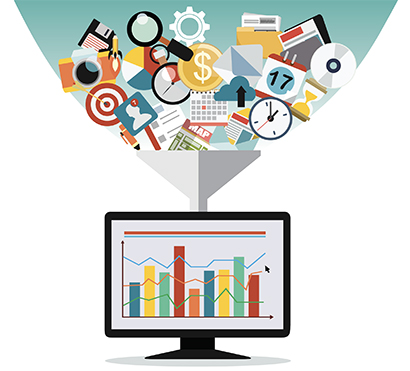
As digital transformation initiatives have picked up steam due to the coronavirus pandemic, companies are getting a lot more disparate data that needs to be put together. They’re looking towards data integration solutions to streamline that process.
A survey conducted by IDC in December last year found that 94% of data engineers, data integration specialists, data stewards, chief data officers are integrating up to five types of data.
This integration process begins with ingestion process, and includes steps such as cleansing, extract, transform, load (ETL) mapping, and transformation. Once complete, the data integration ultimately enables analytics tools to produce effective, actionable business intelligence and compiles it all into a single, unified view.
RELATED CONTENT:
How does your solution help customers with their data integration initiatives?
A guide to data integration tools
Without unified data, a single report typically involves logging into multiple accounts on multiple sites, accessing data within native apps, copying over the data, reformatting, and cleansing, all before analysis can happen, which can be very time consuming for data scientists and developers to set up.
One major factor that necessitates an effective data integration strategy is the fact that there are many different types of data that an organization ingests.
The data can come from mainly three different sources, according to Sameer Parulkar, the product marketing director for Red Hat Integration. One is data that is stored in traditional ERP systems, data warehouses, or data lakes. Another is data in motion, which can come from millions of devices, different customer touchpoints and engagements points as well as physical stores. Last is data in action, which is generated by developers, data scientists and architects to develop applications or services.
“All this data needs to be collected, aggregated, managed, and stored, but there are different data formats. There are different data definitions across different touch points, across different data sources. All of this has to be reconciled in one way or another in a secure way. What about the data quality? All of these are important elements and common pain points with data integration,” Parulkar said.
The various forms of data are also being managed across many different types of data management technologies.
At the top of the list are spreadsheets and relational databases. Other common types are analytical databases and mainframes, according to Stewart Bond, the research director of IDC’s Data Integration and Intelligence Software service.
“Data lakes are surprisingly at the bottom of the list, but the bottom is still over 50% of people who responded to the survey back in December,” Bond said. “I had to answer a lot of inquiries about whether data lakes are going to kill the need for data integration? And no, it doesn’t kill it at all. You still have all those different types of data being stored in that one place. You still need to integrate that data to make any sense of it.”
Meanwhile, gathering all of the data is only one side of the coin, according to Bond. It’s also about understanding that data to make the most use out of data integrations.
“A lot of data integrations have been around for bringing multiple disparate data sets together, trying to understand the correlations between them and then coming up with some sort of insight,” Bond said. “You can only get that insight when putting data together.”
Bond added that data integrations are most frequently used for master data which is data about the people, places, and things that your organization cares about.
“You’ve gotten this data distributed over the place and so bringing that together is a data integration problem, reconciling all the different versions of that data is a master data management data integration problem, and now you have to find the most important, not necessarily the most recent version of the truth, but the best version of the truth for that particular entity in that organization and the context within which that data is being used,” Bond said.
Other common data challenges that organizations face come down to the way the data itself is stored. For example, data from legacy systems often has missing markers such as times and dates for activities and data that’s taken in from outside sources might not have the same level of detail in the data as the ones from internal sources.
“The more you know about your data, the higher quality data you have, and the better you can integrate that data,” Bond added.
Also, different parts of the organization need all that context whether that’s data governance, data quality management, analytics, data science, AI, or machine learning. “Data without intelligence, it’s just data,” Bond said.
This has all exacerbated the need for data integration tools that can simplify each step of the process to save time.
Organizations are looking towards solutions that have a lot of pre-built connectors and ones that they can port to hybrid cloud models without needing to rebuild the integrations. The data integration tools of today need to be able to work natively in a single cloud, multi-cloud, or hybrid cloud environment.
