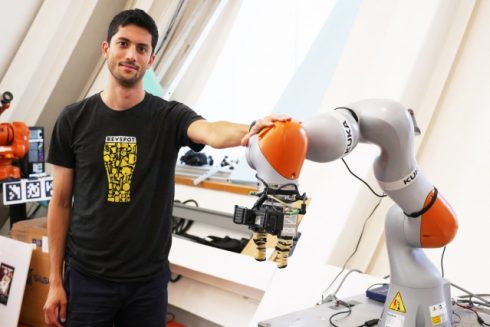
Researchers from MIT’s Computer Science and Artificial Intelligence Laboratory (CSAIL) have announced a breakthrough in their robotics research. The team is presenting the Dense Object Nets (DON) method, a new system that they say will one day make robots useful in home and office situations.
The system uses computer vision to enable robots to understand and manipulate objects as well as find and detect an item in clutter. According to the researchers, this type of dexterity has only been available to humans, until now.
VIDEO: https://www.youtube.com/watch?v=OplLXzxxmdA
“Many approaches to manipulation can’t identify specific parts of an object across the many orientations that object may encounter,” said Lucas Manuelli, PhD student and one of the researchers of the project. “For example, existing algorithms would be unable to grasp a mug by its handle, especially if the mug could be in multiple orientations, like upright, or on its side.”
The researchers believe this type of system would be beneficial to companies like Amazon and Walmart who have multiple items in their warehouses. The robots will also be able to successfully grab specific parts of an object without ever having seen the object before such as a shoe and the tongue of that shoe, the researchers explained.
In addition to manufacturing use cases, the researchers see this type of system being successful in a home environment for uses such as cleaning up a house or putting dishes away.
While robot grasping has been researched extensively throughout the industry, the MIT CSAIL researchers explained their approach uses self-supervised learning while common approaches focus on task-specific learning or general grasping algorithms. According to the researchers, task-specific learning makes it hard to generalize other tasks and general grasping isn’t specific enough.
The DON system differs in that it provides a “series of coordinates on a given object” similarly to a visual roadmap in order to help the robot understand.
“In factories robots often need complex part feeders to work reliably,” said Pete Florence, PhD student and lead author of the team’s paper. “But a system like this that can understand objects’ orientations could just take a picture and be able to grasp and adjust the object accordingly.”