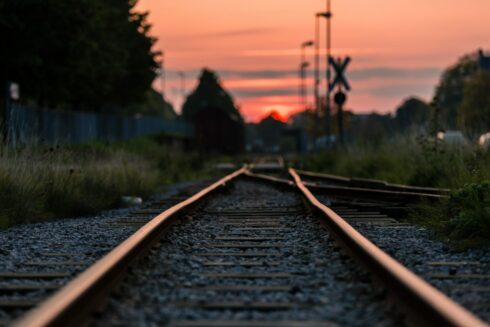
Businesses and developers building generative AI models got some bad news this summer. Twitter, Reddit and other social media networks announced that they would either stop providing access to their data, cap the amount of data that could be scraped or start charging for the privilege. Predictably, the news set the internet on fire, even sparking a sitewide revolt from Reddit users who protested the change. Nevertheless, the tech giants carried on and, over the past several months, have started implementing new data policies that severely restrict data mining on their sites.
Fear not, developers and data scientists. The sky is not falling. Don’t hand over your corporate credit cards just yet. There are other, more relevant ways for organizations to empower their employees with other sources of data and keep their data-driven initiatives from being derailed.
The Big Data Opportunity in Generative AI
The billions of human-to-human interactions that take place on these sites have always been a gold mine for developers who need an enormous dataset in which to train AI models. Without access (or without affordable access), developers would have to find another source of this type of data or risk using incomplete data sets for training their models. Social media sites know what they have and are looking to cash in.
And, honestly, who can blame them? We’ve all heard the quip that data is the new oil, and generative AI’s rise is the most accurate example of that truism I’ve seen in a long time. Companies that control access to large datasets hold the key to creating the next-generation AI engines that will soon radically change the world. There are billions of dollars to be made, and Twitter, Reddit, Meta and other social media sites want their share of the pie. It’s understandable, and they have that right.
So, What Can Organizations Do Now?
Developers and engineers are going to have to adapt their data use and collection in this new environment. This requires new controllable sources of data, as well as new data use policies that can ensure the resiliency of this data. The good news is that most enterprises are already collecting this data. It lives in the thousands of customer interactions that occur inside their organization every day. It’s in the reams of research data that went toward years of development. It’s in the day-to-day interactions between employees and with partners as they go about their business. All the data in your organization can and should be used to train new generative AI models.
While scraping data from across the internet provides a sense of scale that would be impossible for a single organization to achieve, the result of general data scraping is that it produces generic outputs. Look at ChatGPT. Every answer is a mishmash of broad generalities and corporate speak that seems to say a whole lot but doesn’t actually mean anything of significance. It’s eighth-grade level at best, which isn’t what will help most business users or their customers.
On the other hand, proprietary AI models that have been trained on more specific datasets that are relevant to their intended purpose. A tool that’s trained with millions of legal briefs, for example, will produce much more relevant, thoughtful and worthwhile results. These models use language that customers and other stakeholders understand. They operate within the correct context of the situation. And, they produce results while understanding sentiment and intent. When it comes to experience, relevant beats generic every day of the week.
However, businesses can’t just collect all the data across their organization and dump it into a data lake somewhere, never to be touched again. More than 100 zettabytes (yes, that’s zettabytes with a z) were created worldwide in 2022, and that number is expected to continue to explode over the next several years. You’d think that this volume of data would be more than enough to train virtually any generative AI model. However, a recent Salesforce survey revealed that 41% of business leaders cite a lack of understanding of data because it is too complex or not accessible enough. It’s clear that volume is not the issue. Putting the data into the right context, sorting and labeling the relevant information and making sure developers and other priority users have the right access is paramount.
In the past, data storage policies were written by lawyers seeking to limit regulatory and audit risk. Rules governed where and how long data had to be stored. Instead, organizations need to amend their data storage policies to make the right data more accessible and consumable. Data policies need to be modernized – dictating how the data should be used and reused, how long it needs to be kept and how to manage redundant data (copies, for example) that could skew results.
Harnessing Highly Relevant Data that You Already Own
Recent data scraping restrictions don’t have to derail big data and AI initiatives. Instead, organizations should look internally at their own data to train generative AI models that produce more relevant, thoughtful and worthwhile results. This will require getting a better handle on the data they already collect by modernizing existing data storage policies to put information in the right context and make it more consumable for developers and AI models. Data may be the new oil, but businesses don’t have to go beyond their own borders to cash in. The answer is right there in the organization already – that data is just waiting to be thoughtfully managed and fed into new generative AI models to create powerful experiences that inform and delight.