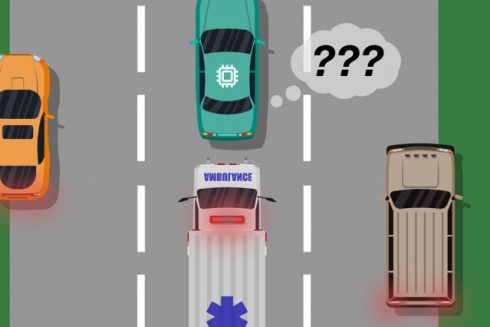
As artificial intelligence is being implemented into advanced systems like self-driving cars and autonomous robots, MIT and Microsoft researchers want to make sure they are covering all their bases. The researchers are tackling what they call “blind spots” of artificial intelligence to provide better security and safety of AI-implemented systems.
While systems like self-driving cars are being extensively tested to prepare for nearly every possible event, researchers worry how these cars will react when unexpected real world errors occur. For instance, if a car isn’t trained on the difference between large white cars and ambulances with flashing red lights, it may not automatically slow down or pull over.
To provide better insights into these blind spots, the researchers have created a new model that takes the traditional approaches such as testing AI through simulation training and adds human input into how the AI worked in the real world and where it can improve. The training data and human feedback is combined with machine learning techniques to pinpoint where systems need to focus more attention on.
“The model helps autonomous systems better know what they don’t know,” said Ramya Ramakrishnan, a graduate student in MIT’s Computer Science and Artificial Intelligence Laboratory (CSAIL). “Many times, when these systems are deployed, their trained simulations don’t match the real-world setting [and] they could make mistakes, such as getting into accidents. The idea is to use humans to bridge that gap between simulation and the real world, in a safe way, so we can reduce some of those errors.”
In addition, the researchers explained while there are traditional training methods that provide human feedback out there, these methods only update the system’s actions instead of identifying the blind spots. With the researchers’ model, the system can also observe how a human reacts in a real-world situation to learn what is steps it could have taken.
“This research puts a nice twist on discovering when there is a mismatch between a simulator and the real world, driving the discovery directly from expert feedback on the agent’s behavior,” said Eric Eaton, a professor of computer and information science at the University of Pennsylvania.