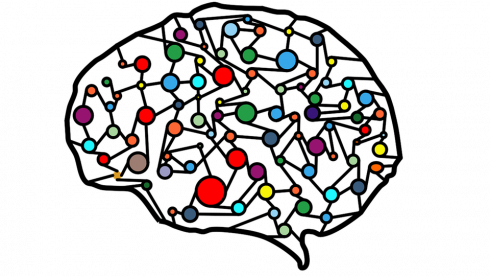
Enterprises of all sizes are looking to leverage machine learning, but not everyone is finding immediate success. A newly released report revealed larger organizations are finding more success compared to smaller ones.
The report, the State of Enterprise Machine Learning, by Algorithmia surveyed more than 500 data science and machine learning professionals to gauge how companies are utilizing the technology.
The report found data science and machine learning professionals in larger organizations with more than 2,500 employees feel more satisfied with their progress. According to Algorithmia, in larger organizations professionals are 300 percent more likely to consider their deployment model sophisticated, and are 80 percent more likely to say they are satisfied or very satisfied with their progress compared to smaller companies with 500 or less employees.
“In 2018, large enterprise companies have an advantage when it comes to machine learning because they have access to more data, can continue to invest in big R&D efforts, and have many problems that machine learning technology can solve cost-effectively,” said Diego Oppenheimer, CEO at Algorithmia. “And yet, even in the largest companies, productionizing and managing machine learning models remains a challenge. Productionizing models is seen as the last step to ROI. Without an enterprise platform to help, these companies are missing out on the rewards of machine learning.”
Ninety-two percent of respondents in organizations with 10,000 employees or more stated machine learning investment within their company has grown by at least 25 percent. The biggest use cases for machine learning here include increasing customer loyalty, increasing customer satisfaction and interacting with customers, according to the report.
The report also looked at challenges companies are facing when it comes to implementing machine learning. The report found product challenges are a considerable challenge with teams either spending too much time on infrastructure, deployment and engineering; or not enough time on training and iterating models. Thirty-eight percent stated problems with scaling models because of resources and lack of existing infrastructure. Thirty percent complained about the different programming languages and training frameworks necessary for machine learning models. And another 30 percent reported problems with model management tasks like versioning and reproducibility.
“In general, larger companies have more machine learning use-cases in production than smaller companies,” said Oppenheimer. “But across the board, all companies are getting smarter about where and how to apply ML technology. We expect to see big leaps in productionized machine learning over 2019 as data scientists can more easily deploy and manage their models.”
Other findings include the focus on reducing costs is higher with sophisticated adopters, and large organizations are putting efforts into using data science for cost savings.