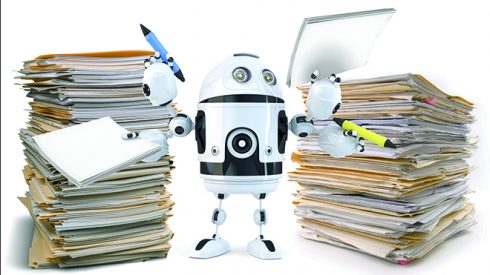
Over the past year, the adoption of robotic process automation — essentially advanced macros or “robotic workers” meant to automate the most mundane, repetitive and time-costly tasks — has seen major growth. As the technology matures alongside machine learning and artificial intelligence, Forrester chief analyst Craig Le Clair said that the most promising future for knowledge workers is one where the ease of setup of RPA and the raw power of machine learning combine to create more efficient, more intelligent robotic workers.
Though Le Clair said that analyst predictions are often “bullish,” growth in the RPA space has greatly outstripped Forrester’s predictions from even a year ago. Forrester has been tracking the space for around three years and has spotted some trends that have contributed to the broader adoption of RPA.
“What we’ve seen is the three top companies are now valued at probably in excess of $10 billion, which is pretty interesting given that most of these platforms were unheard of companies doing $10 million a year in places like Romania, the UK and India,” Le Clair said. “The category has been around for a while, but it was associated with very basic automations that leverage old technologies like screen scraping and so forth. And two things changed to really accelerate it, in my view.”
Through those first three years, Le Clair said the first of these accelerants was the sheer convenience of the RPA model which, unlike other steps that a company might make towards a digital transformation, requires no reconfiguration of core systems or processes.
Joe Blue, director of global data science at data company MapR, shared the conclusion that the pure convenience is key. He described his experience helping customers set up their own RPA processes.
“I think one of the keys for adoption is you don’t want to burden people with a lot of new tools and allow their environments to learn,” Blue said. “In every case, what we try to do is, whatever UI they’re working in, maybe we create a widget, maybe there’s a dashboard that we can add a panel to that that would contain the information that’s needed… Adding to their current UI or adding a step above that routs things to the right person so that they don’t even see 80 percent of the cases that they would have seen because they were automatically delegated and never got there. So it really depends on the process.”
Alongside the success brought by that freedom to place RPA into essentially any workflow and see results, Le Clair said that a new system of central oversight was integral in RPA’s expansion.
“In the early days of the category, bots just ran autonomously on desktops,” Le Clair said. “But the notion of having central management, a central repository where you store the automations for which you could build control tower capability and monitor the activity of the bots and build centralized analytics to dispatch them in an intelligent fashion, that really created more of an enterprise capability. That was sort of the thing that got the category moving and started to experience very high ROI, and an ROI that could be readily understood. You could build an automation, it might cost you $40,000, it might cost you $15,000 a year to maintain a bot, but it was lifting multiple [full time employees] out of an operation. So when you compared it to even the cost of an outsourced capability and a labor arbitrage offshore, the bot was economical.”
But Le Clair contributes the past year’s surprising growth to a very unsurprising factor — the confluence of RPA with AI and ML. Before their integration, RPAs were entirely user-specified to repeat a task as it was performed by the user.
“There’s no learning capability, there’s no AI in the bots that you build,” Le Clair said. “You’re simply mimicking the keystrokes of the human — the mouse clicks, the movements. You’re opening up five or six apps. You’re swiveling between them. The bot’s doing that work that the human is doing. But there’s a perceived limitation in that the set of processes that it was good for was relatively narrow because between programming a lot of decisions in the bots, practicing too many applications and handling exceptions, you had to have very structured, repetitive processes. So a lot of that activity, that could be robotized. But when you start to combine it with machine learning, you start to be able to handle exceptions that aren’t on this highly repetitive, happy path.”
The eventual outcome of this, Le Clair says, is a scenario where every worker has their own robotic assistant to help them out.
“Now, that’s in the future, but those are the kind of messages and direction that’s been driving a lot of value in the category,” Le Clair said.
The present of AI and ML integration in RPA has only scratched the surface, Jason Trent, senior director of product strategy at process automation company K2, said.
“I think if you were to grade this on a maturity scale, at the most mature, organizations are going to start to apply the new learning innovations in things like AI and deep learning to say ‘We’ve gotten and we’ve analyzed all of the work that the bots have done, and we had control groups and we figured out the variances and we’re saving all of this time and money on the bot side,’ ” Trent said.
In the meantime, as AI and ML as part of an RPA stack grows in complexity, both Forrester’s Le Clair and Trent say that companies should learn to treat robotic workers in a similar way to human workers, with proper checks, oversight and managerial accountability, very much in line with Forrester’s assessment of the value of centralized management in RPA.
