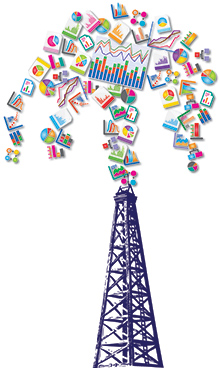
It’s been nine years since former Yahoo search technologist Doug Cutting and computer scientist Mike Cafarella started Apache Hadoop, based on Google’s novel scaling of the 1970s-era Map/Reduce algorithm for data searches on cheap server clusters. That’s about halfway toward the typical 20-year maturity cycle, according to Gartner Big Data analyst Merv Adrian.
Recently he told a SiliconANGLE reporter at the Hadoop Summit that “irrational Hadooperance” is subsiding, and “we are moving from a blunt instrument for processing to a nuanced stack.” But if, as he recommended, it was time to join this ecosystem, it didn’t translate to making a major investment: By his estimates, fewer than 800 customers are currently paying for Hadoop services or distributions.
As Hadoop captures the fancy of futurists, fabulators, inventors and investors alike, the scramble has begun both to capitalize on a new data ecosystem and re-inject relevance into existing enterprise business intelligence solutions.
The age of data deserves better BI
The IT industry has progressed from the iron age (mainframes and minicomputers) to the software age (operating systems, databases and applications) to the age of data, according to Mike Hoskins, CTO of Actian. Google, Apple and Amazon epitomize this content-delivery era, though the search-engine giant is the only one built primarily on data analytics.
If, as Tim O’Reilly posits, data is the new oil, the question isn’t where to find it, but what to ask it. Plenty of Hadoop-era startups like Pentaho, Splunk, TIBCO Jaspersoft and more are angling to answer.
(Related: Big Data’s also in the cloud)
San Francisco’s Alpine Data Labs, founded in 2011, is a perfect example of the new breed of Big Data BI. Its flagship product, Chorus, simplifies the process of building predictive models for Big Data, which has become so cheap to store.
“When you store more data, you will ask more questions, but with this power comes great responsibility,” to paraphrase the comic and movie hero Spider-Man. Bruno Aziza, Alpine Data Labs’ chief marketing officer, put it this way: “You have the risk of a lot more noise. That’s where the BI approach to Big Data breaks. You can’t just be reporting; you must be more descriptive. We’re about to get into the third stage of this industry, where algorithms are going to take control. The companies that can run algorithms at scale are the ones that are going to grow faster.”
Contrast Alpine with Actian. If Alpine is a typical startup, Actian is a classic database survivor, built on a long line of companies dating back to Relational Technology, Inc., a former Oracle competitor founded in 1980, later renamed Ingres Corp. and rebranded Actian in 2011. As a testament to its adaptability, a series of acquisitions in the last few years have reoriented the BI company toward Big Data processing, most notably with its 2010 purchase of VectorWise, founded by large-scale analytical data-management professor and researcher Peter Boncz.
According to Actian, the Hadoop ecosystem is playing catch-up to cutting-edge analytics technology so that it can reach business analysts.
“People have already proven that Hadoop is affordable and scales. They never kept that data before, and now they’re throwing everything into Hadoop and doing discovery analytics to find things that weren’t recognizable in human terms. But about 60% of those Big Data projects are without a business case. They’re still in the lab and haven’t made it into production,” said John Santaferraro, vice president of marketing for Actian.
If only it were easier to get SQL access to data that lives in Hadoop, observed Santaferraro. “Every business has a conduit to data that the business analyst uses: SQL. Now we’ve flung that door wide open with high-performance SQL access to data right in Hadoop with our Vector database product,” he said.
A number of open-source projects have aimed to support fast BI on top of Hadoop, but Actian claims to have beaten these. “Impala and Hive: Both projects refer back to research by Peter Boncz in the 2000s, who built out a 100x engine with vector processing,” said Santaferraro. “But they’re starting from scratch. We started building in the mid-2000s, released in 2010 and have up to 30x better performance than Impala has, along with ACID compliance, security, things that anybody using a database and doing operational BI need. But imitation is the sincerest form of flattery.”
