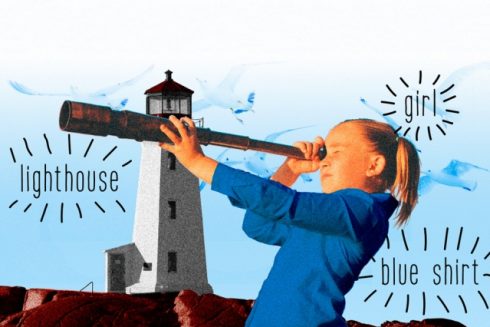
Researchers at MIT have developed a new system that combines voice and object recognition capable of identifying an object within an image given only a spoken description of that image.
When provided with an image and audio caption, the system is able to highlight the relevant regions of the image being described, in real-time.
Current models require manual descriptions and annotations of the examples that it is trained on. This system learns words directly from clips of recorded speech and objects in raw images, associating them together.
“We wanted to do speech recognition in a way that’s more natural, leveraging additional signals and information that humans have the benefit of using, but that machine learning algorithms don’t typically have access to,” David Harwath, a researcher in the Computer Science and Artificial Intelligence Laboratory (CSAIL) and the Spoken Language Systems Group, said. “We got the idea of training a model in a manner similar to walking a child through the world and narrating what you’re seeing.”
Right now the model can only recognize several hundred different words and object types, but researchers hope that one day this model will be able to open new doors in speech and image recognition and reduce the time spent on manual labor.
According to MIT, one of the promising applications of this model is learning translations between languages without needing a bilingual annotator. Of the 7,000 languages useds around the globe, only about 100 of them have sufficient transcription data for speech recognition.
However, in a situation where speakers of two different languages describe the same image, the model can learn the speech signals from both languages that correspond to objects in the images and then assume that those two signals are translations of one another, the researchers explained. “There’s potential there for a Babel Fish-type of mechanism,” Harwath said, referring to the fish that provides instant translations of different languages in Douglas Adams’ “The Hitchhiker’s Guide to the Galaxy” series.
“It is exciting to see that neural methods are now also able to associate image elements with audio segments, without requiring text as an intermediary,” says Florian Metze, an associate research professor at the Language Technologies Institute at Carnegie Mellon University. “This is not human-like learning; it’s based entirely on correlations, without any feedback, but it might help us understand how shared representations might be formed from audio and visual cues.