“As organizations are shifting to hybrid cloud and full cloud, there’s a number of different systems that need to connect on premise and across the different applications and services that they’re using. So as that grows, the integration challenges get more and more difficult,” said Eric Madariaga, the chief marketing officer at CData Software.
The pandemic has accelerated digital transformation, and with it, data integration initiatives
“The pandemic has accelerated digital transformation, and I’ve long stated that data is the lifeblood of digital transformation,” IDC’s Bond said. “It’s all virtual and data is so much more part of what we’re doing these days than it’s ever been before.”
When the pandemic first hit, there was some negative impact on the big data and analytics budgets and data integration is a part of that.
However, as the economic situation changed a little bit over time, the budgets for spending on big data and analytics started to increase and budgets have continued to increase as the economy starts to return to growth, Bond explained.
“When you look at what has happened during the pandemic across many industries, everyone is adapting to this new way of reaching customers,” Red Hat’s Parulkar said.
For example, governments are trying to help their citizens so that they don’t have to physically go somewhere to access their services, retail stores are trying to find new ways for their customers to get their items shipped, ordered online, or done by curbside pickup, as well as the growth of many different delivery services like DoorDash and GrubHub. Data plays an important role in making all of these processes more efficient, Parulkar explained.
The demand for data integrations already existed before the pandemic, but when it hit, the priorities changed, Arawan Gajajiva a principal solution architect at Matillion explained.
“Companies are generating exponential amounts of data every year and they’re recognizing they need to corral, wrangle, and get value out of their data, so that hasn’t changed with COVID. What I have seen, though, is that as COVID hit us last year, priorities have changed,” Gajajiva said. “It’s not that they didn’t have a data focus, it’s just what data they were focused on. And so a great example is that we’re seeing that customers were really prioritizing getting that COVID data loaded into their data warehouses.”
When it comes to types of data, IDC saw a significant increase in the demand for spatial data within the last nine months. This type of data can be used to map out where the virus is and what the numbers are in a particular location which can then be utilized for contact tracing measures.
The size but also type of organization matters when it comes to data integration initiatives
Organizations have had to restructure their infrastructure to handle the influx of data and companies of different sizes are approaching this in different ways.
Large companies have teams that are dedicated to managing data and managing the whole process around getting the data where it needs to be, and also getting the people the tools that they need to be able to process the data, whether that’s analytic tools, alerts and notifications, Mike Albritton, the managing director of ArcESB explained.
“On the other hand, small and medium size businesses, the SMB market, may or may not have teams to own that process and so they’re often looking for something that’s more out of the box, or maybe even looking for a service provider to come and help set up some sort of process for them,” Albritton said.
Red Hat’s Parulkar added that as the size of a company increases, the data integration and the data analysis and data quality becomes much more important.
On the other hand, IDC’s Bond said it has much more to do with how much data you need to function as an organization rather than it has to do with your size.
“With a startup that was born in the cloud and maybe their business is focused on data, they’ve got much greater data integration needs because data is such a core part of what they do as a business. Another company that isn’t based on that data, and they get data as a byproduct of what they do and they use data out of that to get better at what they do, their data integration needs might not be as significant as that startup that was born with data.”
Data integration tool providers are adding new functionalities such as AI and machine learning to predict what’s happening and also to build automations that will handle integrations. AI and ML are being infused into these data management and data integration products and using intelligence about the data and sometimes intelligence from the data to automate some of those activities.
“Data is essentially the fuel for AI. If you don’t have that data, how are you going to do your AI models?,” Red Hat’s Parulkar said.
Also, things like data streaming, data connectivity, and real-time data sharing are increasingly becoming more important as customers adopt microservices, DevOps practices, and more event-driven architectures, Parulkar added.
APIs are also becoming more prevalent to integrate between businesses as opposed to the traditional EDI process. APIs are much more agile, much quicker to market, and a lot easier for customers to connect in the data integration space, according to Albritton.
Another trend is bringing analytics capabilities to the cloud which opens up a lot more resources.
“We’re seeing people moving into the cloud because of cost and scalability, but now once you’ve put your analytics workload in the cloud, you’ve got a lot of different cloud services such as AI and ML that are now available for you when you’re ready,” Gajajiva said.