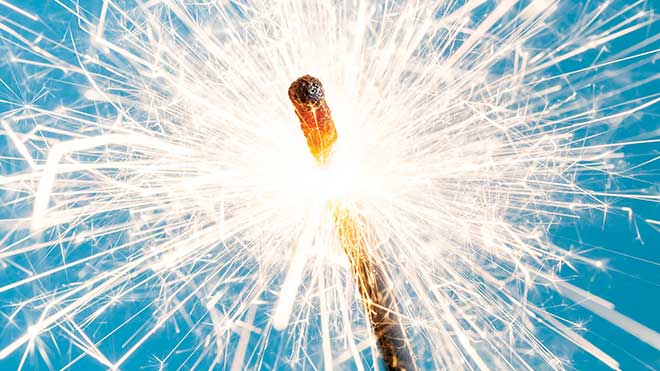
The Apache Hadoop project took off in enterprises over a fairly short period of time. Four or five years ago, Hadoop was just becoming a “thing” for enterprise data processing and experimentation. MapReduce was at the heart of that thing, and Spark was still only a research project at the University of California at Berkeley. Soon after, though, if you were doing “Big Data,” you were using Hadoop.
Spark wasn’t even an Apache project when Cloudera, Hortonworks and MapR were already in full business swing in 2013 with Hadoop offerings. Only two years ago did it graduate to be a top-level project.
Today, Spark is a part of most Big Data conversations, as is evidenced by how many vendors are offering integrations, or are planning them in the near future. Large enterprises, such as Toyota, Palantir, Netflix and Goldman Sachs, are embracing the technology.
(Related: A detailed look at Spark 1.6)
Is this uptake at the expense of Hadoop? That’s a larger question, but to begin with, it’s become clear that Spark is replacing MapReduce. Anand Venugopal, head of product for StreamAnalytix at Impetus Technologies, said he believes this is the case.
“The MapReduce computing paradigm is likely going to get replaced by Spark as the distributed compute model overall for any workload,” he said. “There’s one metric I use [when deciding what to support], which is, what is the number of customers that tell us ‘We don’t want to talk until you have Spark?’ That same metric is used for any technology: Is there a critical mass of customers who have a seriously broad decision-making body in the enterprise customer that has committed itself to a particular enterprise technology?”
He went on to state that this critical mass currently exists in Spark, and that his company’s streaming analytics platform is bringing support online in the first quarter of 2016.
Ajay Anand, vice president of products for Kyvos Insights, said, “Most customers expect to see Spark support in the road map, and we are definitely embracing it along with Hadoop. From my perspective, we look at what is the problem we’re looking to solve, and what is the right technology that is mature enough to help us solve that problem.”
Kyvos Insights has built an interactive analytics solution on top of Hadoop, and Anand said that his team looked for a way to “do fast incremental analytics. There’s capabilities in Spark to do those interactive tasks, and there’s a natural advantage for using Spark’s in-memory computation that can help us in our solutions.”
Two great tastes
The question of whether or not Spark is replacing Hadoop is largely focused on the wrong question. The question shouldn’t be about replacing Hadoop, but rather what portions of Hadoop are being replaced by Spark. At present, MapReduce is the victim of users quickly moving to Spark, but the underlying data storage layer of Hadoop (HDFS and HBase) is likely not going away any time soon.
Thus, Forrester principal analyst for application development and delivery Mike Gualtieri feels that Hadoop and Spark will remain tied together for some time to come.
“I think Spark and Hadoop make the most sense together. You get the best of both worlds. Hadoop was designed for large volumes, Spark was designed for speed. When the data will fit in memory, use Spark, but if you want long term storage you use Hadoop,” said Gualtieri.
Ion Stoica, CEO and cofounder of Spark company Databricks, feels that Spark can completely replace Hadoop when combined with the right data store. That’s because Spark can be run against more than simply HDFSes.
“We are working well with Hadoop,” he said. “Spark is a data-processing engine, so if people already have their implementation of a data lake or data hub using Hadoop and HDFS, Spark will happily consume that data. However, if we look forward, we do believe we will see more and more instances where Spark will consume data from other data sources. If you’re in the cloud storing data in Amazon S3 or in Microsoft Azure’s Block Store, there is not a great reason to just spin up a Hadoop cluster in Amazon.”
Stoica went on to say that usage of Spark against existing enterprise storage systems is growing. “The other thing we’re seeing is if you think about many of the different enterprises, they have a storage solution—be it a database or a simple highly reliable data store—and that company wants to provide an analytics solution, until now the default solution was to also sell the first solution for the Hadoop cluster for analytics,” he said.
That’s a big win for companies like EMC, Teradata and NetApp, which have been scrambling to re-acclimate in our new Hadoop world, where storage of enterprise data is effectively commoditized.
“Going forward, many of these companies are going to align with Spark, first because it’s a good processing engine, and second is because Spark doesn’t provide a storage engine, it is not competing with storage providers,” said Stoica. “If I am going to be a storage provider and sell a packaged Hadoop cluster, it’ll provide very cheap storage, which will compete with my own solutions.
“DataStax offers Apache Cassandra. They used to package it with Hadoop, but now they are packaging only with Spark. SAP HANA is packaging with Spark. I think you are going to see more and more of these storage providers bypassing Hadoop and using [it] when it comes to analytics.”
