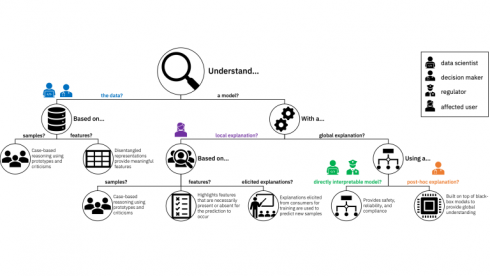
IBM is releasing a new open-source project designed to help users understand how machine learning models make predictions as well as advance the responsibility and trustworthiness of AI. IBM’s AI Explainability 360 project is an open-source toolkit of algorithms that support the interoperability and explainability of machine learning models.
According to the company, machine learning models are not often easily understood by people who interact with them, which is why the project aims to provide users with insight into a machine’s decision-making process.
RELATED CONTENT: AI ethics: Early but formative days
The toolkit offers IBM explainability algorithms, demos, tutorials, guides and other resources to explain machine learning outcomes. IBM explained there are many ways to go about understanding the decisions made by algorithms.
“It is precisely to tackle this diversity of explanations that we’ve created AI Explainability 360 with algorithms for case-based reasoning, directly interpretable rules, post hoc local explanations, post hoc global explanations, and more,” Aleksandra Mojsilovic, IBM Fellow at IBM Research wrote in a post.
The company believes this work can benefit doctors who are comparing various cases to see whether they are similar, or an application whose loan was denied can use the research to see the main reason for rejection.
“This extensible open source toolkit can help you comprehend how machine learning models predict labels by various means throughout the AI application lifecycle. Containing eight state-of-the-art algorithms for interpretable machine learning as well as metrics for explainability, it is designed to translate algorithmic research from the lab into the actual practice of domains as wide-ranging as finance, human capital management, healthcare, and education,” according to the project’s website.
The tool is meant to complement Watson OpenScale, which helps clients manage AI transparently throughout the full AI life cycle, regardless of where the applications were built or where they run on.
Algorithms include Boolean Decision Rules via Column Generation, Generalized Linear Rule Models, ProfWeight, Teaching AI Explain its Decisions, Contrastive Explanations Method, Contrastive Explanations Method with Monotonic Attribute Functions, Disentangled Inferred Prior VAE, and ProtoDash.