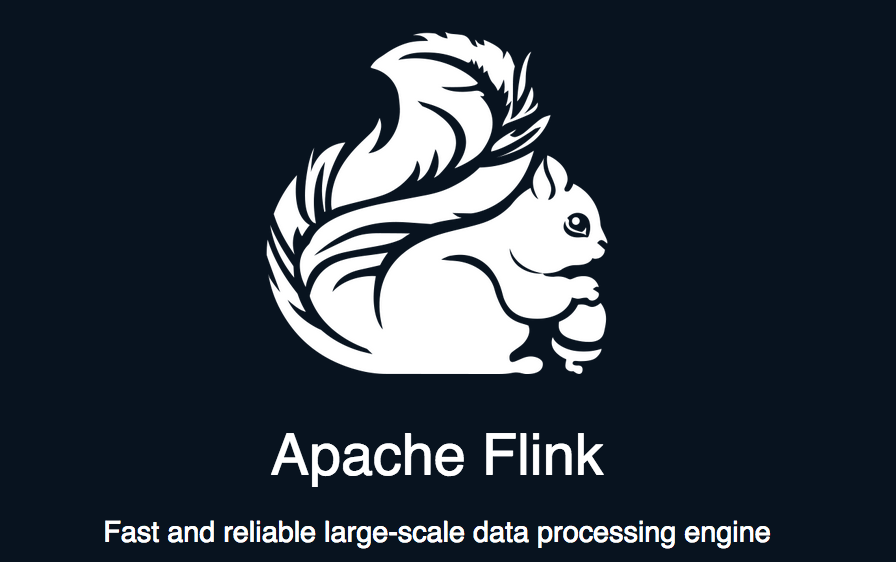
The Apache Software Foundation has announced Apache Flink as a Top-Level Project (TLP).
Flink is an open-source Big Data system that fuses processing and analysis of both batch and streaming data. The data-processing engine, which offers APIs in Java and Scala as well as specialized APIs for graph processing, is presented as an alternative to Hadoop’s MapReduce component with its own runtime. Yet the system still provides access to Hadoop’s distributed file system and YARN resource manager.
The open-source community around Flink has steadily grown since the project’s inception at the Technical University of Berlin in 2009. Now at version 0.7.0, Flink lists more than 70 contributors and sponsors, including representatives from Hortonworks, Spotify and Data Artisans (a German startup devoted primarily to the development of Flink).
The project’s graduation to TLP after only nine months in incubation raises questions about not only its future, but also about the potential it may have for the evolution of Big Data processing. SD Times spoke with Flink vice president (and CTO of Data Artisans) Stephan Ewen and Flink Project Management Committee member Kostas Tzoumas (also the CEO of Data Artisans) about where Flink came from, what makes the Big Data engine unique, and where the newly minted TLP is going.
SD Times: Flink is an open-source distributed data analysis engine for batch and streaming data with Java and Scala APIs. In your own words, describe what Flink is as a platform.
Ewen: Flink, as a platform, is a new approach for unifying flexible analytics in streaming and batch data sources. Flink’s technology draws inspiration from Hadoop, MPP databases and data streaming systems, but fuses those in a unique way. For example, Flink uses a data streaming engine to execute both batch and streaming analytics. Flink contains a lot of compiler technology, using Scala macros, Java reflection, and code generation together with database optimizer technology in order to holistically compile and optimize user code similarly to what relational databases do.
Tzoumas: To the user, this technology brings easy and powerful programming APIs and a system with state-of-the-art performance, which also performs reliably well in a variety of use cases and hardware settings.
Talk about the origins of Flink and the inspiration for its creation. How, if at all, has the project’s core and focus evolved over the past several years?
Tzoumas: In 2009, researchers at TU Berlin and other universities started playing with Hadoop and asked themselves, how can we bring together knowledge from the database systems community and the Hadoop community in a hybrid system?
Back then, Hadoop was fairly new—only MapReduce and quite hard to use—while SQL databases were more established but clearly could not cover some new use cases. During the years, the team built a system that intelligently fuses concepts from the Hadoop and the SQL database worlds—without either being a SQL database or being based on Hadoop—called Stratosphere. The project gained momentum as an open-source GitHub project, and in April 2014 the community decided to submit a proposal to the Apache Incubator. During the years, the scope of the project of course expanded somewhat to cover both streaming and batch data processing, and to provide a smooth user experience to developers that use it, both for data exploration and for production use.
What makes Flink unique in regards to data streaming and pipelining? What does the technology offer, or what use cases does it enable that makes it stand out among similar Big Data technologies?
Tzoumas: The combination of batch and true low-latency streaming is unique in Flink. Combining these styles of processing, often termed “lambda architecture,” is becoming increasingly popular. Unlike pure batch or streaming engines, through its hybrid engine Flink can support both high-performance and sophisticated batch programs as well as real-time streaming programs with low latency and complex streaming semantics.
Ewen: In addition, Flink is unique among Big Data systems (and perhaps unique in the open-source Java world) in how it uses memory. Flink was designed to run very robustly in both clusters with plenty of RAM, and in clusters with limited memory resources, or where data vastly exceeds the available memory. To that end, the system manages its own memory and contains sophisticated Java implementations of database-style query processing algorithms, traditionally written in C/C++, that can work both on in-heap and off-heap memory.
How has the open-source community around Flink grown and developed over the past several years?
Tzoumas: The Flink community came initially from the academic world: [the] Technical University of Berlin, Humboldt University of Berlin, [the] Hasso Plattner Institute. As the project gained more exposure, more people from industry joined the project. Recently, a group of Flink committers started Data Artisans, a Berlin-based startup that is committed to developing Flink as open source. We have been very excited about how the community has been developing. The number of contributors to the project has almost doubled since the project went to the Apache Incubator.
What does this milestone of ascension to Top-Level Project mean for Flink? Where does Flink go from here?
Ewen: Graduating to a Top-Level Project is a very important milestone for Flink as it reflects the maturity of Flink both as a system and as a community. The Flink community is currently in its public developer mailing list discussing a developer road map for 2015, which includes several exciting new features in the APIs, optimizer and runtime of the system. In addition, the community has started to put a lot more focus on building libraries, e.g., a Machine Learning library and a Graph processing library, on top of Flink. This work will make the system accessible to a wider audience that will in turn feed back to the community.