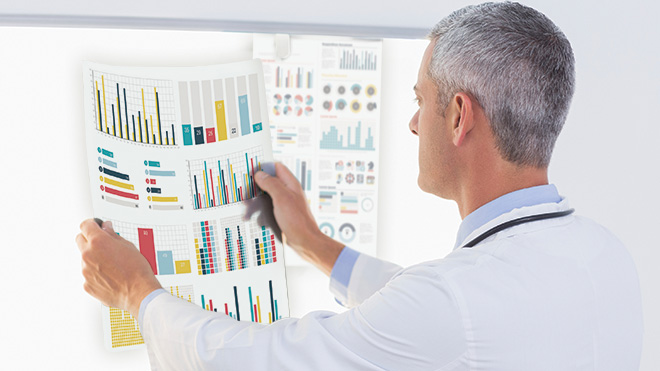
Companies are collecting, generating, storing and analyzing more data than ever before to improve their competitive standing. However, organizations vary significantly in their ability to leverage information across the enterprise and even between departments such as marketing and sales. All too often, available data, reports and analytics aren’t being leveraged as effectively as they could be. Here are a few of the common pitfalls enterprises fall victim to and best practices for driving more value from your company’s data.
Start with the use case
Technology is changing so rapidly, it’s hard for the average enterprise to keep up. As the hype around Big Data, reporting and analytics continues to increase, organizations are often tempted to take a technology-first approach to problem-solving that is less efficient than it might be.
“If you start by purchasing technology, then you’re putting the cart before the horse,” said Traci Gusher, managing director of data and analytics at strategy consulting firm KPMG. “Start with what are my use cases, what are the skills I’m going to need to execute those uses cases, what value do I want to drive out of those use cases, and then look for the technologies that can support that.”
(Related: How Big Data is handling the expanding needs of enterprises)
Many organizations have multiple reporting and analytics solutions in place that are solving department or business-unit-level problems. On one hand, the reporting and analytics options are getting more sophisticated, providing more granular views of customers, situations and assets, for example. At the same time, there is interest in liberating information outside of its traditional silo so it can be used at a cross-functional level to derive additional benefits.
“If a client comes to us and says they want to build an analytics strategy and road map, or [to] understand how they’re using [analytics] today, we talk to all the relevant business units and do an inventory of their analytics activities,” said Christer Johnson, principal of advisory services at professional services company Ernst & Young. “Not all customers are willing to go down this path, but the ones that do get better results.”
Despite the availability of more and better BI and analytics tools, business leaders may nevertheless suffer from inaccurate financial or demand forecasts because their organizations still lack processes that would enable them improve the accuracy of those forecasts.
“We still see people relying on their experience and intuition all the time,” said Chris Jennings, vice president of technology services at strategic technology consulting firm Collaborative Consulting. “We talk to the business and they tell us what’s going on, but when we go behind the scenes to try to prove it, what they told us isn’t true. Before you even go out and look at the business processes and the systems that support those business processes, you need to understand the business.”
Prioritize work
Reporting and analytics capabilities are being included in more types of software, so individuals and businesses can measure their performance and optimize outcomes. To enable a sound enterprise strategy, Capgemini gathers the stakeholders from its various operating units together to understand their analytics requirements from an operational standpoint. Capgemini then has each department come up with a Top 10 list of what they want to achieve.
“We start off trying to understand what the prioritized needs of the business units are and then we work with the IT or data groups within the organization to try to understand the pieces of data that would be required,” said Goutham Belliappa, business information management data integration and reporting practice leader at Capgemini. “We do it by business unit and operating unit to understand the commonality which informs what we want to ingest in the data lake or data ecosystem, and then we start delivering outcomes on the road map.”
The level of business value often determines what projects receive higher or lower priority, but many organizations are still struggling to tie analytics to business outcomes. In a recent Forrester Research survey of enterprise architects, 74% of respondents said their organizations aspire to be data-driven, but only 29% said they’re good at translating analytical results into measurable business outcomes.
“When we work with organizations, we talk about the use cases that are going to drive value for you—things you could or should be doing that would drive value for your organization,” said KPMG’s Gusher.
KPMG conducts “idea generation workshops” in which the biggest business opportunities and challenges are discussed along with whether or not Big Data and advanced analytics address those opportunities and challenges. The point is to generate a list of use cases that have estimated values attached to them.
One of Ernst & Young’s clients refuses to proceed with a project unless it’s able to identify how it’s going to measure the success of the project in dollars and cents.
“We’ve helped them execute 220 projects over the last four years,” said Ernst & Young’s Johnson. “They’ve documented that those projects have created US$1.3 billion of value, and all of those projects are tied to business decisions. They also keep a pipeline of projects with estimated values they think they’ll create. If projects can’t get tied to value, they’re deprioritized.”