“The models are inherently built by us,” Trent said. “We haven’t quite gotten to the world where models are building models as effectively. And we see that in all kinds of different allocations of technology, but at the end of the day, we have to use technology to enhance and benefit, not necessarily to take over. In the AI world, they have the big concept called ‘human in the loop,’ and it’s the same thing for RPA. Those robots still need to have managers and you still need to have quality control and checks in place”
Forrester’s Le Clair elaborated on human manager’s role in relation to their robotic workers.
“You want to know who developed the bot and when,” Le Clair said. “You want to understand the bot’s manager. And that manager is accountable for the credentials that the bot has in the same way that a human is responsible to a manager for any nefarious behavior. That’s where the analogy to a human makes a lot of sense, because these are bots that are replacing pieces of what humans are doing. They’re replacing human elements, and they have a lot of the same attended risks that humans have, and they introduce new ones.”
What about the humans?
The professionals responsible for pushing RPA forward aren’t blind to the fact that there are workers being displaced. Right now, Forrester chief analyst Craig Le Clair said, that there are around 26 million employees whose roles lie in that perfect ‘sweet spot’ for RPA.
“One of the things [Forrester has] done is develop a set of 12 generic work personas. And we’ve mapped all of the occupations, the 980 occupations into the 12. And then looked at the progression of automation and the number of automation dividends and deficits and the net result in each category. And it turns out that the most affected personas are what we call ‘cubicle workers,’ and those are the jobs that are the sweet spot for RPA. So those are back-office workers in finance and accounting and HR and in supply chain and in line-of-business operations groups and telecommunications.”
Because many businesses don’t have a good view of the future of work automation, Le Clair says that Forrester developed those 12 personas to make it easier for them to break down which roles will inevitably be trained into RPA models. Over the next 10 years, Le Clair says that it’s around 60 to 70 percent of those 26 million that Forrester expects to find themselves out of a position. But many of them are preparing for it.
“They are being asked to train the bots in some cases, so they kind of see that, “Le Clair said. “So some set of those workers will have what we call constructive ambition and will be able to move on to more human or customer facing, maybe more analytic tasks and roles, but many of them will not and they will at some point, of course, enter the talent economy, enter the gig economy, or end up elsewhere.”
This transition is also already being preempted in the hiring process. While menial tasks are cleared from workers’ schedules, leaving existing employees with more free time, it leaves hiring professionals with something new to think about when looking at resumes. MapR’s Blue said that in many cases, the automation of some tasks has had the effect of increasing specialization across the automation space.
“When I was recruiting to build a team, I was also getting a lot of unsolicited emails from recruiters directly to me and some of those would say MLP, machine learning engineer, deep learning, data scientist, so even within the field of the people creating the RPAs, there seems to be specialization of tasks, so three years ago, it would have just been ‘data scientist,’ ‘commutative scientist,’ but now we’re starting to get into that specialization of ‘yeah, I need a computer imaging data scientist, I need an autonomous car data scientist,’ so we are starting to get to that next level of specialization, even among people that are trying to affect change with RPA.”
And while changes will be felt throughout a number of industries (the medical, retail and financial sectors have been quite receptive to RPA, all sources agreed) as automation progresses, Forrester’s Le Clair reiterated that there’s still quite a ways to go before the bots can be left to their own devices. In “The Forrester Wave: Robotic Process Automation, Q2 2018” report, Le Clair and his analysis team broke down all of the major vendors in the RPA space, and what they were looking for most in a successful RPA product was the proper integration of the RPA with aforementioned human oversight.
“When you build these bots and eliminate the people, you’re also eliminating a lot of process knowledge,” Le Clair said. “So there’s a particular focus on understanding the nuances of the process and documenting that in a way that’s not documented in the coding of the bots. It’s like if you go from making bread from natural ingredients to using a mix and you lose the mix. You have no one that understands the original process.
“What I looked for in the platforms,” Le Clair continued, “was: ‘Did they have mid-cycle auditing?’ ‘Did they have a concept of lifecycle understanding of a bot?’ ‘Did they treat it as a digital worker?’ ‘Did they have the ability in their technology stack to partition the design and the control and the various components so that each department that they had could use the same stack in kind of a multi-tenant approach?’ ‘Did they have a credential vault to manage the credentials securely?’ ‘Did they have an automation center or operating model that allowed the automation to be designed and built in the business, but had enough control centrally and technology management to assure that things would work and work reliably?’ These are things that all of the platforms are striving for. Put the analytics or advanced analytics aside, for the basic automation capability, you need these things. And these providers were in the mode of trying to make that happen, some were further along than others.”