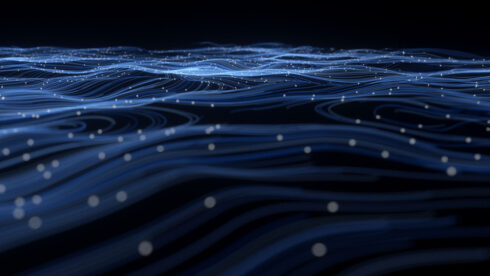
Orca 2 was released by Microsoft to explore the capabilities of smaller language models (LMs) with around 10 billion parameters or less.
The model demonstrates that improved training signals and methods can enhance the reasoning abilities of smaller LMs to make them more on par with larger models.
Compared to similar-sized models, including the original Orca, Orca 2 significantly outperforms them and achieves performance levels similar to or better than models 5-10 times larger, according to Microsoft in a blog post.
It is available in two sizes (7 billion and 13 billion parameters), both fine-tuned on tailored, high-quality synthetic data derived from LLAMA 2 base models. The Orca 2 weights are made publicly accessible to encourage further research on the development, evaluation, and alignment of smaller LMs, Microsoft explained.
The training data was generated to teach Orca 2 various reasoning techniques, such as step-by-step processing, recall then generate, recall-reason-generate, extract-generate, and direct answer methods, while also teaching it to choose different solution strategies for different tasks.
Detailed instructions and multiple calls were used to obtain the teacher model’s responses, allowing the student model to learn underlying strategies and reasoning capabilities in the absence of explicit task instructions. The aim is to optimize performance for smaller models by tailoring solution strategies to the task at hand.
“Orca 2’s success lies in its application of diverse reasoning techniques and the identification of optimal solutions for various tasks. While it has several limitations, including limitations inherited from its base models and common to other language models, Orca 2’s potential for future advancements is evident, especially in improved reasoning, specialization, control, and safety of smaller models. The use of carefully filtered synthetic data for post-training emerges as a key strategy in these improvements,” the Microsoft team wrote in the previously mentioned blog post. “Our findings underscore the value of smaller models in scenarios where efficiency and capability need to be balanced. As larger models continue to excel, our work with Orca 2 marks a significant step in diversifying the applications and deployment options of language models.”