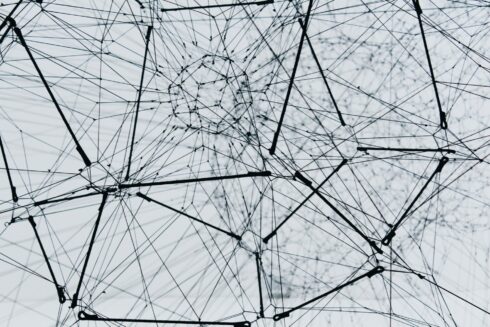
The age of Big Data inevitably brought computationally intensive problems to the enterprise. Central to today’s efficient business operations are the activities of data capturing and storage, search, sharing, and data analytics. Get these wrong and chances are your enterprise processes and systems will suffer. So would your bottom line, let alone the ability to create a competitive advantage.
Poor data management, data silos, and a lack of a common understanding across systems and/or teams are the root cause that prohibits an organization from scaling the business in a dynamic environment. It also prevents them from maximizing ROI, reducing costs, and building/sustaining efficient processes and software solutions that underpin critical operations.
As a result, organizations have spent untold money and time gathering and integrating data. While Big Data was all the rage, now “small and wide” data is the focus, giving more specificity to the information being developed. From that perspective, the priority has shifted from accumulating as much data as possible, to how can the organization create meaningful data. However, for this to happen, there needs to be context for the data to become knowledge.
Context: The Key to Making Data Useful
It goes without saying that data without meaning can yield incorrect insights, leading to potentially dangerous decisions. Beyond that, and without a way to visualize, connect, and utilize the data, it’s still just a bunch of random information.
Without metadata management and other data-related operations with semantic technologies, organizations often struggle to connect data sets and achieve a unified view of their enterprise data. Disconnected data, difficulty accessing it, and incomplete information, result in delays in decision making and often inaccurate decisions. Worse, and according to Gartner, upward of 80% of enterprise data today is unstructured which further exacerbates the loss of knowledge, insights, and the wisdom needed to make effective business choices. As a result, organizations are looking for fresh data integration approaches to challenge the mindset with which we created them.
Enter the Semantic Edge Era: How to Derive Value from Semantic Metadata
The problem with Big Data is not the data itself. Rather, it is the inability to develop and utilize meaningful connections across that data, in other words – it lacks semantic metadata. To be able to derive and deliver value from data, the business needs to establish a trusted foundation that is shared and accessible across the enterprise, with context to drive usage. Built with semantic metadata, which is metadata expressed with formalized, agreed upon meaning, users can share and agree upon the meaning of data so it becomes more valuable. After all, enhancing data with standardized meaning and identifying relationships among data types helps organizations better understand and access data. It also creates a knowledge base to power growth through well-defined context and coherent systems.
Additionally, applying semantic metadata removes the vicious circle of drowning in data but thirsting for information. By establishing a layer on top of existing enterprise systems and data warehouses, semantic metadata unlocks incredible new ways to interact with information, forging new experiences out of exploration and discovery. Through integrated sets of vocabularies, taxonomies, and ontologies, as well as harmonized and interlinked previously heterogeneous sources, semantic data expands the value of existing data in multitudes.
Technicalities aside, semantic metadata alleviates a lot of data integration and management problems by simply encoding information across data pieces that link between data components. As a result it turns them into the type of data that can be managed programmatically while containing all the agreed upon meanings for human reference. With semantic metadata, enterprise data gets linked to one another and to external sources. Using machine readable definitions, it creates a highly interconnected data object that delivers high value and meaning as well.
How to Get a Semantic Edge?
Now that all enterprises are data producers and their stakeholders are data consumers, strategizing data management and integration with clearly defined semantics in mind is critical. While all data integration and management solutions ultimately seek to reduce costs, increase performance levels, and generate additional value, few succeed in doing so. One of the greatest obstacles for increasing efficiency is the disconnected nature of heterogeneous data sources and the exponentially increasing data volumes. As a result, enterprises require information management solutions that facilitate the integration and interlinking of heterogeneous data, so they can derive value from all the information they manage.
Semantic metadata provides this by allowing a higher level of abstraction where deeper understanding of the data relationships is achieved. Semantically integrated data makes metadata meaningful, allowing for better interpretation, improved search, and enhanced knowledge-discovery processes. It involves bringing together different pieces of information from various sources and making them work in concert. It also includes importing and organizing diverse data types, then connecting them into a graph database, using semantic technology.
Semantic Technologies To The Rescue
Establishing context for enterprise data that describes information with meaningful metadata, starts with the understanding that it is a long-term effort that requires team participation. Agile and iterative engineering and flexible stakeholder management is required as well.
Starting small is key, so many organizations start with one or two datasets, define their underlying model, describe their facets and formalize the relationships between the data pieces in each dataset. They also tend to approach the initial phase of building a semantic metadata edge with one or two major data challenges in the enterprises which makes it easier to tackle the complexity inevitably involved in each business case. And just like business models vary, semantic metadata projects have their unique characteristics.
Like signing a machine-readable contract, semantic data schemas serve as contracts to enable correct interpretation and diligent management of the data. It is through that contract that semantic metadata helps enterprises interconnect and harmonize siloed data, building context and as a result, a competitive advantage. By leveraging semantic metadata, organizations can better understand and access all of their data with context to gain a shared understanding and description of the data developed.