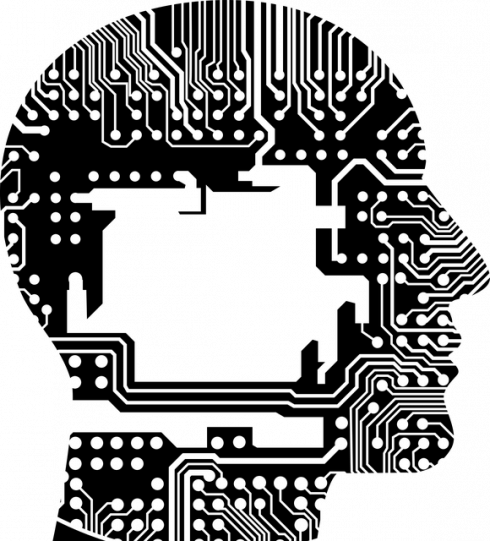
Last year, we stated 2016 was the year of artificial intelligence as tools and solutions became smarter and more advanced. This past year, artificial intelligence went from a sought-after technology to a reality for most organizations.
Today, we have devices that can react, respond and recommend on their own. Developer solutions have also evolved to automate and speed up the software development lifecycle through automated testing and automated builds as well as the ability find, detect and fix bugs through monitoring and testing. With the Internet of Things, and the multiple devices people have today, it would not be possible to manage the amount of data and learn from that data to make intelligent business decisions without artificial intelligence.
A few years ago, those capabilities were only an idea for the future or only available to a few technology giants, and now it is becoming the new norm for devices and solutions. This is only the beginning as organizations invest heavily in the technology and clear the path for developers. It is important to note that when we talk about artificial intelligence, it is used as an umbrella term and encompasses AI, machine learning, deep learning, and other cognitive technologies.
Google continued its work in the machine learning realm with its open-source framework for machine learning, TensorFlow. In February, the library finally reached 1.0. Google built upon the library throughout the year with the release of TensorFlow Debugger for visibility into TensorFlow graphs, Tensor2Tensor for training deep learning models, the TensorFlow Object Detection API, and TensorFlow Lite for machine learning models on mobile and embedded devices. By the end of the year, TensorFlow reached version 1.4 with the machine learning framework Keras, the dataset API, support for Python generators, and new functions for simplifying training and evaluation.
Google also started the AI through the People + AI Research initiative announced in July. The initiative is designed to look into how users interact with AI and the benefits it will provide.
Other companies worked on providing developers with more tools to build on AI. NVIDIA announced a mission to close the expertise gap in the field of artificial intelligence in May at the GPU Technology Conference. The company announced plans to increase the amount of AI developers tenfold this year through the NVIDIA Deep Learning Institute. NVIDIA also teamed up with Facebook earlier in the year to release Caffe2, an open-source deep learning framework for creating large-scale distributed training scenarios. Salesforce announced Einstein Platform Services to give developers more tools to build AI into their apps.
Microsoft continued to invest in artificial intelligence technology throughout the year with the launch of the Open Neural Network Exchange in conjunction with Facebook to provide neural network framework interoperability, the release of its Security Risk Detection tool that uses AI to find and detect software bugs, the launch of the open-source deep learning library Gluon with Amazon, Visual Studio Tools for AI, and Azure IoT Edge.
Other investments included: IBM announced plans to create an AI research partnership with MIT, and the Partnership on AI to Benefit People and Society expanded its efforts with eight new for-profit partners and 14 non-profit partners. New venture capital funds such as Toyota AI Ventures, and Google’s Gradient Ventures were created to provide funding, mentorship and support to AI startups.
The Linux Foundation also introduced the Acumous Project to help democratize the building, sharing and deploying of AI apps.
However, despite the advantages to artificial intelligence, others warned that users should not get too comfortable. Carnegie Mellon University’s Software Engineering Institute released its 2017 Emerging Technology Domains Risk report in October warning about the security impact of vulnerabilities in machine learning, especially when sensitive information is involved. “The ability of an adversary to introduce malicious or specially crafted data for use by a machine learning algorithm may lead to inaccurate conclusions or incorrect behavior,” according to the report.
Hyrum Anderson, technical director of data science for cybersecurity provider Endgame, had similar views when he spoke at the 2017 USA Black Hat event in July and stated that machine learning was not a silver bullet for security. According to Anderson, machine learning has blind spots and can be easy to exploit.
DeepMind launched the DeepMind Ethics & Society research unit in October to not only help technologies develop AI application, but to pave the way for beneficial and responsible AI.
Looking towards 2018, Gartner revealed having an AI foundation will be a top strategy technology trend with areas like data preparation, integration, algorithm and training methodology selection, and model creation being ripe for investment. Gartner also sees new intelligent apps, analytics, and things such as autonomous vehicles, robots, drones and IoT being prevalent in the AI landscape next year.
In Forrester’s 2018 predictions, the organization foresees artificial intelligence and machine learning continuing to replace manual and repetitive tasks through bots in the coming year.