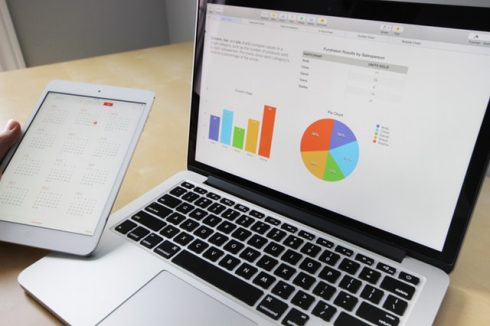
The most challenging obstacle facing companies is recognizing if they have a valid use case requiring the use of Big Data technologies. Having a large amount of data does not necessitate the deployment of a Big Data solution architecture. A company should only determine to use a Big Data solution based on the characteristics and its intended use. This allows actionable insights to be more readily derived.
Other obstacles include the lack of skilled resources to administer and construct the solution, which poses a risk of underestimating the effort to deploy the solution, as well as a sub-par architecture that would lead to performance issues. Two other problems are insufficient governance associated with affected business processes and organizational change management, and misunderstanding the limitations of Big Data technologies and its ever-expanding ecosystem.
Companies must also adjust to new technologies and toolsets that are continuously introduced. Some are very mature, while some are quite nascent. Making sure the correct toolsets are select and deployed can be very challenging. With this, businesses often try to tackle too much too soon – the size of the selected use case may be so large and complex that it never produces any actionable insights in a reasonable amount of time.
Overcoming Big Data obstacles
Make sure that the identified use case is appropriate for a Big Data solution. Highly structured and transaction-oriented data may be better served by a traditional DBMS. Big Data solutions are extremely well-suited for unstructured and semi-structured data. Structured data may also be stored and transformed as required and integrated with unstructured data to provide richer insights.
Companies should not skimp on training or hiring. Having skilled resources is critical for the timely delivery of Big Data solutions that are efficient and meet consumers’ analytic requirements. This should include understanding the Big Data ecosystem and how tool sets are related to specific use cases as well as to each other. This will lead to the right tool for the right job.
Businesses should also focus on governance and the definition of processes for security, data storage, movement and the deployment of analytic artifacts. This will ensure that discovered insights remain relevant to the identified use cases and target audiences. This cannot be overemphasized: keep it simple, but challengin, meaning the use case should be manageable. Capabilities should be defined that can be delivered in 30-60-day windows (otherwise, interest will be lost). Select a use case that is fairly well-understood. This will provide continuous delivery of capabilities, allow easier validation of results and provide a learning opportunity.
Consider leveraging cloud-based solutions. Cloud vendors have solutions that can be deployed very quickly (minutes to hours). These solutions can provide dynamic scaling and/or scaling on-demand. When analytic requirements change, the solution can be scaled up as required allowing for more complex and deep insights (data visualization, machine learning).
Finally, if using a dedicated analytics tool, make sure that tool has native Big Data integration. This will facilitate the development of self-service analytics and insights and lead to an agiler decision-making process.
The future of Big Data
The future of Big Data is strong and fast from a growth perspective:
- New types of data are constantly appearing: Big Data tools and technologies are evolving to keep up. For example, data associated with virtual assistants and AI (Artificial Intelligence) applications is growing at an astronomical rate. New ways of storing and retrieving this data are always being developed.
- It is becoming easier to develop, deploy and interact with Big Data solutions. This indicates that the barriers to entry to the world of Big Data are being reduced.