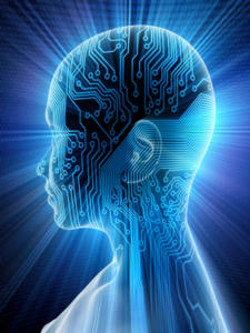
How can programmers benefit from the “the year of Neural Nets”? Statistical machine learning techniques have been surging in popularity in academic settings for years, but 2015 was a watershed in terms of industry awareness and deployment. It was not long ago when the term “Deep Neural Networks” seemed about as dubious an explanation as “Applied Phlebotinum,” but now, Google’s open-source release of TensorFlow was the source of celebration and rewriting of business plans. (Although the consensus seems to be that Theano may hold multiple advantages.)
It’s important to understand that neural networks are not on the threshold of replacing traditional programming techniques, much less general intelligence.
Neural networks do not do any symbolic processing and are neither “logical” nor “creative.” Neural networks are “universal approximators,” meaning that they can generate arbitrarily accurate results for a given function. The inputs are swept forward through massive numbers of amplifier weights and transfer functions, and the output is the (surprisingly effective) mapping of this gestalt into meaningful results. The “intelligence” in artificial neural networks stems from the manner in which the amplifier weights are trained and the specific topology used to map inputs to outputs (for instance, the use of recurring sliding windows over small sections of an image).
(Related: How IBM is using Watson beyond winning at trivia)
While advances in recent years in how the networks are configured and trained have led to fantastic results, there are limits to what can be done if (at runtime) the process is strictly feed-forward. We are all walking proof that sophisticated symbolic processing can arise on a substrate of deeply interconnected neurons whose behavior has rough analogies to the weights-and-transfer functions of artificial neural nets, but it is certain that complicated feedback modes are required for anything remotely in the realm of “higher-level thinking.” Understanding such modes is almost entirely speculative and far beyond the state of the art in artificial neural networks.
All of which is to say that artificial neural networks and other statistical machine-learning technologies are not going to start competing for software development jobs soon. Advances in symbolic processing seem to be languishing, except, perhaps, for IBM’s Watson project, which seems to be establishing a coherent niche for integrating large-input corpuses to produce correct answers.
Data does not influence the way we develop software. There is virtually no development “best practice” based on statistically meaningful data (the exception being “shorter user-feedback cycles are better,” which I feel has been amply supported). And yet, two of the most popular websites in the world are potential treasure troves: Stack Overflow (57th in world, according to Alexa.com) and GitHub (85th). And it’s hard to imagine a task better suited to monitoring for analytics than the computer-based task of writing code (always bearing in mind that this is just a subset of the job of software development).
It’s easy to write scripts that process Stack Overflow data by tag or text. While this can be immediately helpful in identifying popularity trends, this is barely scratching the surface, given that Stack Overflow is literally a database of cognitive stumbling blocks. Every question on Stack Overflow is a testament to something not being clear (to return to the topic of my last column).
A good example of the way that data should influence the way we develop software is Microsoft’s “Windows Error Reporting” tool. Talk about Big Data: Microsoft receives more than 100 million crash reports per day. A fascinating article from 2009 by Kirk Glerum and others at Microsoft discusses how these reports are used. Not surprisingly, the major benefit appears to be prioritizing defects. More to the point, the article discusses the attempt to properly map a crash report to a single defect “bucket.” When the article was written, this was done using hand-written functions comprising “100,000 lines of code implementing some 500 bucketing heuristics.” This is testimony to the difficulty of the task, but I would be disappointed if, by now, Microsoft Research had not applied machine learning to the problem. The article also discusses the use of massive data to find root causes, although the implication is that this is (or was) based on manual searches.
In addition to analytics about the crashes that occur at runtime, what about the errors that are seen at compile time? I was recently talking with a colleague about the potential (and privacy objections) for compiler and IDE vendors to analyze compiler errors. It’s probably safe to assume that missing semicolons and miscounted closing braces are going to lead the field, but wouldn’t it be interesting to know which library functions are most frequently called with the wrong parameter types or are correlated with defect-prone functions?
Although I argued previously that developers do not have to worry about software taking their jobs, I think it’s very possible that developers could benefit greatly from machine-written code that is proposed as a possible solution. At the Splash 2011 conference, Markus Püschel gave a fascinating keynote on “automatic performance programming” in which he described the use of generative techniques to create fine-tuned implementations of the fast Fourier transform. The paper is unfortunately behind a paywall, but you can find some information at the Spiral project homepage.
Finally, there’s something fundamentally wasteful about writing unit tests and then writing code to satisfy the constraints you’ve just specified. I like to imagine that a day will come when, for certain programming tasks, I can ask my computer to present me with some suggestions. A developer asking for a proposed function that satisfies defined constraints is a vastly simpler scenario than a generalized software-writing scenario, and although it’s certainly in the realm of a research-level problem, I think it’s something we might see within several years.
None of the scenarios I’ve proposed are possible without considerable pre-processing of the data into a usable form, as well as some amount of symbolic processing to create the patterns for neural nets to work upon. But software developers have for too long been “the cobbler’s children who go shoeless” and deserve to reap the benefits of this technology. The data sets are there, the deep-learning libraries are open source; all that is required is some insightful developers to put it all together.