NoSQL databases continue to proliferate as the demand for Big Data solutions grows. While relational databases aren’t going away anytime soon, different data models require different types of solutions. As a result, several types of NoSQL databases have emerged, each with its own pros and cons.
“It’s important that you’re not just going with a traditional database because that’s what everyone else is using,” said Evaldo de Oliveira, business development director at FairCom. “Pay attention to what’s going on in the NoSQL world because there are some problems that SQL cannot handle.”
NoSQL databases have been gaining momentum because organizations want the ability to query unstructured and semi-structured data, and they want to take advantage of database technologies that were designed for the Web and Big Data. NoSQL solutions are generally open source, provide linear scalability across commodity hardware, and ensure high availability through distribution and replication. Many of them also store data in a schemaless manner.
The four major types of NoSQL databases are key-value stores, document stores, wide column stores, and graph stores. Some of them, particularly key-value stores, may be broken down further into subtypes depending on who is classifying them. It is also worth noting that some NoSQL databases span more than one category and some of them also support SQL queries.
For example, HPCC is an open-source computing platform originally developed and contributed to by LexisNexis Risk Solutions. It is programmable and supports different data models. LexisNexis Risk Solutions typically uses a row-oriented layout for its own data services, although HPCC also supports columnar and adjacency table (graph) layouts as well as multi-key retrieval, according to Flavio Villanustre, vice president of information security at LexisNexis Risk Solutions. HPCC also supports SQL queries.
Couchbase Server combines a key-value store with a JSON document store and caching. It is also a mobile JSON document store, said Rahim Yaseen, senior vice president of products at Couchbase. Couchbase Server combines elements of Memcached, the open-source, high-performance, distributed memory object caching system, with elements of the Apache CouchDB document store, along with proprietary enhancements.
Meanwhile, FairCom c-treeACE is a key-value store, but unlike most NoSQL databases that are non-transactional, c-treeACE is a fully consistent NoSQL transactional database that provides SQL capabilities on top of its NoSQL main core technologies. According to FairCom’s de Oliveira, c-treeACE combines the NoSQL benefits of high performance, low latency and precise data access control with the flexibility of SQL interfaces. The SQL support allows organizations to integrate with SQL environments such as reporting, BI and data warehouses.
Which NoSQL database or combination of databases organizations use varies, which has created a market for the expanding number of database types and hybrid implementations.
Pythian, a provider of data-management consulting and managed services, wisely takes a problem-first approach to identifying potential solutions. Its customers use document stores for user profiles and news articles, and key-value stores for counters and time series data.
“Most people who choose NoSQL as their primary data storage are trying to solve two main problems: scalability and simplifying the development process,” said Danil Zburivsky, solutions architect at Pythian. “Despite all the efforts, working with relational databases still feeds ‘awkward’ in most programming languages.”
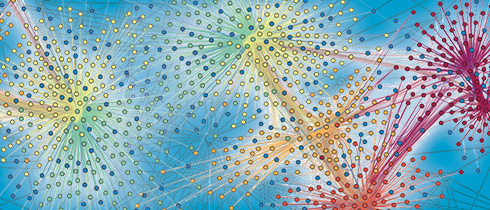
#!Key-value stores
Key-value stores are the most basic type of NoSQL database. They are fast and highly scalable but have less built-in intelligence than wide column stores or document stores, for example. Key-value stores assign a key and a value to an item or a BLOB n a database, and retrieve it using a key.
“If I’m looking for a specific piece of information or want to search for a specific piece of information in a BLOB or all BLOBS, it’s not set up to do that,” said Kurt Cagle, principal evangelist for semantic technologies at Avalon Consulting. “If I’m interested in the entire thing and I can do it one key at a time, it’s great.”
Key-value stores are used in many industries for purposes ranging from gaming to payment authorizations.
