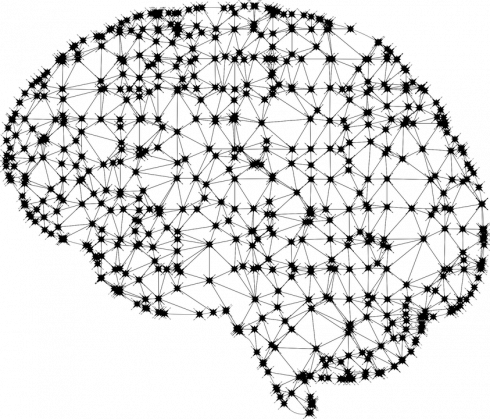
Despite the promises of artificial intelligence, companies are still trying to figure out how to stabilize and scale their AI initiatives. A newly released report revealed while 63.2 percent of businesses are investing between $500,000 and $10 million on AI efforts, 60.6 percent of respondents continue to experience a variety of operational challenges.
According to the report, the top reasons for implementing AI initiatives included efficiency gains, growth initiatives and digital transformation. The top issues data science and machine learning teams faced after implementing an initiative included duplicated work, having to rewrite models after a team member has left, justifying the value of the project, and slow and unpredictable AI projects.
RELATED CONTENT:Most companies prioritize AI, only few have a successful enterprise-wide strategy
The report, State of Development and Operations of AI Applications 2019, was conducted by DevOps and machine learning solution provide Doscience, and based on responses from 500 industry professionals.
“With the amount of resources and money that organizations are spending on their AI initiatives, they cannot afford to make sacrifices when it comes to the productivity and efficiency of the teams responsible for realizing their AI ambitions,” said Luke Marsden founder and CEO at Dotscience. “It is difficult to be productive when different team members cannot reproduce each other’s work. Reproducibility is key to enabling efficient collaboration and auditability. Many companies still rely on manual processes which discourage collaboration and make it difficult to scale and accelerate ML teams.”
The report also found that manual tools and processes still are still predominantly used amongst teams. Teams reported they still use manually updated spreadsheets for metrics and collaborate by working in the same office together. This results in slow and inefficient AI deployments, according to the company.
“When model provenance is tracked manually, AI and ML teams often use spreadsheets without an effective way to record how their models were created. This is inflexible, risky, slow and complicated. To simplify, accelerate and control every stage of the AI model lifecycle, the same DevOps-like principles of collaboration, fast feedback and continuous delivery should be applied to AI,” Marsden said.
In an attempt to get rid of these challenges of operationalizing AI in the enterprise, Dotscience emerged from stealth this week with a new platform that aims to provide a collaborative end-to-end machine learning data and model management solution. According to the company, the platform will enable teams to collaboratively track runs to gain a record of the data, code and parameters used when training an AI model, as well as collaborate on, develop, test, monitor and deliver their ML projects. Features include integration with continuous integration and monitoring tools, ability to work with data from any source, and the ability to use familiar tools such as PyTorch, Keras and TensorFlow.
“The current state of AI development is a lot like software development in the 1990s. Before the movement called DevOps, modern best practices such as version control, continuous integration and continuous delivery were far less common and it was normal that software took six months to ship. Now software ships in minutes,” said Marsden. “At Dotscience, we are applying the same principles of collaboration, control and continuous delivery of DevOps to AI in order to simplify, accelerate and control AI development.”