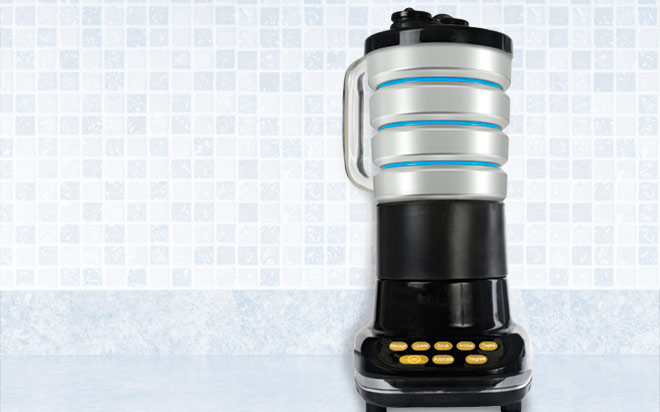
Making the case for version control, testing environments and continuous integration when it comes to software development these days is a no brainer. But when it comes to the data behind your important applications, life-cycle management and data flow automation are still new ideas struggling to find their place in the market.
That doesn’t mean, however, that managing your data is an impossible task, devoid of vendors and best practices. But it does mean that most enterprises may not yet fully comprehend what exactly data automation entails.
It can refer to a number of different things, or to all of them together in a single workflow. These include things like data scrubbing to remove sensitive data before use in testing environments. It can also refer to the flow of data from production systems, back to data warehouses, then forward again into analytics data stores. Data automation can even refer to the actual change management of data as it comes into the system and evolves over time.
With such a broad space to cover, the term data automation has a lot of heavy lifting to do when it comes to being advocated for inside your organization. But it doesn’t have to be an impossible struggle, thanks to numerous companies and tools that make managing that data life cycle much easier.
Seb Taveau, senior business leader and technical evangelist at MasterCard for its Open APIs, said that the management of data in the software development life cycle is extremely important for a heavily regulated organization like his.
(Related: Scaling agile in databases)
MasterCard has a particularly interesting set of problems when it comes to the data life cycle. As a credit card company, financial regulations weigh heavily on what types of data MasterCard can collect, let alone share. And yet, the company still has been able to build up a developer network of APIs and services based on their data. This data includes information like purchases made at specific locations, as well as the time stamps associated with them. As a result, using MasterCard APIs, developers can determine which restaurants in their town are the most popular among locals, or when certain businesses are open.
“MasterCard’s been working on taking some of its private APIs and making them public for the past four years,” said Taveau. “The developer program is not new inside MasterCard. This became a key project for MasterCard about a year ago. They wanted to make sure MasterCard was a tech companion, not just a payment network.”
But having all that data publicly available means there’s a lot of work to be done before an API can go live. Depending on the data source, the project to open the data behind an API can sometimes be very easy, and sometimes require a lot of hands-on work.
“Sometimes it works great; sometimes it requires manual work to make sure it works,” said Taveau. “It depends on the complexity of the API and the data you’re trying to reach. Location is very easy to automate: It doesn’t require the security. The data is not critical—just a pinpoint to a merchant location or restaurant location or ATM location. That’s not critical.”
When it comes to more delicate information, said Taveau, the effort requires more coordination and care. “When you talk about a merchant identifier API, that’s a completely different story. As you expect, the review process for the location API and the tokenization API or risk management are very different.”
Shifting tools
Yaniv Yehuda, cofounder and CTO of DBmaestro, said that managing software is almost second nature to developers, but managing the data is another story entirely. “Tools are important, as well as human processes. But what we found out is that people are really challenged when they get to the database. Databases don’t follow the same processes the code does. They require special attention,” he said.
The reason for the difference in managing them is that they are created in different ways and have a different workflow, said Yehuda. “When you deal with traditional code, you have your development people working with version control. Then it gets built by a build server and pushed to the next environment,” he said.
“When you deal with a database, a database is not compiled in one area and then pushed to another. The database actually holds its internal structure of the data: the schema in each environment. If you have a development environment, it holds the structure code content as the application, but it still hosts the same data in the Q/A environment. In order to deal with that, you create transition code that changes the database from one state to the next.
“The database, in order to be promoted from one version to the next, it requires additional steps in order to deal with that transition code. Because people are really doing stuff with traditional tools, developers have to do this stuff manually. This code is really static, so if you have some changes in your development environment and you want them to push to another environment, then you write the code to deal with that transition. You have code override, and people are not using the database itself to manage version control because they have to extract the objects from the database and put them in a different version control from the code.