Intelligent vs. smart
Though “Big Data” has possibly exceeded “cloud” as an over-hyped technology catchall in the last two years, the data tide has not lifted all BI boats. “Business intelligence” took off as a term in the late 1990s, evolving from decision support systems of the 1980s. Some experts now say BI is bifurcating again, thanks to Big Data, and the winners will be in “data discovery,” not BI.
“Visual data discovery took center stage in 2013, with specialty vendors (Tableau, QlikView, TIBCO Spotfire) growing rapidly ,and mega vendors (SAP, IBM, Microsoft, Oracle, MicroStrategy, SAS) stagnating,” blogged Cindi Howson, founder of the BI analyst firm BI Scorecard.
“BI heavyweights have taken notice that more agile and visual solutions have eaten into their bread-and-butter, query-and-reporting market share. All have responded with new interfaces and solutions.” But it’s hard to balance between what she termed “agile yet trusted data—user-driven but IT-controlled.”
Despite the buzz around discovery and personalization, traditional BI tools haven’t seen an uptick in adoption, according to Howson’s survey of 513 respondents in 2013. Her firm measured adoption as a percentage of total employees at 22%, down several percentage points since the survey was first conducted in 2006. That dovetails with findings in the Gartner Magic Quadrant for Business Intelligence and Analytics Platforms report of February 2014. The study claimed that large-scale, IT-centric systems-of-record reporting with online analytical processing and ad hoc query that traditionally comprised BI never caught on. Rather, a five-year trend shows legacy BI platforms are being complemented or replaced by “business-user-driven data discovery techniques.”
From IT monitoring to BI
There’s another market movement afoot, thanks to the Big Data hype. Increasingly, application-monitoring tools are putting their foot in the door left ajar by BI and Hadoop. Splunk, New Relic and AppDynamics are among application intelligence tools that are encroaching on BI. The claim? That software-driven businesses need data about the health of their products, not just the happiness of their customers. These tools can convert obscure IT scenarios into clear business conversations.
Take the example of a recent AppDynamics customer situation: a large movie ticket vendor using application performance management (APM). “Our APM detected an outage using real-time business collectors and began computing revenue lost per minute,” said Adam Leftik, director of product management for AppDynamics.
“IT was no longer talking the language of ‘There’s a JVM outage.’ It was, ‘Ticket sales have dropped from US$5 million per minute to zero.’ We’re changing the way that people talk about the system in general, no longer dealing with key performance indicators that are tech-related, like load in terms of calls per minute, or average response time, but in business terms. It’s all about revenue and business impact that owners understand.”
Collecting so-called “machine data” has broader utility in the age of Hadoop. Tapan Bhatt is vice president of retail systems at Splunk, an app-monitoring company founded in 2004 whose event and machine data analysis has been expanded with a new product, Hunk, which works to dice and visualize batch data stored in Hadoop.
“Machine data, to us, is broad,” he said. “It might be coming from app servers, mobile devices, RFID chips, sensors on buildings or cars. It’s not human-generated data entered in a CRM system. The founders of Splunk came from running data centers, and the original use was IT ops.”
There are six main uses for Splunk, said Bhatt. “First, app management; second, IT operations; third, security and compliance. After that come digital intelligence, business analytics and Internet of Things. The first three are where we started. The next three are emerging.”
What will Big Blue do? Watch Watson
No other modern software company has the database history that IBM does. Starting with the Tabulating Machine Company, founded in the 1880s—one of the three separate companies that merged to become the future IBM in 1911—Big Blue has been at the forefront of data analytics. Looking at its installed base of mainframe customers and enterprise database users, it would be easy to discount IBM’s ability to turn on a dime. Enter Watson.
Though these are early days for the next-generation BI tool, who can resist the promise described on its website: “Watson is a cognitive technology that processes information more like a human than a computer—by understanding natural language, generating hypotheses based on evidence and learning as it goes.” In early 2014, IBM unveiled the Watson Business Group, comprising 2,000 employees and a $1 billion investment, to hail what Michael Rhodin, senior vice president of the IBM Watson Group, called the third era of computing: cognitive computing.
The May 2014 acquisition of Cognea, an artificial intelligence startup, brings on virtual assistants sure to complement Watson, IBM’s “Jeopardy” question-answering supercomputer. Though all this seems far afield from BI, a recent Gartner report ties Watson Analytics to the “smart data discovery” trend, noting the aging IBM Cognos BI platform.
