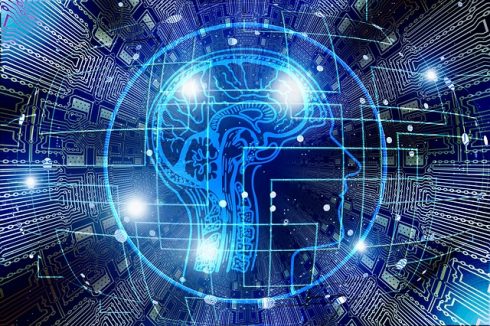
Practical adoption of artificial intelligence (AI) is driven by an exclusive club of companies. A report from McKinsey Global Institute, published in June 2017, ascertained that investment in AI is dominated by digital giants such as Google and Baidu. On the other hand, the vast majority of firms have not taken any practical steps at all yet. According to Gartner’s 2018 CIO Agenda Survey, four percent of CIOs globally have implemented AI, while a further 46 percent have developed plans to do so.
This investment asymmetry is creating a dangerous gap between a few firms that are increasing their competitive advantage and their already dominant market position with help of AI – and the many firms that are left behind.
Huge opportunities – low adoption
The potential of adopting AI in the enterprise is huge. PricewaterhouseCoopers predicts the global gross domestic product to grow 14 percent – the equivalent of $15.7 trillion – by 2030 as a result of AI, driven by increased productivity and higher sales. Going forward, AI will be embedded everywhere, both in the products and services sold to customers and along the entire value chain, which is employed to plan, produce, market and provide those products and services – driving outcomes such as better demand forecasting, improved operational efficiency and increased sales.
With such huge opportunities, why is the adoption rate so low? Many firms struggle to even get started because they cannot find meaningful and profitable AI use cases. Autonomous vehicles, humanoid robots and voice assistants are in media headlines every day, but it’s hard to define the starting point for how to deploy AI in everyday business.
And when this starting point is found, technology is the next challenge. Data and infrastructure environments for AI must be configured to meet the specific requirements of a particular use case. Firms often don’t have the expertise to build such tailored environments.
“AI democratization” creates value, but not a competitive advantage
Some technology companies claim they want to close the gap and “democratize” AI by providing off-the-shelf solutions – pre-trained AI models, ready to use in the cloud and equipped with algorithms that are constantly optimized.
These solutions relieve firms from the burden of setting up an AI data and technology environment from scratch. They can also provide superior value as they are fed with data from thousands or millions of similar deployments in other firms. Think of an AI solution that monitors your corporate IT environment to detect looming outages and recommends actions to prevent them. The more data sources this solution is based on, the better the detection and recommendations will be. Such off-the-shelf AI solutions are still rare, but the number is quickly increasing.
However, while this approach can create superior value, it does not suffice when the goal is to create a competitive advantage. By definition, off-the-shelf AI is suitable for a firm’s use cases that are identical or very similar to other firms’ use cases. Creating a competitive advantage, on the other hand, means creating value that is different or superior to other firms.
The analytics-AI continuum
Unfortunately, there are no shortcuts when it comes to implementing AI in the enterprise to create a competitive advantage. Firms must define their AI strategy, identify promising use cases, source the data, buy and build technologies, and put the right people and processes in place.
Moreover, AI does not flourish in isolation, but is the ultimate step in a continuum, where everyday business analytics graduate to big data to autonomous systems based on AI. That’s a key reason why tech giants extend their edge so quickly – they already have the data and analytics foundation in place to which they can add AI – while laggards have to build this foundation first.
A key success factor is the underlying data, because algorithms can only be as good as the data with which AI models are fed – crap in, crap out. Ultimately, AI requires a unified data architecture and governance, providing standardized, labeled, clean data and centralized data lakes that feed all AI projects. This will prevent firms from creating AI islands. And it will ensure that AI models can discover patterns and relationships in data that might seem unrelated in the first place.
This does not mean firms have to wait with implementing AI until they have established an enterprise-wide data and analytics foundation. Long-term plans and short-term projects will be tightly interwoven, with the plan guiding the projects and the projects generating learnings that enrich the plan. Here is a three-phased approach to consider.
1. Find the use case
It might seem simple to find AI use cases, some of which are well-documented. In its study of the AI landscape, McKinsey Global Institute reviewed 160 AI use cases – only 12 percent of which had reached commercial adoption. Where is the problem? The use case can be too big or too complicated. It can be hard to prove short-term value, which is required to convince stakeholders. Or data is not available in the required variety, quality or quantity.
More often than not, the best initial use case for AI won’t be the company’s biggest problem. Making AI real means going beyond the hype, focusing on what is doable in a defined timeframe, with the budget, resources and data that are available. By doing this, firms often discover a more specific use case than they initially considered. For example, instead of trying to improve prediction of customer demand overall, they start with sentiment analysis on social media to establish a better customer dialogue process. It doesn’t matter how big the initial use case is. The key is to get started, show tangible success, enter the learning curve and extend AI usage across the enterprise.
2. Create the data and technology environment
Companies need to be very specific about what they’re trying to achieve to determine the technical resources required to get there – from identifying the data sources to selecting the data science tools, AI frameworks and models to the CPU/GPU ratio for processing and storage and backup needs. Think of two use cases employing AI-enabled video analytics as an example – identify license plates to determine taxes in a parking garage and facial recognition to find criminals at an airport. They vary significantly in terms of complexity, real-time requirements and retention policies and thus require different hardware/software stacks. This means firms have to precisely characterize and benchmark their specific AI workloads and process requirements to determine the optimal technology and its configuration, as well as the required data sets and data quality. This requires deep and specific technology expertise, as well as benchmark and configuration tools which can significantly simplify the task.
3. Stick to your plan and scale across the enterprise
While a firm goes through the exploration phase, it’s crucial to keep the long-term plan in mind which defines the role of AI in the company’s strategy, including opportunities, obstacles and critical success factors. Scaling AI usage throughout the value chain will require to systematically close gaps in a firm’s capabilities. This can be infrastructure modernization, skills, management of change, data governance and security.
One of the most important gaps to close will be to create a company culture in which humans interact with machines in fundamentally new ways. In a traditional world, data analysts are giving recommendations to decision makers. With AI, firms are automating business processes based on decisions taken by an algorithm – and this will always involve uncertainties and probabilities along the way. This means creating a competitive advantage with AI requires both trust and vigilance; trust in the power of technology and vigilance to remain the master of one’s own AI journey.