“What DBmaestro does first is to create a bulletproof version-control system. We created an enforced version control to the database. You cannot change the database unless you check out the object. You go to the database and say ‘add column,’ but only when you check it out. When you check it back in, it gets version-controlled. The second thing DBmaestro does is it safely automates deployments from one environment to another.”
Red Gate offers similar tools for data automation and management. Ben Rees, general manager at Red Gate, said that the company has been building tools around this problem for 15 years now. “People were using them to do database life-cycle management, but we didn’t call it that back then. Back then we called it agile database delivery and agile database development,” he said.
“The essence of it is that there are developers and DBAs who want to make changes to their databases, and more importantly, they want to make those changes live. They’ve been doing that using our tools, and have been following this process. We’ve only realized recently what they’re doing is application life-cycle management, except for the database.”
(Related: A better way to look at databases)
Rees said that Red Gate’s tools can help a development organization get control of data management within its application life cycle. “It’s about source controlling the database. It’s about automating the updates. It’s about properly managing the release process, and having proper release management to get changes into production. Then it’s about monitoring what happens after the event.”
Thus, as developers have awakened to database management, Red Gate has awakened to how its customers are using its tools. “What we’re realizing now, over the last year or so, is that we have this complete story that our customers have been telling us over and over for years,” said Rees. “We used to sell these 1,500-point tools to the end user. Now we’re selling something that’s about changes in process, changes in how you work.”
That means Red Gate can no longer rely on single developers using a credit card to buy a tool. Instead, data management and the database life cycle have moved up the stack to become CIO-level concerns. Getting buy-in from that far up the chain can ensure that a new process and life cycle can be pushed out across the organization, not just into small pockets.
Ron Huizenga, data architect and product manager at Embarcadero Technologies, said that change management in databases is a great way to ensure changes don’t destroy essential information.
“We have database change-management tools, from the metadata approach, from the modeling approach, and the metadata artifacts,” he said. “It’s extremely important to be able to map out all of what that is.
“There’s also a difference in terms of the data content in those stores. And that’s where we really get into an enterprise data lineage. You may have a company that has employee data scattered across a number of different systems. How do we map that together, and how do we know if info is changing in its journey through the system?”
Embarcadero, he said, has tools that can tie different data store terms together. If one database refers to employees with the field “EMP” while another uses the field title “PEOP,” Embarcadero’s tools can be used to define these two different data columns as meaning the same thing, thus allowing for quick integration of data from disparate data sources.
New stores, new processes
When it comes to the actual database, NoSQL data stores are offering another wrinkle. Couchbase, for example, is a NoSQL database that behaves similarly to Lotus Notes. For mobile applications, this means that Couchbase can be used to embed a datastore onto a mobile device, then sync that datastore with the remotely hosted version of Couchbase.
Wayne Carter, chief architect of mobile at Couchbase, said that database management has changed drastically in recent years.
“I come from Oracle, where I spent 14 years,” he said. “I grew up in CRM from Siebel, and the models in CRM are extremely complicated. We went through several variances, and even a simple thing like a contact… the model associated with that becomes monstrous over time.
“At the application logic level, some things you’d do in the database, like validation into your application code, and things like joins and queries just to get simple objects. That just doesn’t work in today’s mobile space. Applications move faster and need to evolve a lot faster. These are completely dependent on databases, from the change tracking perspective.”
He went on to say that, “For our database, because it’s moved to the application for management, it’s managed at the code level. The same code can be used for archiving [and] versioning, and could be stored on GitHub… rather than within the database. The actual migration of the database is being managed on the change management [system].” Thus, NoSQL data stores allow the application to define the layout of the data. Because of this, simply doing version control within the application code itself can help to manage the data.
Mongo extends this concept even further with its MongoDB Management System. This enterprise tool for managing MongoDB instances can quickly spin up test environments and replicate data from one area to another, ensuring testers and developers can access the data during development.
That doesn’t mean it’s the entire solution to the data management problem, yet, however. Kelly Stirman, director of products at Mongo, said, “What it doesn’t do is things like data masking and sampling. It doesn’t generate different distributions of fields and attributes or create randomized samples. There’s a lot of interesting stuff you could do to load test your applications based on the distribution of certain types of values on a known data set. We’re looking at that and thinking about [adding these capabilities to future versions].”