Ironically, as Big Blue boldly goes where no BI has gone before, Hadoop sellers Cloudera and Hortonworks are busy becoming staid. Cloudera has brought on data warehousing guru Ralph Kimball to help architect something useful around overflowing, underperforming Hadoop “data lakes.” And Microsoft aims to bring Big Data to a billion users in a partnership with Hortonworks.
With Excel, Microsoft already has the world’s most popular—albeit puny—data analysis tool. But the company’s Azure-and-mobile play is innovating BI access for all, while it takes advantage of the Big Data hype to remind corporations of the speed and power of proprietary in-memory OLTP for SQL Server 2014.
Finally, for starry-eyed types, Microsoft CEO Satya Nadella did not disappoint when he announced the limited public preview of Azure Intelligent Systems Service in April. The Internet of Things is a popular aspiration for Big Data futurists, though many pragmatists note that much more basic information (such as “How many orders did we fill yesterday?”) is still beyond the grasp of many companies.
Mining the hype
To a degree, the hyping of Big Data is itself a symptom of data generated by click-baiting headlines that would be rendered powerless with sentiment-based algorithms. A case in point: The canny, possibly apocryphal, description fed to New York Times reporter Charles Duhigg by an unnamed Target employee of the store visit by a father irked by the ultimately correct direct mail his pregnant daughter received. That 2012 article, which helped Duhigg’s book “The Power of Habit” become a best-seller, is now part of the Big Data gospel. But even if the predictive analytics that buying patterns reveal are ever more useful, no algorithm comes without false positives.
“We should not buy the idea that Target employs mind-readers before considering how many misses attend each hit,” writes Tim Harford in FT Magazine. (Interestingly, in its 2013 white paper on Big Data journalists, LexisNexis reveals that the Financial Times had published 49 articles on Big Data in the previous two years, making it the leading U.K. outlet covering the topic. Too bad the analysis didn’t delve deeper into, say, sentiments expressed, tools covered or sources cited.)
The 2012 U.S. presidential election was another moment where (Obama for America CTO Harper Reed’s current protestations notwithstanding) data played a starring role in the victory. Witness self-taught statistician Nate Silver’s subsequent best-seller “The Signal and the Noise,” which played up the role of Bayesian inference in making predictions such as his successful bet on Obama’s win. Suddenly, stats were exciting again, and fevered debates over Fisher vs. Bayes, among other flavors of statistical design, took off.
Damn lies, and other statistics
In the O’Reilly book Big Data Now: 2012 Edition, UC San Diego cognitive science professor Bradley Voytek describes a 2008 scientific paper that found that neuroscience papers with brain scan images were perceived as more credible than those without.
“This should cause any data scientist serious concern,” he writes. “In fact, I’ve formulated three laws of statistical analyses:
1. The more advanced the statistical methods used, the fewer critics are available to be properly skeptical.
2. The more advanced the statistical methods used, the more likely the data analyst will be to use math as a shield.
3. Any sufficiently advanced statistics can trick people into believing the results reflect truth.”
At the O’Reilly Strata 2014 conference, author David McRaney told a compelling story about how the Department of War Math in World War II, populated by women statisticians, calculated survival rates of bombers. An insight derived from their work was survivorship bias, which blinds us to studying factors contributing to failure not from the winners but, more importantly, the losers.
Who are the new data scientists?
Contrary to popular belief, the term “data scientist” doesn’t mean “hipster”—though some who hold the title come awfully close, like UC San Diego cognitive science professor Bradley Voytek, who is both an academic and a data evangelist for the Uber ridesharing startup. The term is quite a bit older than that, in fact.
“‘Data scientist’ dates back to 1974, when computer scientist Peter Naur used it,” said Carla Gentry, founder of Analytical Solution in Louisville, Ky. She has more opinions about the topic than a BI vendor can shake a stick at, and has shared them widely on blogs and at conferences.
“Data science is more than data mining. It’s not as easy as you think it is,” she said. I wrote a blog called How to Hire a Real Data Scientist. Big Data means data science, but data science doesn’t necessarily mean Big Data.”
Gentry added that the term took off in popularity around 2011.
“If you add visual and unstructured text, then of course there’s more. Yes, you have been tracked. But it’s really nothing new. We have a little more data, and more ways to look at it, like sentiment analysis,” she said.
