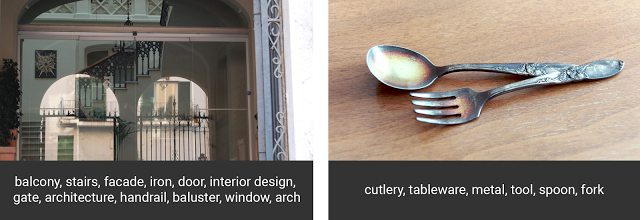
Recent advances in machine learning have changed the way computers understand digital images and video. In order to further advance natural language and machine learning, Google introduced Open Images, a dataset consisting 9 million URLs to images that have been annotated with labels that cover more than 6,000 categories.
According to Google software engineers Tom Duerig and Ivan Krasin, the company tried to make this dataset “as practical as possible.” This means the labels cover more real-life entities than the 1,000 covered by ImageNet classes, and there are enough images for researchers to train a deep neural network from scratch.
(Related: How machine learning became the new SOA)
The image-level annotations are populated automatically with a vision model, similar to the Google Cloud Vision API, according to the blog. Human raters verified automated labels to find and remove false positives, and on average, each annotated image has about eight labels assigned.
For instance, an image of a fork and spoon has the following labels: cutlery, tableware, metal, tool, spoon, and fork, whereas a picture of a well-designed building would have labels like balcony, stairs, iron, door, interior design, and architecture.
Google engineers hope to improve the quality of these annotations in Open Images so that the models can be better trained. So far, Google has trained an Inception v3 network architecture based on Open Images annotations alone, and “this model is good enough to be used for fine-tuning applications” or for other things like DeepDream, said Duerig and Krasin.
These annotations are licensed by Google under the CC BY 4.0 license. Also, the images are listed as having a CC BY 2.0 license. Google tried to identify images that are licensed under a Creative Commons Attribution license, but according to its GitHub page, Google makes no representations or warranties regarding the license status of each image, and developers or researchers should verify the license for each image themselves.
The dataset is the result of collaboration from Cornell, Carnegie Mellon University and Google, and there are a research papers built on top of the Open Images dataset in the works.