Understanding what exists
The entire scope of data, reporting and analytics being used may well be unknown in an organization. Sometimes data is available, but the people (or some of the people) who need access to it can’t get access to it, or it may be underutilized or unused for whatever reason.
“Doing an inventory of your data requires you to understand what’s in the systems of record and that you bring them together, so you leverage all of them for the relevant insights you’re looking for,” said Jennifer Belissent, principal analyst at Forrester Research.
Forrester often recommends companies inventory their data assets by looking at the systems of record and the data generated by those systems such as data coming out of financial systems, asset-management systems, ERP, CRM, service records, sales transactions, social media, inventory tracking, and supply chain management.
Capgemini, like some other consulting organizations, has automated tools that can inventory reporting and analytics assets and the surrounding ecosystem. Using those tools, it can determine how many people have access to a system and how often they’re using it, which provides clues for necessary improvements.
“The problem with [automated discovery] is that all outcomes are treated as if they have the same level of priority, so it’s important to have not just a mechanical understanding of what exists but the gaps that need to be filled to move the organization to a higher level of maturity,” said Capgemini’s Belliappa.
Talking to people is also important, since members of a department will tend to know which tools it uses, for what purposes, and the shortcomings of the systems. However, the entire scope of investments may not be clear because some things may have been lost, forgotten or overlooked.
“In BI management, there’s the ability to build a data dictionary, do MDM and so forth,” said Andrew Brust, senior director at Big Data platform provider Datameer. “In the Big Data world, it’s a little harder to be methodical and straightforward. There’s a lot of detective work, because it’s not simple discovery. It ends up being kind of an exercise of enumerating and inventorying and then drilling down into those things.”
Many organizations have a pretty good understanding of the management reports that are being generated, but there’s also still a lot of data trapped in spreadsheets.
“If the organization understands what information is critical and needed by which constituencies, then it becomes a matter of understanding whether we have it and getting it to the right place, so there is management of the data that needs to take place so there’s a view of that,” said Dan DiFilippo, global and U.S. data and analytics leader at PricewaterhouseCoopers. “Otherwise, you end up with a situation where you don’t realize you had it and it would have been great if we knew that.”
Some IT groups have documentation describing the complete architecture and technology landscape, which can aid the understanding of what technologies are in place, and which ones contain transactional data, master data, and other data. Sometimes the entire scope of external data sources may not be apparent, since it’s easy for departments to get access to such data without IT’s help or involvement.
“Understanding the technology landscape and architecture is one way of determining what data you have. The other is ascertaining what data, dashboards, reports and KPIs are being used, which can also help identify what data exists and what data is being used,” said KPMG’s Gusher.
But before data is inventoried for its own sake, organizations should think first about what they’re trying to accomplish, and what data, reports and analytics they’ll need to accomplish those goals.
“Before you start thinking about inventorying your data, you need to inventory all the key decisions to need to make and prioritize those to determine what data may be relevant to those decisions and whether you can get access to the data,” said Ernst & Young’s Johnson. “Time and time again, clients have taken a data-first approach to itemize the data they have. They’ve wasted time and energy because they haven’t been able to drive any new, informed decision at that point.”
Consider a center of excellence
Not every company has the resources to establish a formal center of excellence (COE), but those that have one are in a better position to help individual operating units and the enterprise as a whole because they have an enterprise-wide view of what’s used, who’s using it, and for what purposes. A COE can help eliminate redundant efforts and can help fuel the adoption of best practices throughout the organization.
“People with sophisticated analytics are moving toward a COE,” said Shawn Rogers, chief research officer at predictive analytics software provider Dell Statistica. “I believe for larger companies with diverse reporting and analytics landscapes, the only way to do it is to establish a COE and assign personnel to become the enabler of insights for the entire company.”
COEs can help identify the data-related investments that have been made and the gaps that exist, as well as democratize relevant capabilities that may be popular in one department but completely foreign to another.
“IT has produced a lot of this stuff, but once it’s produced, they don’t have the manpower to go back and examine whether or not it’s being used,” said Rogers. “COEs help ensure that you’re not building stuff that’s not consumed, and they can help you maintain focus on the smartest and most impactful things you need to do for the business. I’ve been in too many meetings where one person says it would be great to have a certain capability and another says his department has been doing that for the last three years.”
Some companies, particularly large companies, have also appointed a Chief Analytics Officer (CAO) and/or a Chief Data Officer (CDO), whose reporting structures and responsibilities tend to differ across industries and organizations. The CAO tends to focus on driving business insights through analytics, whereas the CDO typically focuses on governance—although the responsibilities can vary from company to company. In some organizations, a CDO’s role may evolve to include CAO responsibilities after an appropriate foundation has been established. Alternatively, one person with a CDO, CAO or other title may be responsible for both areas.
“The organizations I’ve seen do this best have a strong CDO and a strong CAO who work very well together,” said Ernst & Young’s Johnson.
