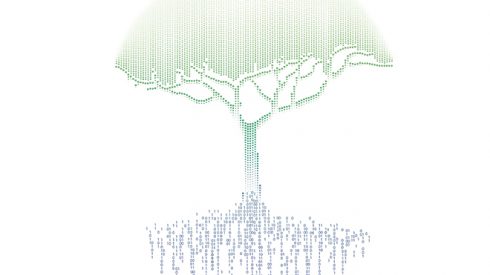
With the rise of big data platforms such as Apache Hadoop and Spark, more and more enterprises are pouring enterprise information into data lakes and launching related initiatives around data quality, data governance, regulatory compliance, and more reliable business intelligence (BI). To prevent the new lakes from turning into swamps, however, businesses are organizing their reams of data via the data’s lineage.
Enterprises have long managed and queried relational data in structured databases and data marts. Emerging environments such as Hadoop, however, often bring together this information with semi-structured data from NoSQL databases, emails and XML documents as well as unstructured information like Microsoft Office files, web pages, videos, audio files, photos, social media messages, and satellite images.
“Even though data is becoming more accessible, users still rely on receiving data from trusted internal sources. For a company, it’s important for users to know and understand the source and veracity of the data. Data lineage tools enable companies to track, audit and provide a visual of data movement from the source to the target, which also ties into the required data governance processes,” said Sue Clark, senior CTO architect at Sungard AS, a customer of Informatica, Teradata, and Qlik.
Through new laws like the General Data Protection Regulation (GDPR) and California Consumer Privacy Act (CCPA) of 2018, government regulators are requiring organizations to perform better management of data originating from all types of raw formats. Enterprises also face increasing demands from business managers for higher quality data for use in predictive analysis and other BI reports.
“Today, companies can’t afford not to make data-driven decisions, which means understanding where data comes from — and how it has changed along the way – to solve business problems,” according to Harald Smith, director of product management, at Syncsort, a specialist in data integration software and services.
“Regulatory compliance demands accuracy, and data lineage tools guarantee a significantly more accurate approach to data management,” echoed Amnon Drori, founder and CEO of Octopai, maker of an automated data lineage and metadata management search engine for BI groups.
Data lineage tools also show up in self-service BI solutions, although apparently, such solutions aren’t yet available to all that many users. In one recent study, TDWI found that only 20 percent of the companies surveyed said their personnel could identify trusted data sources on their own. Further, merely 18 percent responded that personnel could “determine data lineage — that is, who created the data set and where it came from — without close IT support,” according to the report.
“If users and analysts are to work effectively with self-service BI and analytics, they need to be confident that they can locate trusted data and know its lineage. For self-service to prosper, IT and/or the CDO function must help users by stewarding their experiences and pointing them to trusted, well-governed sources for their analysis,” recommended TDWI.
Even fewer of the respondents to TDWI’s survey, or 16 percent, said their end users were able to query sources such as Hadoop clusters and data lakes – but then again, only about one-quarter of the participating organizations even had a data lake.
What are data lineage tools, anyway?
Dozens of proprietary and open-source vendors are converging on data lineage, from a bunch of different directions. Vendors, customers and analysts define data lineage tools in a wide variety of ways, but Gartner has arrived at one short yet highly serviceable definition.
“Data lineage specifies the data’s origins and where it moves over time. It also describes what happens to data as it goes through diverse processes. Data lineage can help to analyze how information is used and to track key bits of information that serve a particular purpose,” according to Gartner’s 2018 Magic Quadrant for Metadata Management Solutions (MMS) report.
Muddying the definitional waters a bit is the fact that enterprises generally use data lineage tools within sweeping organizational initiatives. Accordingly, vendors often integrate these tools with related data management or BI functions, either within their own platforms or with partners’ solutions. Customers also perform their own tool integrations.
Some data lineage tools also transform, or convert, data into other formats, although other vendors perform these conversions through separate ETL (extract, transform, load) tools. Syncsort’s DMX-h, for example, accesses data from the mainframe, RDBMS, or other legacy sources and provides security, management, and end-to-end lineage tracking. It also transforms legacy data sources into Hadoop-compatible formats.
