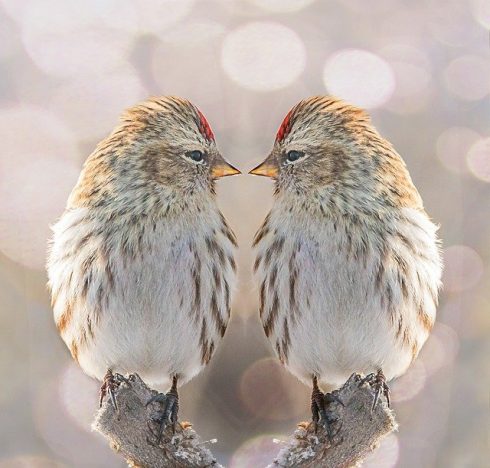
Synthetic data and digital twins are wildly different things but at the same time complementary in the sense that one simulates data for AI and other simulates the interactions of models for people and AI.
Rather than being generated by real-world events or processes synthetic data is information that’s artificially manufactured. It is data created algorithmically, and used as a substitute for test datasets of production or operational data, to validate mathematical models and recently and more frequently, to train machine learning models.
To generate synthetic data, you use an original dataset and learn the joint probability distribution using a generative model from which you sample new data.
Digital twin software is an advanced form of simulation software, using sensor data from embedded machines to create accurate, real-time simulations of assets. These virtual models allow for intelligent structural assessments and analyses, with actionable insights into machine performance and maintenance needs.
Digital twins enable what-if-scenario analysis by providing an interface which replicates and responds to human and environmental input virtually as if the physical twin was being acted upon with the physical items the twin represents. A digital twin allows for multiple experiments and scenarios to be performed and optimization of defined parameters and outputs achieved without taking the equipment or process the digital twin represents offline.
Synthetic data uses its’ algorithm to simulate the creation of data to and from a physical entity. Synthetic data can provide data volume and data variability before physical entities and their related components ceven exist e.g., during the design phase of facilities, equipment, or products. Synthetic data could then be used to create the digital twin and influence the revision to design by using optimization modeling for both minimization of risk and improvement of the performance of the product or service.
Both digital twins and synthetic data use algorithms to simulate a physical entity. Both have data that can be compared to the input\output of that physical entity. A digital twin uses data from the physical entity to create the algorithm which then “models” the physical entity. Once the digital twin AI algorithm is created it can then be used to generate synthetic data. Therefore, they can work in unison in an AI workflow cycle. Synthetic data can “prime the pump” to create the initial digital twin. Then data capture from the physical twin allows the digital twin to improve over time. Then the digital twin can be used to enhance the quality of Synthetic data in a cycle of continuous improvement.
Since both Synthetic data and Digital twins are tools with embedded AI there exists technical infrastructure that benefit both. Data storage is one aspect of common shared infrastructure which can be leveraged and if harmonized can improve the AI workflows used to create and improve Synthetic data and Digital twins. Processing capacity is a second component that can be shared and leveraged e.g., big data repository and cloud-based services that are easily scalable and shareable. AI Ops tools which allow you to version, compare and deploy models help in the generation of synthetic data work hand in hand to improve each other.
Just as any physical item can have a digital twin. Any data source and outcome can have synthetic data which emulates it. Synthetic data which represents customers, use cases, design options, and manufacturing equipment, maintenance, and capacities along with incident and accidents all can have a synthetic data set that can be used for optimization, risk analysis and decision making. Combining these synthetics data sets with AI models into digital twins which represent equipment, processes, policies and markets creates a continuously improving virtual ecosystem of synthetic data and digital twins that can be used to test, optimize and decide on the best choices, mitigate risk and optimize opportunities.