The chicken and egg dilemma
Effective decision-making needs to be based on reliable data, but the desire for timely insights and the time it takes to ensure data quality can often work against each other. In the past, organizations built data warehouses and data marts to store their enterprise data, but to lower storage costs and to take advantage of massive volumes of unstructured data, they’ve been adopting newer technologies—including Hadoop and Spark.
Some companies are building massive data lakes and throwing all possible data into it, hoping it will help produce some valuable insights in the future. The problem with that approach can be poor data quality, which can be difficult and costly to rectify later. On the other end of the spectrum are companies that are so focused on data quality, they’re impeding their own progress.
“If you have access to data today, storing it in a data lake is cheap enough that you’d be foolish not to do it,” said Johnson. “Obviously there may be some data you don’t need to keep, but for the most part, it’s so cost-efficient to store it, you store it and label it so when people do analytics it’s fine.”
On the other hand, just because the data is available doesn’t necessarily mean the organization will use it. For example, one of Ernst & Young’s healthcare clients built a model describing how long it retained customers. With Ernst & Young’s help, the company came up with 150 variables, but it identified some external data that it had excluded because the company didn’t consider the data relevant to the problem. Since the data-mining techniques could process 282 variables as fast as 150 variables, Ernst & Young convinced the client to use both internal and external datasets. Of the 282 variables, 20 were highly correlated with customer retention, and of those 20, 12 came from the external dataset.
“The only reason they didn’t know the data was relevant was because they weren’t using the appropriate analytical techniques,” said Johnson. “Now they have 12 variables that are external to their own system they can apply to [customer acquisition] rather than just looking at existing customers.”
Progress DCI has seen some of its customers take some of their old legacy systems or on-premise enterprise systems and build a data lake that contains a whole history of information, including lower-value data coming in from the core systems.
“You can build your data lake quickly and let data scientists and data modelers define some kind of analytics, or they can do some data science-type programming and build some statistical models,” said Sumit Sarkar, chief data evangelist at Progress DCI. “You can put all the data you think has business value in a data lake and let your data science team decide what to do with it.”
Don’t overlook data quality and data governance, though. Otherwise the data lake may become a data swamp that gets increasingly difficult to manage over time.
“If an organization is bringing in all their data, then they’re going to have the information available to ask lots of questions,” said KPMG’s Gusher. “The problem is if you haven’t taken the right data management approaches—metadata tagging, lineage, policies, data dictionaries, etc.—then what you have is a data swamp and it won’t provide value, so putting data under governance is imperative. If you don’t do that, you might as well not bring the data together.”
Verifying you’re on the right track
Companies realize they need to actively invest in reporting and analytics capabilities, but it isn’t always clear whether the data strategy, reporting, analytics, or even business processes are what they need to be. Technology, business environments and end-user expectations are changing rapidly. To keep pace, organizations need to be more agile than they’ve been historically, and they need the fortitude to improve what’s working and deemphasize that which is not providing business value.
“People often ask me where to start. I always say [to] look for something that’s critical to your business and optimize it. Don’t go off and start some brand new, glorified project,” said Dell’s Rogers. “Set goals, milestones and metrics to measure success along the way.”
While it’s important to have a plan in place that includes goals, strategies, tactics and a timeline, the definition of success tends to shift over time. Rather than embarking on large, expensive projects that take years to complete, organizations are phasing deployments to deliver stages of capabilities at a certain cost and in a certain timeframe, adopting agile methods that allow for experimentation, validation, and refinement—or both.
“Saying you’re going to drive value out of your Big Data initiative the first year may or may not be reasonable given your organization and focus,” said KPMB’s Gusher. “It may be a three- to five-year road map to value. It depends on the organization and what it’s trying to achieve.”
Prototyping can help organizations avoid time and cost overruns that may otherwise be caused by expensive, long-term endeavors that fail to meet the needs of the end customers.
“The biggest roadblock to companies getting value out of data and analytics is the inability to identify the decisions that are most critical to a company’s success,” said Ernst & Young’s Johnson. “A lot of people say ‘I don’t want to talk about advanced analytics because I’ve got to get my data straight first.’ Three years later, they’re still trying to perfect the data warehouse.”
In short, companies need to be able to do more intelligent things with their data. While having the right technology in place helps, it doesn’t guarantee that a company’s data strategy will be successful. Ultimately, though, reporting and analytics exist to improve business performance, whether that’s increasing customer satisfaction or reducing the number of equipment failures.
Although solving departmental or business unit challenges can be difficult, enterprise undertakings are more complex and are not necessary in all circumstances. Working backward from desired business outcomes is the most straightforward way to determine the data, reporting and analytics that are necessary, and at the same time, companies need to be nimble enough in their approaches to manage change effectively as circumstances, market requirements, customer demands, and business objectives shift.