Beyond simply tracking data, for example, organizations need to be able to consume the data lineage information in a way that gives them a better understanding of what it means, said Syncsort’s Smith. Consequently, Syncsort recently teamed up with Cloudera to make its lineage information accessible through Cloudera Navigator, a data governance solution for Hadoop that collects audit logs from across the entire platform and maintains a full history, viewable through a graphical user interface (GUI) dashboard.
For organizations that don’t use Navigator, DMX-h makes the lineage information available through a REST-API, which IT departments can use for integration with other governance solutions.
Some perform impact analysis
Impact analysis capabilities are also offered in some data lineage tools. “With the implementation of GDPR, companies in possession of personal data of EU residents have had to make significant changes to ensure compliance. A large part of this pertains to access — giving people access to their own personal data, enabling portability of the data, changing or deleting the data,” according to Drori.
“Before any company can make a change to its data, it must first locate the data and then of course understand the impact of making a particular change. Data lineage tools are helping BI groups to perform impact analysis ahead of compliance with regulations like GDPR.”
In one real-world scenario, for example, a business analyst needed to erase PII, an age column in a particular report, so that customer age would become private. Data lineage tools helped to solve the problem.
“Before erasing a column the analyst had to understand which processes were involved in creating this particular report and what kind of impact the deletion of this age column would have on other reports. Without data lineage tools, impact analysis can be really tricky and sometimes impossible to perform accurately,” he told SD Times.
Where does data lineage fit?
Experts slice and dice the data management and BI markets into myriad kinds of pieces. In characterizing where data lineage tools fit, major analyst firms such as Gartner and IDC place these tools in the general classification of metadata management.
Gartner’s take. Beyond tools for data lineage and impact analysis, products in the metadata management category can include metadata repositories, or libraries; business glossaries; semantic frameworks; rules management tools; and tools for metadata ingestion and translation, according to Gartner.
Tools in the latter category include techniques and bridges for various data sources such as ETL; BI and reporting tools; modeling tools; DBMS catalogs; ERP and other applications; XML formats; hardware and network log files; PDF and Microsoft Excel/Word documents; business metadata; and custom metadata.
Vendors who made it into Gartner’s 2018 Magic Quadrant for MMS are as follows: Adaptive, Alation, Alex Solutions, ASG Technologies, Collibra, Data Advantage Group, Datum, Global IDs, IBM, Infogix, Informatica, Oracle, SAP, and Smartlogic.
“I don’t have exact adoption rates, but awareness of doing proper metadata management is growing. Initial resistance at the thought it would take away from agility is going away. Organizations can actually add new workloads much faster because the proper discipline is in place,” said Sanjeev Mohan, a research analyst for big data and cloud/SaaS at Gartner, during an interview with SD Times.
Organizations, though, have differing reasons for engaging in data quality initiatives. Before launching an initiative and deciding on an approach to take, an enterprise should first determine the business use case, he advised. “Is it regulatory compliance? Risk reduction? Predictive analysis?”
IDC’s views. Stewart Bond, an IDC analyst, classifies metadata management tools as belonging to a larger category, called data intelligence software. Further, Bond views data intelligence software as a collection of capabilities which can help organizations answer fundamental questions about data. The list of is rather long, but it includes questions about when the data was created, who is currently using the data, where it resides, and why it exists, for example. The answers can inform and guide use cases around data governance, data quality management, and self-service data, he says.
“To collect these answers, organizations must harness the power of metadata that is generated every time data is captured at a source, moves through an organization, is accessed by users, is profiled, cleansed, aggregated, augmented and used for analytics for operational or strategic decision-making. Data intelligence software goes beyond just metadata management, and includes data cataloging, master data definition and control, data profiling and data stewardship,” Bond wrote in a recent blog.
Data intelligence is a subset and different view of Data Integration and Integrity software (DIIS), another market view defined by IDC, according to Bond, who is research director of DIIS at IDC. “Data intelligence contains software for data profiling and stewardship, master data definition and control, data cataloging and data lineage – all which also map into the data quality, metadata management and master data segments in the full DIIS market,” Bond told SD Times in an email.
Examples of vendors included in IDC’s data intelligence and DIIS views are Alation, ASG Technologies, BackOffice Associates, Collibra, Datum, IBM, Infogix, Informatica, Manta, Oracle, SAP, SAS, Syncsort, Tamr, TIBCO, Unifi, and Waterline Data.
