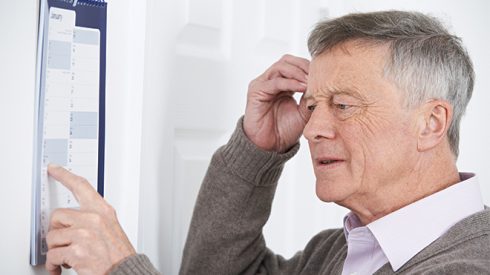
Late September, IBM and the University of California San Diego announced their partnership with the opening of the Artificial Intelligence for Healthy Living Center on UCSD’s campus, the latest piece of IBM’s Cognitive Horizons Network, a research collective focused on the emerging fields of Internet of Things, artificial intelligence and machine learning.
UCSD’s team of researchers are tasked with tackling what Susann Keohane, founder of IBM’s Aging-in-Place Research Lab, wrote “will have unprecedented effects on health care, the economy, and individual quality of life,” — society’s demographic shift towards an older population.
“For the first time ever, there are more people over 65 than under 5,” Keohane said. “There is essentially a shortage of care providers. If you look at this demographic shift across the globe, this is why a company like IBM is interested in looking at aging. What does the aging demographic shift mean for our clients? We’re in every industry and every one will be impacted.”
By combining IBM’s IoT and health care research with their Watson machine learning, Keohane says that the effect this shift will have can be broken down in such a way that it will benefit everyone from the elderly in need of improved care to businesses, now more aware of their customer base.
“Aging isn’t a disease,” Keohane said. “We’re all doing it. But it does have impact on health. So could we surround ourselves with emerging technology in the home, while assuring the privacy and security that comes with health care and design something that will help someone understand how well they’re aging in place?”
The project’s Cognitive Infrastructure team, led by professor Tajana Šimunić Rosing, one of the directors of UCSD’s AI for Healthy Living Center, has already taken a stab at answering this question. By tracking things like bathing and toileting with unobtrusive, IoT-connected sensors installed voluntarily in some elder-care facilities like Paradise Village in National City, California, with more test beds being set up over the course of the next year, Keohane and Rosing say that some trends are already coming to light.
“We are starting to see consistent patterns in behaviors,” Keohane said. “So what that tells us is that, one, because the sensors are in the right place, our algorithms are working really well. For example, if I was to look at a month of data and I was trying to detect bathing with a consumer-grade sensor, I, with really good accuracy, can tell you that with 31 days in a month, that sensor goes off at 8:30 [a.m.] every day but two days. The consistency and the accuracy is really exciting, because if it was all over the place, then maybe the person wasn’t doing so well, maybe they weren’t taking care of themselves.”
Rosing described the example of a 90-year-old grandfather who’d begun forgetting to turn off the stove after cooking though he was otherwise fairly capable. “The goal is to try to understand how people’s habits may change as they get a little bit older,” she said. By combining a sensor with robotics and AI, the latter two of which are handled by other branches of the research team, their efforts would serve to “detect and then provide intervention and turn off the stove when we know that the person isn’t standing in front of it or when there’s no pot on it and it’s still burning, for example.”
Keohane provided further examples, such as tracking bathroom and shower usage to reasonably infer risk of urinary tract infection, a common cause of death for those over 70, and tracking motion for nighttime wandering, an early sign of dementia.
Rosing says that it’s these types of easy-to-track detections — running water or an alert that a subject has missed a doctor’s appointment — that are the backbone of the research. Keohane refers to these small but significant data points as “little ‘heartbeats, just on-and-off signals.” But by corroborating data from all of the individual sensors and finding the patterns, there are many observations to be made.
“This is important because it tells us about the state of people’s cognitive capability,” Rosing said. “The more of this type of forgetfulness you have, the more it correlates with issues you may have during a daily-living situation. This could be indicative of needing more support or needing some intervention.”
While collecting accurate readings seems to be a cinch for IBM’s IoT sensors, the next step is feeding that data to IBM’s Watson computer for AI. That’s where Keohane says the true challenge of their research lies.
“One of the core technologies we have is the Contextual Data Fusion Engine, which allows us to pull together disparate, siloed data sets, normalize them and look for the patterns across them,” Keohane said. “The challenge is really having good data and data that’s labeled. If you start to train your algorithms on data, you want to make sure it’s good, otherwise your predictive model is going to be completely off.”
Keohane says that UCSD’s research will help improve the reliability of the data with a team of dedicated ethnographers that will annotate the collected readings and look for patterns themselves, before they start to generate a predictive model for AI.
Rosing says that one of the most exciting parts of the project for her is the way that the team is utilizing tried-and-true ethnography techniques on a much broader scale, looking for more specific behaviors over a much longer period and across populations.
“Studies look at coarse behavior usually — when do you wake up, when do you shower, eat and so on,” Rosing said. “What we’re looking at in this case is much finer — are you having longer pauses in your speech now, are your body movements a little bit rougher or a little bit more clumsy? These microbehavioral changes are very highly correlated with the kind of cognitive changes we’re seeking to detect.”
By studying how these “microbehaviors” correlate with data gathered from the sensors installed by Rosing’s team, the ethnographers will be able to begin designing the predictive model that will eventually guide the initiative’s efforts.
The ethnographers are also working on ways to create customized monitoring methods for individuals that can then be extrapolated. Rosing described how she might test the cognition of her grandfather, an avid gardener, by asking him to list off what should be planted around March.
“Right now he can rattle off plenty of things you should be planting in March,” Rosing said. “Maybe he doesn’t remember all of them; the fraction that he forgets is a metric that tells us that maybe his cognitive abilities need a little support.” Rosing says this would not be useful, for instance, in testing the cognition of her mother-in-law, who doesn’t care much for gardening, but might respond better to questions about her beloved dog. Knowing how to apply specialized tests like these on a broader scale is something that Rosing says the ethnographers will continue to work on.
Past that, Keohane thinks the final hurdle for assistive technologies like the ones her team is researching is adoption of the technology.
“How do we help people embrace and feel good about embracing it?” said Keohane. “Privacy and security are very important to [IBM]. So we have the right infrastructure in place, and I think that creates a lot of potential for what a company like IBM can do in this space.”