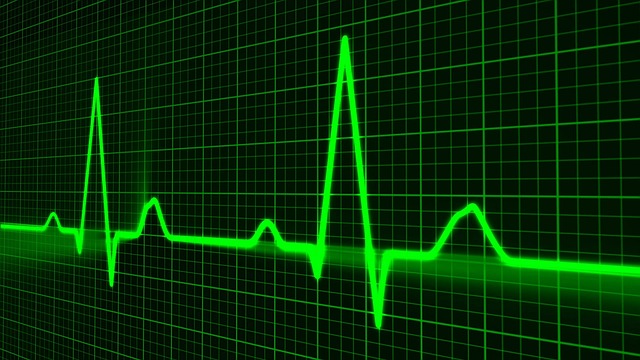
Stanford computer scientists believe they have developed an algorithm that can diagnose heart arrhythmias with cardiologist-level accuracy. The new deep learning algorithm sifts through hours of data to find irregular heartbeats.
Typically, arrhythmias are detected with an electrocardiogram, but doctors often prescribe a wearable ECG to continuously monitor a patient’s heartbeat. This wearable device results in hundreds of hours of data. The researchers worked with a heartbeat monitor company, iRhythm, to train a deep neural network model that could accurately detect irregularities in a massive dataset.
According to the researchers, the algorithm is capable of diagnosing 14 different types of heart rhythm defects better than some trained cardiologists. They hope this will help speed up diagnosis, and improve treatment. In addition, the researchers say this could benefit people in remote locations that don’t have access to cardiologists.
“One of the big deals about this work, in my opinion, is not just that we do abnormality detection but that we do it with high accuracy across a large number of different types of abnormalities,” Awni Hannun, a graduate Stanford student, said in a statement. “This is definitely something that you won’t find to this level of accuracy anywhere else.”