However, many products containing data lineage tools are not included in IDC’s data intelligence and DIIS views, or in Gartner’s MMS Magic Quadrant, typically because they don’t meet the specific criteria for those categories and are covered by other areas of analysts’ organizations.
Which data lineage tools are best?
With so many choices available, which data lineage tools will best meet your needs? Factors to consider include whether an initiative is IT- or business-driven, the types of additional data management or BI functionality that will be required, and whether using open-source software is important to the organization, experts say.
Some IT-driven initiatives are concerned with pruning through and curating the organization’s information into data catalogs, so that the most accurate data can then be reused through enterprise applications. Other initiatives are sparked by business managers seeking to quickly put together consistent and reliable data sets for use within corporate departments or company-wide.
For IT-driven initiatives, for example, Informatica provides data lineage through Metadata Manager, a key component of Informatica Power Center Advanced Edition. Metadata Manager gathers technical metadata from sources such as ETL and BI tools, applications, databases, data modeling tools, and mainframes.
Metadata Manager shares a central repository with Informatica’s Business Glossary. The technical metadata can be linked to business metadata created by Business Glossary to add context and meaning to data integration. Metadata Manager also provides a graphical view of the data as it moves through the integration environment.
IT developers can use Metadata Manager to perform impact analysis when changes are made to metadata. Enterprise data architects can use the solution’s integration metadata catalog for purposes such as browsing and searching for metadata definitions, defining new custom models, and maintaining common interface definitions for data sources, warehouses, BI, and other applications used in enterprise data integration initiatives.
In stark contrast, Datawatch targets its Monarch platform at business-driven initiatives. Monarch allows domain experts in business departments to pull metadata for documents in multiple formats – such as Excel spreadsheets, Oracle RDMS, and Salesforce.com, for example — and then use the metadata to build dashboard-driven models for reuse within their departments, said Jon Pilkington, Datawatch’s CPO, in an interview with SD Times.
Monarch’s data lineage tools document “where the raw data came from, how it’s been altered, who did it, when they did it,” for instance. “The model then becomes what users search for and shop,” Pilkington remarked.
Monarch extracts the raw data in rows and columns. After it’s extracted, a domain expert uses Monarch’s point-and-click user interface to convert, clean, blend and enrich data without performing any coding or scripting. It can then be analyzed directly within Monarch or exported to Excel spreadsheets or third-party advanced analytics and visualization tools through the use of built-in connectors.
Within its own marketing department, for example, Datawatch has used its tools to generate reports by salespeople about how information turns into a sales lead and how long it takes to turn a lead into a sale. “We use 11 different data sources for this – including Google Ad Words and the Zendesk support system – and the apps don’t necessarily play well together. It took many steps for the domain expert to get the information into shape, but now that the model is done, it can be reused by any salesperson in the department.”
Three approaches to data management
As Gartner’s Sanjeev Mohan sees it, enterprises can take any of three approaches to data management initiatives: customer-developed, mixing and matching best-of-breed tools, and investing in a broader platform or suite.
By choosing a best-of-breed data lineage tool or metadata management package, a customer can achieve strong support for a specific use-case scenario, the analyst observed. On the other hand, customers often need to perform their own tool integrations, a process that can be expensive and time-consuming.
Sungard AS is one example of an enterprise which is taking a best-of-breed approach. “As part of its internal handling of data and its sources, Sungard AS uses Teradata and Informatica, with Qlik on top of Teradata for ease of business user access and to make data-backed business decisions easier.” Sungard’s Sue Clark told SD Times.
Open source vs. proprietary. Most solutions offering data lineage capabilities are proprietary, said Gartner’s Mohan. Yet some are open source, including offerings from Hortonworks, Cloudera, MapR and the now Google-owned Cask Data, in addition to Teradata’s Kylo.
“We don’t like to lock customers into a specific vendor,” said Shaun Bierweiler, vice president of U.S. Public Sector at Hortonworks, in an interview with SD Times.
Hortonworks is now working with the United States Census Bureau to provide technology for the 2020 census, the first national census to be conducted in a mainly electronic way. HDP will serve as the Census Data Lake, storing most of the census data, while also acting as a staging ground for joining data from other databases. The Census Lake will store both structured data in addition to unstructured data such as street-level and aerial map imagery from Google.