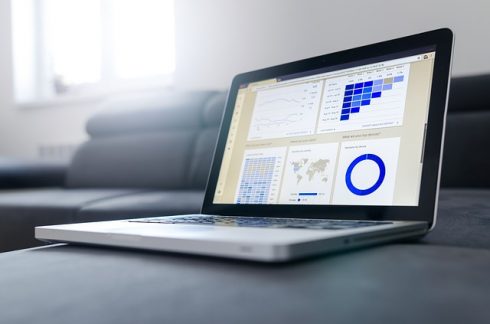
One of the remarkable consequences of the contemporary proliferation of data is the corresponding profusion of data-driven analytics across a wide range of industry verticals. In particular, research in verticals such as environmental science, education, pharmacology, medicine and public health differentially explore concepts such as correlation and causality as they relate to two or more variables.
For example, the contemporary profusion of data has enabled a surfeit of research that asserts a relationship between variable X and cancer, variables Y and Z and the longevity of a marriage or variable Z and a detriment or benefit to the environment. Concrete examples of such analyses include claims about the relationship between BPA and cancer, the financial investment in a wedding and the longevity of the associated marriage and vaccines and the onset of autism.
Historically, statisticians have been central to efforts to understand the statistical significance of correlative analytics that illustrate a relationship between two variables. That said, one of the notable consequences of the widespread adoption of business intelligence platforms is their diminution of the importance of the statistician and corresponding elevation of the ability of business users to derive actionable insights about large-scale datasets. In addition, business intelligence platforms absolve users of the need to write custom code and subsequently accelerate the derivation of analytic insights.
While the acceleration of the derivation of analytic insights, the democratization of data scientist related capabilities and enhanced data visualization functionality represent some of the key advantages of contemporary business intelligence and data analytics platforms, drawbacks include the diminution of considerations related to statistical or analytic methodology, significance and rigor. Another way of putting this would be to say that contemporary business intelligence platforms have ushered in the death of the statistician, and laid the foundation instead for data savvy, business users capable of rapidly wrangling through a massive dataset and understanding relationships between one or more variables by means of a panoply of rich and multivalent visualizations.
The prioritization of accelerated time to insight in contemporary business intelligence platforms and the corresponding evacuation of statistical rigor have facilitated the proliferation of spurious correlative analytics that confuse the distinction between correlation and causation. For example, recent analyses claim a correlation between an embryo’s exposure to deep ultrasounds and the onset of autism, the consumption of carrot juice and the amelioration of cancer and the ability of classical music to slow down dementia. While such studies may individually have merit with respect to the sample size upon which they operate, they evacuate deeper questions about the dataset in question, such as whether the correlation can serve as the theoretical foundation for causation on a broader scale.
Machine learning technologies promise to resurrect the role played by statisticians by empowering data analysts to model relationships between a multitude of variables in contrast to business intelligence platforms that deliver correlative relationships between two variables. Moreover, machine learning platforms have the capability to model evolution in relationships over time.
While the proliferation of BI platforms ushered in the death of the statistician and enabled the acceleration of the derivation of data-driven insight, it correspondingly facilitated the production of analyses that may have lacked the statistical rigor of analyses that examined correlation through the rich lens of tools at the disposal of trained statistician.
Machine learning tools promise to restore some of that analytical rigor to data-driven analytics by providing additional dimensions of insight to conversations about correlations that may or may not be illustrative of causation. In conversations about health and wellness, in particular, enhanced analytical rigor about correlation vs. causation can go a long way toward ensuring that consumers of data driven analytics make well-informed choices about the best options for their health instead of falling prey to the flotsam and jetsam of findings that are produced by the emergent factory of analytical insights that has been made possible, in part, by the democratization of BI tools.