The business of data
Sean Poulley, vice president of databases and data warehousing at IBM, said that understanding the data life cycle requires an understanding of how a database works in a services environment. “One of the things people often misunderstand is that having a database is one thing, but running a data service is another. There’s a world of difference between having a database-management product and actually delivering it as a service,” he said.
To this end, IBM has been focusing on CouchDB as its platform for the future. IBM acquired Cloudant in February 2014, and has since been building enterprise products around CouchDB, the database originally created by ex-Lotus developer Damien Katz. CouchDB is, essentially, an attempt to recreate the database system inside of Lotus Notes.
“CouchDB has built a lot of clever management tools around how they load balance and around the database capabilities,” said Poulley. “Cloudant did a really nice job. At one stage, they looked like they were going to fork CouchDB, but they decided to contribute that back to Apache CouchDB. We’re seeing tremendous uptake; it’s one of those teams with all the ingredients for success. I actually spent a period of my career inside Lotus. Not everybody recognizes what CouchDB is. The first time I saw CouchDB and Cloudant I thought, ‘That’s Lotus!’ ”
IBM’s view of the database-management problem boils down to the enterprise network that includes data zones, said Poulley. “We talk about data zones, and we came up with these different zones based on thousands of customer engagements. What we observed is that customers had four or five data zones. One would be a relational, operational store. Then we would find, classically, the data warehouse zone, and the data-mart zone, typically with the conditional relational model. Then we’re seeing this emergence, particularly with Hadoop, of an exploration zone, where data is being brought in raw and dumped into Hadoop.”
That’s a change, said Poulley, from where data has traditionally lived and for how it’s being managed. “Before, people would do preprocessing before they moved it into a relational data warehouse,” he said.
“Similarly, we’ve seen with the explosion of data analytics, and the growth of data, we’re seeing a tremendous growth in our relational data warehouse as well. Understanding the true value is not in the physical storage itself, it’s in being able to process the info you need at the speed you need it, for the period you need it. This idea, which is not new, is becoming increasingly interesting as a logical data warehouse where data flows to and from a high-performance environment like a Netezza data warehouse, into something like Big Insights, and vice versa.
“If you move info from the relational world into the Hadoop world, you can still introduce the queries. That’s what we describe as an actionable archive: You can run the same queries on Hadoop, keep the hot data in the performant environment, and move the longer-term data into a colder environment.”
Thus, Poulley advocates the reuse of queries instead of the replication of data. Rather than moving data around from data warehouses to relational data stores and into NoSQLs or test environments, he advocates keeping the data where it is and writing queries that can run on any type of data store.
So, perhaps data management and automation isn’t just about moving around the data and masking it. Perhaps it’s more about moving as much code out of the database as possible so that it can be managed in the same way as software. Then, instead of bringing the data to the software, it’s perhaps easier and more efficient to bring the software to the data. That requires some steps in between to ensure some basic capabilities around the data, such as masking, however.
“Once you know you can mask the data, the idea of capturing the workflows is almost like a VCR form,” said Poulley. “To really capture the workloads that are happening on a day-to-day basis is something most companies don’t do, which they should. They put it in a staging environment, but it never really experiences true workflows until it experiences the workflows of live data. Being able to capture sample data and being able to mask the data is something all companies should be doing.”
Thus, Poulley advocates testing applications with real-world data traffic as much as possible. Doing so, he said, will give you a better idea of what the application will do in production. And doing all of this will allow developers to “bring the analytics to the data, rather than the other way around,” he said.
“The innovation in the next few years won’t be about data movement; it’ll be about query movement. It’s much easier to move the queries and federate the queries.”
Back to the master
No matter how you manage your data, there is one thing that is for certain: dealing with the data itself requires discussions with the controller of that data. At MasterCard, every API in the company is controlled by one group or another. That means when MasterCard’s Taveau and his team are beginning the work of taking an API public, their first stop is in the offices of the team that manages the API and data they’re working on.
“When you’re looking at an API, we won’t be exposing single data points through an API; we create an aggregate of the data, and that’s what the API will look at,” he said. “This requires a lot of discussion internally on the whys and the why nots, where we discuss the value that can be brought out of these type of packages. How do you do it? There’s this filter, then it’s reviewed, then we check the data we’re putting in the package. We have the information security team to make sure the code is made properly, and we have friends in sales to see if there’s value in it.
“At the end of the day, the value is in the data and the quality of the data.”