Feedback is routinely requested and occasionally considered. Using feedback and doing something with it is nowhere near as routine, unfortunately. Perhaps this has been due to a lack of a practical application based on a focused understanding of feedback loops, and how to leverage them. We’ll look at Feedback Loops, the purposeful design of a system or process to effectively gather and enable data-driven decisions; and behavior based on the feedback collected. We’ll also look at some potential issues and explore various countermeasures to address things like delayed feedback, noisy feedback, cascading feedback, and weak feedback. To do this, in this four-part series we’ll follow newly onboarded associate Alice through her experience with this new organization which needs to accelerate organizational value creation and delivery processes.
As Alice looked at the bigger picture of the quality process, it became clear that earlier feedback impacted, and may have created or obscured, subsequent feedback or issues.
A significant challenge of the past has been the ability to realistically represent and measure performance in all but the simplest of processes. The reality is that most of our processes have dependencies and external influences. While these were difficult at best using manual tools, automation of processes and the advent of observability enables a more realistic representation. Exposing obscure relationships through discovery and understanding the relationships enable a better and more robust model for identification and measurement. This is especially important to begin to see and understand relationships, especially those that are complex and not easily observed.
Alice realized that the feedback loops that were providing information to product management were frequently misunderstood or used data that was not appropriate for the use (e.g. not fully burdened costs) as conflicting and not well documented microservice architecture and API implementations that have proliferated in their current environment. Of course, we’ve long struggled with aggregating multiple KPIs that do not really reflect on or result in the desired outcome.
As Alice explained to the product manager, the interactions between complex components of a microservices environment and automated business process ecosystems are an increasingly complex environment of interactions. The delivered value or outcome must be engaged, such as the introduction of market leading capabilities faster and better than anyone else.
We can think of interdependent processes as something like the availability impact of multiple dependent systems, using availability as an analog for confidence in the feedback results as well as likely performance expectations. Additionally, this approach identifies relative capability improvement with current approach / architecture:
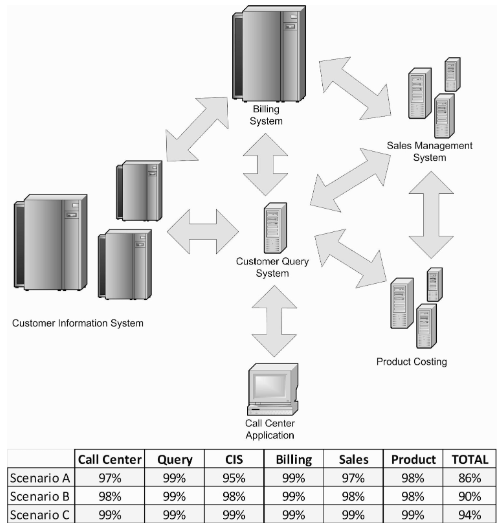
(image from Standing On Shoulders: A Leader’s Guide to Digital Transformation ©2019-2020 Standing On Shoulders, LLC, used with permission) Image & Table depicts aggregated availability based on interdependent system availability and resulting net total availability.
In this example, the total system availability is the product of the dependent systems for the same business process scenarios, in this case by looking at component improvements and availability outcomes. The impact of the performance of otherwise independent systems can have an enormous impact on complex business processes. We must take care to understand the feedback loops and how we may encourage or even create subsequent noise via cascade. Transparency can be the key.
Earlier, we talked about noise in testing and impacts to trust and confidence. That is another dimension of this same challenge, and opportunity.
Alice and the product manager concluded that this might be related to their objectives for reduced fire fighting and improved collaboration. Improved monitoring and if possible adding instrumentation or telemetry might be effective countermeasures that are consistent with other ongoing work. The direct visibility of impact and alignment with the outcome is the best feedback of all, particularly when our part may be somewhat obscured or limited by other stream components. Understanding and modeling enable us to experiment and learn, especially with critical value systems.
Looking ahead, improving ecosystem visualization capabilities in an evolving value stream management environment to capture and evaluate model quality and data consistency seems imminent. Doing this might be a goal state that should soon be realizable soon with dynamic traceability maturing and observability seemingly in our near future.