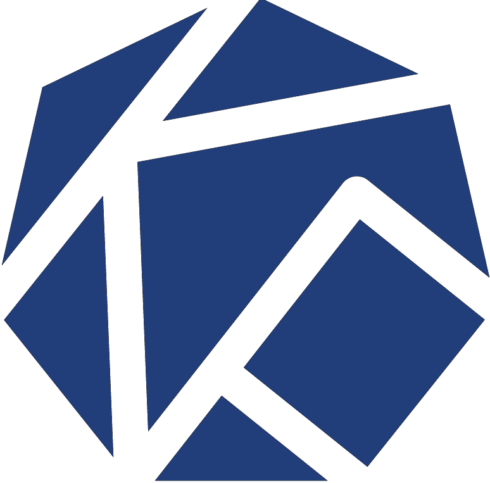
Kubeflow is an MLOps toolkit originally created by Google that has integrated components for model development, model training, multi-step pipelines, AutoML, serving, monitoring, artifact management, and experiment tracking.
The project aims to reduce costs associated with Running production machine learning workflows at scale with new capabilities. The PyTorch training operator can now be scaled up and down introducing elastic training to make use of ephemeral or spot instances and Arrikto added the ability to monitor notebook servers and shut down those that are idle.
The newly released Kubeflow 1.5 includes lower infrastructure costs and helps simplify the operation of the end-to-end machine learning platform. The newest version also includes contributions from Google, Arrikto, IBM, Twitter and Rakuten, and others.
Kubeflow’s UI now also has a more uniform user experience across its components and also simplifies support for high-availability options in its AutoML component.
“Kubeflow is one of the most powerful tools for reducing the complexity of machine learning at scale” said Constantinos Venetsanopoulos, CEO at Arrikto. “As large-scale machine learning projects drive more and deeper value for the world’s largest companies, tools like Kubeflow will be instrumental in helping those companies not get bogged down in complexity and cost that so often hamper those efforts. Arrikto’s mission to help lead the Kubeflow community will continue as we make the development, training and serving of models at scale less complex, more cost efficient and a create a more tightly integrated experience.”
Additional details on Kubeflow are available here.