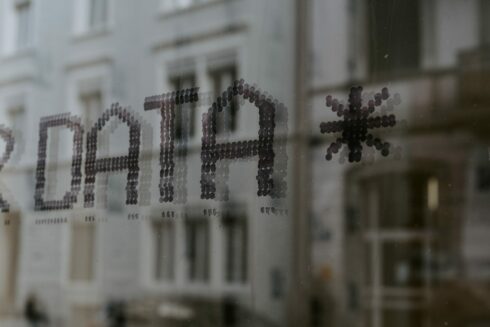
Data capture provides a better way to make informed decisions, but only when an organization truly taps into the value of that data. We capture data on and around everything, meaning information availability isn’t the issue. The challenges now are: How can we get more value from that data? How can we make sure we’re getting the information we need? How can we speed up time to insight?
Modern streaming systems send massive amounts of data consistently at lightning-fast speeds. It takes a long time for data processing systems to clean and reshape the raw data before sending it to the correct analytical tools. This process adds time between ingest and when analysis can begin, meaning “real-time” is still happening on a delay. Most data-driven organizations must engage in this lethargic process, but not all. There is a way to have true real-time analysis and predictive modeling available for those decisions that can’t wait. Purpose-built time series databases speed up time to insight and process data faster and more efficiently.
A time series database is uniquely qualified to handle streaming data and share insights with up to nanosecond precision. Time series data is any data that an organization or individual uses to monitor changes over time. General categories include sensor data, server metrics, application monitoring data, and stock pricing but also include any data indexed by time. Streaming data or events occurring over time certainly fall under this category. Time series databases have features built specifically for working with this use case.
Painting the full data picture
Preventive maintenance
A capital equipment manufacturer who offers customer support and extended warranties on their equipment began including instances of a time series solution with every machine they sold. The hardware/software solution included a dedicated hardware gateway with open source data collection software, the cloud-based time series database instance, and real-time dashboards. This allowed both the manufacturer and the customer viewing access for all the machine process data such as amperage, pressure, water levels, battery power, or pH levels.
The manufacturer who wanted to keep costs down on a possible replacement harnessed the analytical powers of the time series database. They leveraged the tiered storage and compression along with the real-time data and performed a historical trend analysis. This advanced statistical analysis alongside machine learning models helped the manufacturer estimate when the machine is in need of valuable fluid replacement. They now inform the client on when to replace these fluids rather than wait for the client to estimate when to refill the tank.
Seeing previously imperceptible issues
Time series data tracks the smallest of details and builds a model of changes over time versus the baseline. A factory’s press was experiencing downtimes just under one minute. There was a notification threshold, but those short downtimes fell under the notification threshold yet were still long enough to cause delays. The time series data revealed the remaining piece of metal after the initial machine process completed was longer just before the downtime. The scrap length difference was so small it was unnoticeable to the operators. After time series analysis discovered this difference, the cause was immediately pinpointed. Now, as soon as the metal scrap reaches a certain length, a real-time alert notifies stakeholders, and the problem is addressed before the downtime occurs.
Energy management
It’s no secret that the energy sector is experiencing major upheaval. Whether it’s meters, turbines, gas/oil pipelines, or solar panels, this equipment is outfitted with sensors. But this is only helpful with a solution that can handle the massive amounts of real-time data generated by these devices.
A gas storage and transportation company updated their business model to include a multi-energy mix, as it was clear that their legacy infrastructure wasn’t set up to handle their evolving needs. Their legacy on-prem server, firewall, and data historian were difficult and expensive to set up and manage, created lag times between ingest and analysis, and didn’t integrate with other technologies which made it challenging to add new software when necessary. After the company replaced their legacy data historian with a time series database, they experienced the benefits of real-time metrics, unrestricted data ingestion, and better ecosystem integration. The gas storage and transportation company found the benefits of working with a time series database so successful that they built a customized data platform on top of the time series database. This platform is now available as a software-as-a-service platform so other companies can also experience its benefits.
Time series is the future
Data is everywhere and collecting it is fairly straightforward these days. The challenge now is getting the most value from that data to better inform decisions. This includes using that data to stay ahead of the curve and having the ability to notice details that were previously imperceptible.
Time series data points the way forward by addressing all those challenges. In order to get the most out of time series data, businesses need a time series database due to the very specific nature of time series workloads. Time series data requires a purpose-built tool with features specifically designed to extract the immediate, meaningful insights that such data makes possible.