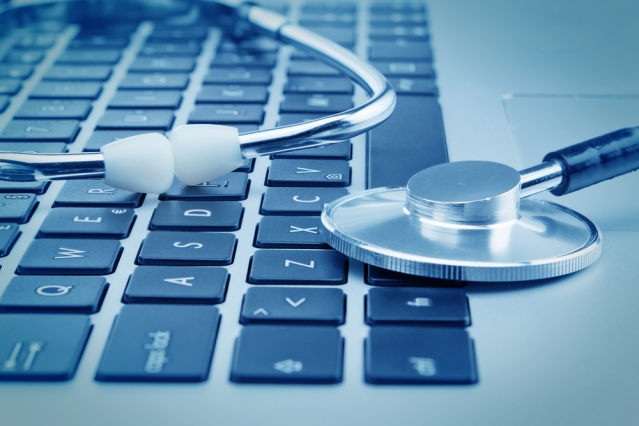
Cancer is a scary thing, but it can be even scarier if doctors can’t properly diagnose the specific type of cancer a patient has. Researchers at MIT’s Computer Science and Artificial Intelligence Laboratory (CSAIL) want to solve this problem with a program that they said can help doctors better identify the disease.
The researchers specifically looked into improving the diagnosis of lymphoma, a cancer that has more than 50 subtypes. According to Ephraim Hochberg, director of the center for lymphoma at the Massachusetts General Hospital, 5% to 15% of lymphoma cases are misdiagnosed or misclassified, which can lead to difficulty in treating the disease.
“As pathology advances, what previously constituted one cancer category is now often regarded as multiple diseases or even a spectrum of diseases,” said Yuan Luo, Ph.D. student at CSAIL. “Lymphoma diagnosis, as a particular example, is seeing continuous knowledge evolution, and has been a difficult task and a source of debate for pathologists and clinicians.”
The problem, according to Luo, arises when a doctor tries to diagnose a patient. The physician has to refer to dozens of similar cases and guidelines, and that can be a labor-intensive task. “The amount of patient data exceeds the capacity of human experts,” he said.
To address this problem, Luo, along with Peter Szolovits, professor at MIT’s CSAIL, other CSAIL team members and a team from Massachusetts General Hospital, have built an interpretable computational model designed to automatically recommend cancer diagnoses after analyzing a collection of data and patient records. While Luo noted that there have been machine-learning models that have been just as accurate, they do not provide a way for clinicians to interpret the model.
“Often software developers build a machine-learning pipeline that has pretty good accuracy, but you hear domain experts (in our case, pathologists) complaining that they don’t understand what the model is doing,” he said. “Our method tries to bridge the interpretability gap. It can automatically capture relations between concepts.”
The model is able to improve a doctor’s diagnosis of lymphoma by suggesting subtypes based on cases it examines and providing a summary of the data for experts to confirm.
“By finding better descriptions of commonalities among lymphoma subtypes, it can help improve our understanding of lymphoma, and by learning diagnostic criteria from many past cases, it can help advise medical experts on the most likely disease in a specific patient,” said Szolovits.
In the future, the researchers plan on advancing the model by making it more interactive “so that doctors can teach the program by adding/removing test results to the proposed panel,” said Luo.
More information is available here.