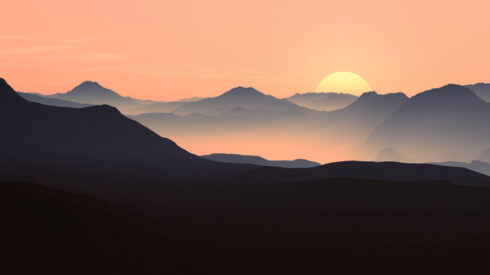
The growing mountains of data generated by organizations is literally staggering, so ensuring that data is of good quality is a huge challenge. As the SD Times Data Quality Project 2021 has revealed, one area in particular that can give companies fits is product information.
In the case of the pharmaceutical industry, but likely true in many other industries, companies want to know who is ordering the product, who is using it and for how long. But things can go wrong that affect the data these companies rely on.
James Royster is the head of analytics at Adamas Pharmaceutical and formerly the senior director of analytics and data strategy for biopharmaceutical company Celgene. He explained the difficulty in keeping track of product use in an industry that offers hundreds of thousands of products sold at some 60,000 retail outlets nationwide as well as being dispensed at health care facilities and doctor’s offices across the United States.
Companies compile huge datasets from all those transactions, and have developers write code that brings the datasets together in a way that can be digested by the companies to use to make better business decisions. But, as Royster pointed out, “as they’re changing code, updating code, collecting data, whatever it is, there’s millions of opportunities for things to go wrong.” A programmer mistyping a product code into the dataset can result in literally millions of transactions not being recorded properly, and when the organization sees a big dropoff in sales of that product, it has to launch a time-consuming effort to find out what happened.
In the case of medicines, when a prescription is written and a patient picks it up from the pharmacy, that is recorded. Then there are scenarios in which a prescription is written by a doctor and filled at a pharmacy, but the patient decides he doesn’t want to pick it up. Royster that said is known as a ‘restatement,’ when the pharmacy puts the drug back on the self and reports that, that is normal and can be traced.
But problems can arise when, for instance, when a pharmacist enters the wrong code, or a developer working in SQL changes something in the data that has a downstream effect that the company isn’t aware of. “Let’s say that a company like IQVIA, which used to be IMS, is aggregating a bunch of data from pharmacies, and all of a sudden somebody does something and two pharmacies are no longer in that data. We don’t see it at that level. But we do see that there’s a historical shift in the volume. Now, that is not something that’s supposed to happen. So if you’re a good company, you would detect that and say, why did that shift happen, and then we can go back and trace it and find out that somebody made a mistake in some sort of coding that knocked one or two of the pharmacies out of the data set. So the data set itself was not correct. And there are thousands of nuance things with these data sets that can go wrong, exactly like I’m describing. Some of them are legitimate and are supposed to happen. And that data is supposed to change historically, and for the future. And some of it is the artifact of something that somebody did that made something happen that wasn’t supposed to happen.”