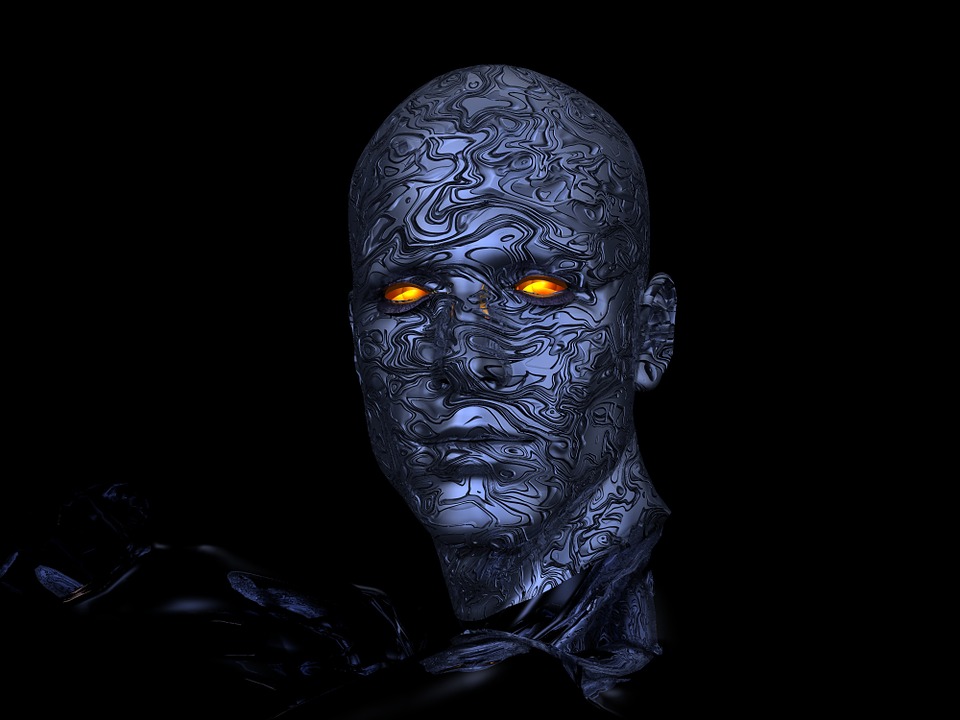
It seems almost comical to think that artificial intelligence and machine learning are becoming, or rather have become, full-blown buzzwords. First of all, according to the annals of science fiction literature, we should have already invented a smarter-than-human AI by now, and according to “Terminator,” we’re 19 years overdue for a giant, AI-based nuclear annihilation of the human race.
1997 was the year pegged in the Terminator series, the year Skynet took over and decided humans were no longer of use. I prefer the 1970 movie, “Colossus: The Forbin Project,” a film that does not appreciate the premise that the United States could, alone, create a computer smart enough to take over the world. Colossus, you see, required the help of its Soviet counterpart.
(Related: Google builds a chip for machine learning)
The world of AI is far more scientific and mundane than this, however. Its history is littered with startups, hardware firms and software projects that were often just as much a product of the times as they were products of machine-learning advances.
All roads lead to Marvin Minsky, of course. But there are other stops along the way, from the Thinking Machines Corporation, to Lisp machines, there have been many attempts to turn computers into “thinking” brains.
The reality, however, is that it’s still all just software underneath. Whether the dataset being used is the product of a human curator, or the product of a deep-learning algorithm and a neural network, it’s still just 1’s and 0’s being pushed through copper and stacked up in registers.
What has really changed in the world of AI in the past 30 years? Many things. But fundamentally, the problem for the… call it a genre… has been the inability to gather enough good data for training, or the lack of speedy enough hardware to enable the so-called brain to be of much use.
Naturally, these problems are remedied by cloud-based Big Data systems. And thus, the sudden interest in AI and machine learning. These are technologies that can now be brought to the enterprise markets.
What form will they ultimately take, however? As I have always done with buzzwords in the enterprise market, I like to compare them to SOA.
Service-oriented architecture is not exactly a smoke-and-mirrors proposition. It’s not like Crocs or the Slanket in its temporary faddish appeal. SOA has fundamental underpinnings that are of great use to enterprises.
And yet SOA, as it existed during the SOA boom from around 2003 to 2007, large software companies couldn’t keep their hands off of registry and repository companies. The Enterprise Service Bus was a thing, and all manner of cart-before-the-horse solutions to enable the SOA migration were flooding the market, and many were even being acquired.
The big words in the SOA market were “Web services standards” and “SOAP”. Everything was XML, and it was all going to be validated through complex security and identity projects.
Today, SOA still exists. Everyone is building services. They’re everywhere. It’s standard. What don’t exist are all the solutions to help with this new world. Instead, they’ve all been recreated from scratch, or are still being recreated.
Why? First of all, it’s because XML and SOAP were awfully heavy. REST was chosen by developers, not by committees or startups or foundations. It was chosen by the actual developers because it was easier and it just worked with the technology that already existed.
So while AI and machine learning are without a doubt the path forward for software to evolve and become more powerful in the future, the current marketplace and the current stream of products that have arisen in this space are highly unlikely to be the right choices for the future.
Instead of being tangled up in a buzzword, this is the time to learn about real AI and machine learning, and to foster your internal center of excellence in fundamentals. Buying AI and machine learning products now is probably not a great bet in the long term.
Especially not when companies like Google and Amazon are betting they can build better-generalized AI for everyone to use than you can build in your own Hadoop clusters.