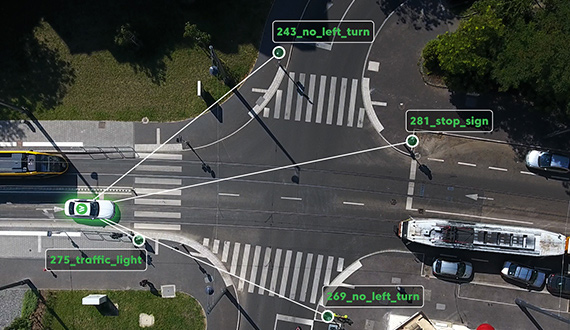
The race is officially on to market seamless autonomous driving technology within the shortest possible timeframe. One of the biggest challenges, however, lies in preparing autonomous vehicles to navigate the many unforeseen and unexpected obstacles that face drivers on any given journey.
Real-world testing, while ideal, of course, falls short of producing enough quality, representative, diverse and well-labeled data to properly train the AI components of self-driving vehicles. The very nature of the real world makes it impossible to predict, and the driving experience is fraught with various unforeseen obstacles, unexpected traffic delays and detours, and sudden changes in weather conditions. To properly prepare autonomous vehicles to properly navigate and react, it is imperative the industry looks beyond real-world testing.
Simulation Superiority
A game engine-based, virtual reality environment could offer an attractive alternative as it is cheaper, faster and safer than experimenting with real vehicles. Also, scenes can be repeated an endless number of times that would either hardly ever happen in real life, or would pose an intolerable risk to human drivers.
Take the very basic example of someone lying on the roadway. You certainly can’t use a real person to mimic this scenario, and a dummy would cause untold traffic problems. The same applies, for example, to having hitchhikers in the emergency lane, or unexpected roadwork or an impaired driver, or a sudden traffic accident.
Applying a digital setting for autonomous vehicle (AV) testing brings the ultimate benefit of engaging AI for scenarios that cannot be replicated under real-life conditions.
Even if driving conditions were fairly standard, covering thousands of miles would take a massive fleet and a significant investment in time. Alternatively, a set of high-performance computers gets the job done within an hour. Simulating cameras and sensors, in real time if necessary, can cut testing time tremendously and save big dollars.
Handling Traffic Madness Hotspots
That brings up another key issue, namely motion planning. According to current consensus in the industry, machine recognition software can be trained by feeding AI images or footage captured in traffic. The same doesn’t apply to motion planning, however, since the very nature of a moving car influences variables in your surroundings. This is why simulation-based AI is the only way of training around motion.
Look at the Arc de Triomphe in Paris, one of the craziest roundabouts in Europe. Traffic from 12 major avenues feed into it, and drivers navigate in six lanes without road markings. The French even have two types of insurance policies: those that exclude and those that include using your car there. Drivers inch rather instinctively in that roundabout, and it would be pretty hard to imagine risking accidents just to train AI on the spot. By contrast, a simulated algorithm can prepare AI to fine-tune motion planning specifically to navigate the Arc de Triomphe roundabout or any number of hotspots all around the world.
Walking on the path of game engines
The most recent survey of the National Highway Traffic Safety Administration lists critical reasons for vehicle crashes in the United States. The good news is, many of the non-driver-related factors that cause accidents can be effectively simulated, including technical errors.
Game engine-based testing comes in handy, for instance, when you need to see what happens if a sensor or a car part breaks down. But grave danger may often be an issue here too – you can imagine how hard it would be to find candidates willing to sign up to navigate a tire blowout while driving 100 miles per hour.
Our in-house developed simulator at AImotive can already handle one hundred traffic scenarios under different weather, lighting and road surface conditions. We started out with the idea that we could use video games to create the first neural networks for our development purposes, relying on images from Project Cars. However, it wasn’t able to be as flexible as we needed, and it didn’t feature conventional driver obstacles such as buses or pedestrians. Consequently, a customized version of Unreal’s game engine was created, which provided us with the variability needed for full-scale simulation. The merging of AI-trained self-driving tech with gaming technology has been just the right bridge needed to make testing much more accurate, far less time consuming and at an ideal cost.
Real-World Testing Won’t Be Gone for Good
It is worth noting, however, that a gaming environment is not the cure-all solution. Real-world testing, despite its shortcomings, still plays a critical role in autonomous development as simulation often lacks the kind of variability that can be found only in real life.
For the self-driving industry, however, it is perfectly fine if around 90-95% of testing takes place in a simulated environment. Observing that ratio is crucial to reaching full autonomy in a timely manner, and smart developers are discovering that simulation provides the smartest, safest and fastest way to put self-driving vehicles on the road.