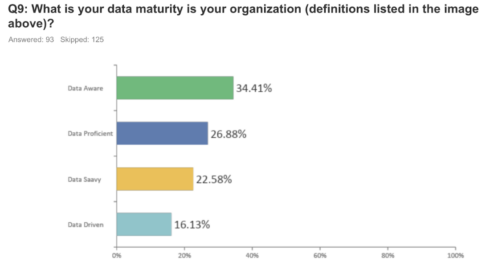
The Data Quality 2023 Study study reveals that a significant 34% of the organizations responding are at the ‘Data Aware’ stage, indicating they are in the initial phases of recognizing the importance of data but have not yet fully integrated it into their decision-making processes.
However, the most advanced stage, ‘Data Driven’, where data is fully integrated into those processes at all organizational levels, is achieved by 16% of the respondents’ organizations. This stage represents the pinnacle of data maturity, where data is utilized as a critical asset for business strategy and operations.
The study, compiled by SD Times and data quality and address management solution provider Melissa, garnered a total of 218 complete responses. The dataset provided a comprehensive overview of various aspects of data quality management, including challenges faced by organizations, time spent on data quality issues, data maturity levels within organizations, and the impact of international character sets.
When it comes to the most common issue when managing data, international character sets are the most prevalent challenge, with 28.1% of respondents rating it as their primary data quality challenge. Interestingly, international character sets were very prominently listed as both very difficult and the least difficult challenges at their organization at 28% and 37.5%, respectively.
International character sets present unique challenges in data quality management, primarily due to the complexity and diversity of languages and scripts they encompass. One of the primary issues is encoding, where different standards such as UTF-8 or ASCII are required for various languages.
Incorrect or mismatched encoding can result in garbled text, information loss, and complications in data processing and storage. Additionally, the integration and consolidation of data from multiple international sources can lead to inconsistencies and corruption, a problem especially pertinent in global organizations.
The second and third most difficult challenges for organizations were incomplete data and duplicates, with 22% and 23%, respectively, of respondents rating it the highest difficulty score.
This year’s study, the third of its kind, shows that organizations still struggle with the same issues they’ve been wrangling with over that time. “To me, this shows that organizations are still not understanding the problem on a macro level,” said David Rubinstein, editorial director of D2 Emerge, the parent company of SD Times. “There needs to be an ‘all-in’ approach to data quality, in which data architects, developers and the business side play a role in ensuring their data is accurate, up to date, available and fully integrated to provide a single pane of glass that all stakeholders can benefit from.”
According to the study, 54% of respondents indicated they are fully engaged in multiple areas of data quality. This suggests a comprehensive approach to data quality management, where professionals are involved in a range of tasks rather than specializing in just one area.
The key areas of involvement include Data Quality Management (48.9%), Data Quality Input (45.9%), and Data Integration (47.9%).
Data Quality Management involves overseeing and ensuring the accuracy, consistency, and reliability of data. Data Quality Input focuses on the initial stages of data entry and acquisition, ensuring that data is correct and useful from the outset. Data Integration involves combining data from different sources and providing a unified view.
A smaller proportion of respondents, 33.6%, are involved in Choosing Data Validation API Services/API Data Quality Solutions, reflecting the technical aspect of ensuring data quality through application programming interfaces and specialized software solutions.