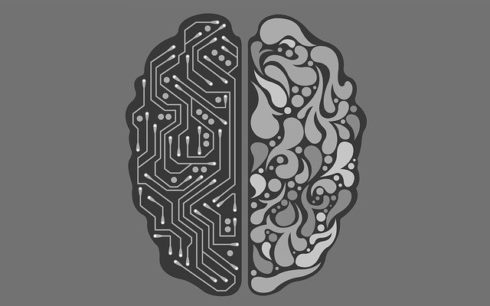
Organizations are moving AI and ML up their strategic priority lists and investing more in the technology without yet fully knowing how to turn those investments into increased efficiency and scale, according to the “2021 enterprise trends in machine learning report” from Algorithmia.
Approximately 83% of all surveyed organizations said they increased their budgets and that the number of data scientists working for them has increased 76% year-on-year. The most common use cases for the technology are for customer experience and process automation.
Despite the increased budgets, organizations are spending more time on model deployment as the time required to deploy a trained model to production keeps increasing every year. 64% of all organizations take a month or longer to deploy a model.
About 38% of data scientists are spending more than half of their time on model deployment and more models at an organization equal more time spent despite the vast functionalities that the technologies offer.
“The bottom line is, organizations have increased their AI/ML resources without solving underlying challenges with operational efficiency. This has exacerbated the problem and led to organizations spending more time and resources on model deployment,” Algorithmia wrote in the report.
The company that put together the report, Algorithmia, is machine learning operations (MLOps) software that manages all stages of the ML lifecycle, including ML deployment, collaboration between operations and development, and security.
There are still major concerns when it comes to implementing the technology. 67% of all organizations report needing to comply with multiple regulations for their AI/ML as their most common concern. Meanwhile, 56% of organizations ranked governance, security and auditability as a concern.
Basic integrations continue to be a concern as cross-functional alignment remains a roadblock for achieving AI/ML maturity.
However, there is a light at the end of the tunnel as organizations see improvements in efficiency when they implement third-party machine learning operations (MLOps) solutions.
According to the report, these solutions save data scientists about 22% of their time and the average time to put a trained model into production goes down by 31%.
“COVID-19 has caused rapid change which has challenged our assumptions in many areas. In this rapidly changing environment, organizations are rethinking their investments and seeing the importance of AI/ML to drive revenue and efficiency during uncertain times,” said Diego Oppenheimer, the CEO of Algorithmia. “Before the pandemic, the top concern for organizations pursuing AI/ML initiatives was a lack of skilled in-house talent. Today, organizations are worrying more about how to get ML models into production faster and how to ensure their performance over time. While we don’t want to marginalize these issues, I am encouraged by the fact that the type of challenges have more to do with how to maximize the value of AI/ML investments as opposed to whether or not a company can pursue them at all.”