“I keep saying the sexy job in the next 10 years will be statisticians,” said Google chief economist Hal Varian in 2009. “The ability to take data—to be able to understand it, to process it, to extract value from it, to visualize it, to communicate it—that’s going to be a hugely important skill in the next decades.”
He was right. According to Gartner analyst Mark Raskino, in 2014, Chief Data Officer “is one of those titles that has been rising in visibility over the last two or three years,” he blogged. To be precise, he found more than 100 chief data officers in large companies, “more than double the number we counted in 2012.”
An interesting aspect of data science is that it is noticeably more female than computer science. Raskino reported that more than 25% of those holding the exact “chief data officer” title are women, while only 13% of CIOs are women. Women are also more prevalent as chief data scientists and BI consultants. Could a preference for statistics and math be one reason for relatively more women? A 2011 National Center for Education Statistics survey found that while women earned less than 20% of all computer science bachelor’s degrees, and just under 30% of master’s degrees, they make up more than 40% of undergraduate and master’s degree earners in mathematics and statistics.
Is questioning a human condition?
Perhaps the bigger question many have is not whether more women or men are drawn to statistics, but whether humans or machines will be the ones asking the questions. (Even Stephen Hawking recently expressed concern that allowing AI to develop unabated posed grave risks to the future of humanity.) For the short term, anyway, it seems unlikely that even pre-sentient supercomputers like Watson will replace humans anytime soon. After all, it’s our insatiable appetite for entertainment that drives this era of content on computers, right? Or is it our attention span?
“I prefer a different definition of Big Data, and that is that Big Data is data made useful,” said data scientist Hilary Mason to the crowd at Mort Data’s first New York City data science meetup in February 2013. “In the case of deploying specialized infrastructure to count things, like having a Hadoop cluster, it means we can ask a question at the data and get the answer back before we forgot why we asked the question in the first place. It’s a human problem. Big Data is a technical solution to a human problem, and that is our inability to pay attention to things for very long. We’re really just little data monkeys.”
Dark Data joins the fray
Despite its ominous name, coined a few years ago and now widespread across the Web, dark data isn’t something most people lie awake worrying about. That’s just the problem, according to Boulder, Colo.-based Parascript. Whether they are insurance adjusters dealing with tornado relief, social workers visiting homes, farmers assessing crops, or oil and gas experts in the field, many professionals submit handwritten notes to corporations, which in turn fail to do anything further with the information.
“The category of dark data is very broad. Gartner says it’s data you collect, process and store, but fail to use for other purposes,” said Don Dew, director of marketing for Parascript, who said that call data and images can also be included in this category. His company’s longstanding handwriting-recognition solution, already employed by the US Postal Service, FiServ, IBM and others, could be part of a solution designed to convert the meanings of dark data into something businesses can act on.
A recent Web survey commissioned by Parascript of 385 members of the Association for Information and Image Management (AIIM) found that, of the 73% of survey respondents who scan forms, only half do text recognition. Twenty-five percent only scan to archive, and the remaining 25% workflow scan images but manually re-key the data. But the use of captured text for archive indexing has grown from 64% to 87% (of those who do use text recognition) since 2012.
“Dark data is starting to get a lot of mileage,” said Bob Larrivee, director of custom research at AIIM. “The idea is that all this information is sitting out there, but nobody accesses it and gains the maximum benefit from it.” Possible applications range from fraud detection, large analog conversions, litigation discovery, signature detection or comparison, and sentiment analysis of hand-written annotations to documents.
“This study reinforced that we’ve used handwriting recognition on well-defined applications like check and mail processing, but it starts to highlight the potential for this,” said Dew. “As the report points out, actual adoption has been pretty low.”
Parascript’s technology, like the data it wants to discover, has been made faster and more accurate thanks to neural networks and speedy processors.
Ultimately, the promise of Big Data discovery would be to associate dark data with tags that help point it toward the people who can use it, said Larrivee. “The realm of knowledge management has been around for a long time,” he said.
“In it’s purest sense, it means you wouldn’t have to look for it; it would find its way to you based on profiles. When something enters the enterprise, we extract that dark data, and it becomes one of those elements that says ‘I recognized what this is, and there’s a couple of profiles that want this information.’ ”