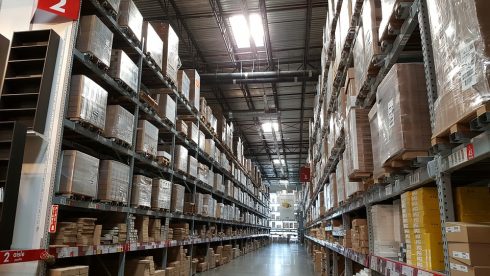
Data warehousing is one of the core sources of enterprise information, but most organizations are still unable to unlock the potential value of their investments. For one thing, traditional data warehouses require significant domain experience and manually-configured rules to enable the extraction of useful data. Modern data warehouses add machine learning, AI and deep learning capabilities to surface insights that exceed the capabilities traditional data warehouses.
“Machine learning and deep learning add a different dimension to data warehouses, said Flavio Villanustre, CISO and VP, Technology at LexisNexis Risk Solutions. “For example, you may be able to pinpoint anomalies without even looking for them such as why a loyal customer’s behavior has changed.”
RELATED CONTENT: Is DataOps the next big thing?
Data scientists know this. However, most software developers, business analysts and IT professionals haven’t learned the capabilities and limitations of machine learning, AI and deep learning yet because their positions didn’t require such knowledge in the past. Given the rapidly growing popularity of machine intelligence and its associated use cases, just about everyone must acquire new knowledge and skills so they can drive new forms of value. It’s also helpful to take advantage of tools that allow organizations to mature at their own pace as they transition from traditional data warehouses to their modern, intelligent counterparts.
Work Smarter, Not Harder
Traditional data warehouse administrators work with the business to define a set of rules that transform vast amounts of data into reports. As organizations add machine learning and deep learning capabilities, they may continue to update the old hard-coded business rules and they may even encode new ones, but the insights they get are no longer limited to preprogrammed rules. Instead, businesses get the dual benefit of traditional reporting and advanced analytics.
“If you’re at the earliest stage of maturity, you’re used to asking questions of an SQL or NoSQL database or data lake in the form of reports,” said Villanustre. “In a modern data warehouse that has a deep learning capability with anomaly detection, you also get new insights that could have a profound effect on your company and customers such as a security breach, other crimes in progress, the early warning signs of a disease outbreak or fraud.”
Unlike pre-programmed rules that identify the “known knowns” in data, deep learning can identify the “unknown unknowns” which come in the form of risks and opportunities. To help democratize the use of machine learning and deep learning, HPCC Systems provides a consistent data-centric programming language, two processing platforms and an end-to-end architecture for effective processing. With it, developers can design Big Data-powered applications that improve the quality of business decisions and accelerate time to results.
In the absence of HPCC Systems, organizations can add basic machine learning capabilities on top of their data warehouse, which requires specific domain knowledge, time and investment. To take advantage of that, they also need labeled data for training purposes. With the right expertise, data governance and maintenance, the system may yield the desired results. If it does, the organization will add deep learning capabilities next, which require yet more specific knowledge, skills and investments.
By comparison, each HPCC Systems instance includes a traditional data warehouse system, including a data lake and strong integration with deep learning frameworks including TensorFlow so organizations can mature at their own pace without unnecessary expenses and friction.
“If you use HPCC Systems, you can leverage its strong data management capability to build your data warehouse, data lake and all the analytics you need,” said Villanustre. “You can also leverage TensorFlow to build deep learning models on top of your data which is something that’s hard to get from the other platforms.”
HPCC Systems also does not require data duplication, which further lowers costs and risks.
“If you’ve got two copies of data, keeping them synchronized is a big challenge,” said Villanustre. “If the data changes and the import didn’t work as required, you have to reimport the data in both locations. That’s unnecessarily expensive and time-consuming.”
Get Open Source Flexibility
HPCC Systems is an open source platform so there are no software licensing fees. It integrates with the Google TensorFlow and all TensorFlow-compatible components including the Keras API for building and training deep learning models.
“With HPCC Systems, you have the flexibility to extend the system as much as you want using proprietary or open source components,” said Villanustre. “Our decision tools combine public and industry-specific content with advanced technology and analytics so customers can evaluate and predict risk and enhance operational efficiency.”
Learn more at www.hpccsystems.com.
Content provided by SD Times and LexisNexis.