“Key-value stores provide simple key-based storage and retrieval,” said LexisNexis’ Villanustre. “They tend to be fast and easy to distribute and parallelize, but they suffer drawbacks in two specific cases. If the key is not completely known and you have to use wildcards, or when the retrieval query needs to use the intersection of multiple keys to identify a candidate set, performance drops considerably because of the large size of the intermediate candidate sets.”
Document stores
Document stores store, retrieve and manage document-oriented information. They also use key-value pairs, but unlike key-value stores, they recognize objects (usually JSON objects) as documents and therefore do not require the document to be translated. However, the additional overhead taxes processing speed as compared to key-value stores.
“The JSON document model is a lot more in tune with where the Internet is,” said Couchbase’s Yaseen. “It came from JavaScript and is used not just to store data but for a lot of the processing done on the Internet (which is done in JSON documents).”
Madison Logic, which provides data and online lead-generation services, uses a document database to help model the intent of companies.
“We have complex models that contain varying amounts of information and varying types,” said Madison Logic’s CTO Mark Hershberg. “Because we’re always investigating new data sources to improve our models, we can’t be certain what data fields may be part of the model 18 months from now. Traditional databases don’t have [that] flexibility or in many cases the ability to scale to handle the models that we need. While column-based and document-based NoSQL databases made the most sense, we felt the document model provided the right amount of flexibility.”
Wide column stores
Wide column stores (a.k.a. column stores or columnar stores) are optimized for queries that span multiple columns of data (super columns) or multiple columns of related data (column families). Like relational databases, wide column stores organize data in rows and columns; however, the column orientation is better suited to certain types of queries. Like document stores, column stores share attributes with key-value stores.
“Wide column stores are particularly good at reducing the amount of disk seeks required for retrieving data,” said LexisNexis’ Villanustre. “Unfortunately, they are not very efficient when most of the columns are required as part of the candidate set.”
Wide column stores are better at handling sparse data than relational databases. For example, if a user profile contains a first name, last name and interests, some users may choose not to specify their interests. A relational database would require “no value” to be specified and the processing logic to handle that; a column store just accommodates the variance.
“One of the key advantages of columnar stores is the way you can store the information,” said Avalon Consulting’s Cagle. “You can compact information and deal with more sparse content. You gain flexibility, but it comes at a cost of less performance, and that’s the big trade-off with all of these [NoSQL databases]. There are very few that offer high performance, high scalability and high flexibility.”
Graph stores
Graph stores are used to explain relationships. Some graph stores use adjacency nodes where one node points to another; triple stores store graphs as subject-property-object. Graph stores are commonly used for network diagrams and social graphs.
“Graph databases tend to be optimized for referentiality. The problem is they’re a lot like assembly language once you start defining everything,” said Cagle. “A triple store is a way of representing an assertion so you can essentially build into these structures a data model between classes of things rather than just between instances.”
Wargaming.net is currently evaluating graph databases with the goal of understanding all of the relationships in its games.
“Who you play with is very important in online games,” said Craig Fryar, head of global business intelligence and the global business intelligence data engineering team at Wargaming.net. “Using [the graph database], we define relationships between players in the different ways they can interact with each other from platoon and clan membership, to chatting with and shooting at each other.”
Wargaming.net uses a combination of NoSQL database types, as does Zephyr Health. The company uses a document database, a graph database, a cache, a relational database, and a NoSQL database service to accommodate its wide range of use cases.
“We use a variety of NoSQL databases in a polyglot persistence model,” said Brian Roy, director of products at Zephyr Health. “We firmly believe that the primary lesson to be learned from the NoSQL movement is that no one database is appropriate across a wide range of use cases. Ultimately, this was the fatal flaw in the relational-database-for-everything approach.”
FairCom’s de Oliveira agreed, underscoring today’s need for performance.
“If you’re at the point where the service level is critical, don’t rely on complex SQL queries,” he said. “You have options available to handle it differently.”
The vendors featured in this Buyers Guide are available here. You can also find additional solutions analyzed here.