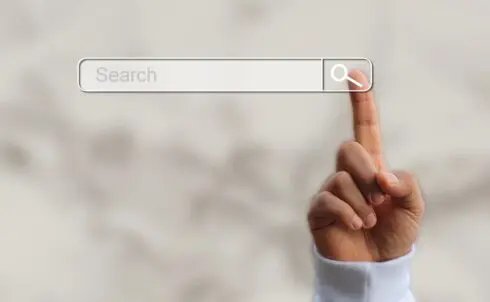
The vector database Qdrant has developed a new vector-based hybrid search capability, BM42, which provides accurate and efficient retrieval for RAG applications.
The name is a reference to BM25, which is a text based search that has been used as the standard in search engines for the last 40 years.
According to Qdrant, the introduction of RAG has made several of BM25’s assumptions no longer relevant. For instance, the typical length of documents and queries is quite different in RAG compared to web search.
“By moving away from keyword-based search to a fully vector-based approach, Qdrant sets a new industry standard,” said Andrey Vasnetsov, CTO & co-founder of Qdrant. “BM42, for short texts which are more prominent in RAG scenarios, provides the efficiency of traditional text search approaches, plus the context of vectors, so is more flexible, precise and efficient.”
BM42 combines the capabilities of text search and vector search to provide better results at lower costs. With BM42, both sparse and dense vectors are used to pinpoint relevant information. The sparse vectors are used for exact term matching, while dense vectors are used for semantic matching.
“Qdrant does not specialize in model training,” Vasnetsov wrote in a blog post. “Our core project is the search engine itself. However, we understand that we are not operating in a vacuum. By introducing BM42, we are stepping up to empower our community with novel tools for experimentation. We truly believe that the sparse vectors method is at exact level of abstraction to yield both powerful and flexible results.”
You may also like…
RAG is the next exciting advancement for LLMs
Elastic launches low-code interface for experimenting with RAG implementation
DataStax releases a number of updates to better facilitate RAG implementation