But Gualtieri thinks there’s a specific class of business that will choose to forego Hadoop and bang straight for Spark. “I think who’s going to say that is a startup with a lot of venture money, and really if you think about it, the relationship between the two is that Spark has no file system, but someone can say ‘I’m going to use just UNIX or an EMC SAN,’ ” he said.
That’s because, at the end of the day, HDFS is still the cheapest way to put petabytes on disk, and even without the rest of Hadoop, many enterprises have already begun the migration to an HDFS data lake, the momentum of which has an effect on future architecture of a company’s data as a whole.
One company that has ditched HDFS in favor of its own storage medium is IBM. “IBM has really made some investments and moves, not the least of which is Spark running on the Z Series mainframe, which is just amazing,” said Gualtieri. “That’s Spark without Hadoop, and that’s very interesting because that’s where many companies’ transactions are. Now you can do your analytics on the database.”
And mainframes are still where it’s at for many enterprises. While Hadoop has grown dramatically inside many organizations over the past five years, it’s still early days, says Gualtieri.
“The main [enterprise] questions are still about Hadoop. From an enterprise standpoint, they still want to adopt Hadoop. They see that as the first step, but at the same time they understand Spark is part of what I call ‘Hadoop and Friends.’ All the major distributions include Spark now. The cloud providers provide it as well,” said Gualtieri.
Multi-talented
Perhaps one of the reasons for Spark’s quick rise to prominence among a field of Apache projects for Big Data on Hadoop is the fact that Spark has more capabilities. While it’s clearly an easier way to write processing jobs for a Hadoop cluster, Spark also includes Spark SQL and Spark Streaming.
Spark Streaming is part of a burgeoning movement toward more stable and open-source stream-processing solutions, but it is likely that the hardcore real-time users will stick with Apache Storm or move to Apache Flink; Spark Streaming typically has about a second or so of latency involved.
StreamAnalytix’s Venugopal said, “There are other wonderful advantages of Spark Streaming, like the simplicity of machine learning. But it is not the solution to many problems that other solutions exist for. Low-latency stream processing, such as anything under 500ms, is not a candidate for Spark Streaming. We see enterprises using Storm and Kafka for their streaming stack.
“We see many large enterprises still…adopting things like Apache Storm for low-latency streaming use cases, so we have taken the approach of abstracting both Spark Streaming and Storm under a single UI layer so enterprises have the freedom of choosing for the right use case.”
Spark SQL, on the other hand, is keeping up very well with the competition from Cloudera: Apache Impala. According to benchmarks performed by AtScale, Spark SQL and Impala each have their advantages and performance benefits. He also pointed out that they’re on the interactive query end of the scale, while Hive on Tez are on the batch-processing end of the spectrum.
AtScale’s CEO Dave Mariani said that his company’s benchmarks showed that “Essentially there’s not one SQL-on-Hadoop engine that does it all. There are different workloads: There’s batch and interactive. Batch means we’re computing in aggregate on the cluster. Where you find Big Data with the trillions of rows, tools like Hive on Tez tend to be more stable and be able to consistently produce those results.
“For interactive queries, Spark SQL and Impala are really good at accessing smaller data sets very quickly. Hive on Tez is the tortoise that wins the race. You can’t run a Hive on Tez query and get an answer faster than 10 seconds, versus Impala and Spark SQL, where you can get an answers in milliseconds.”
Because of this, Mariani said he sees customers mixing SQL engines: They’ll process the massive cluster data with Hive on Tez, then use Impala or Spark SQL to run interactive queries on the data aggregated by Hive on Tez.
“You need to have more than one engine depending on the workload. There is a reason for these multiple engines to exist,” said Mariani.
While he said that Impala outperformed Spark SQL when running many queries concurrently, he also said that Spark 1.6 has made major improvements that make life with SQL much easier for developers and data scientists alike.
“There’s been a dramatic improvement in Spark SQL’s ability to not just perform quickly, but also to not fail on large data sets. 1.6 is significantly improved on both fronts. They basically rewrote the internals of how they do query processing, and they improved the joins and join functionality dramatically,” he said.
It’s just easier
At its core, Spark is about making large-scale data processing jobs easier in every direction. These benefits are evident when compared to MapReduce. In fact, Spark is quickly replacing MapReduce simply because it puts the power of the Hadoop cluster directly into the hands of the data scientist, without the need for a Java developer in between.
Thilina Gunarathne, director of data science and engineering at KPMG, does a lot of work processing large data sets for big enterprises, and he’s got more than six years of Hadoop experience under his belt to help with that.
“When we do these solutions, [enterprises] don’t care much about what’s underneath, but they care about the data science layer and the analytics layer,” he said. “Spark is a home run for data scientists and data analysts.”
Gunarathne said his teams build both internal Hadoop queries and systems as well as help with external consulting. He said that in both cases, the data scientists are the ones driving the demand for Spark.
“Right now it’s mostly Spark SQL, but there are people who want to query Hive tables and do their things with [the Spark Machine Learning library] and use the Python bindings,” he said. “Traditional companies that have been using Hadoop for a while, they’re still a little bit behind in terms of adoption of Spark.”
He went on to say that “Most of the time, what ends up happening is people write a lot less code because of the APIs and the available libraries. The guys familiar with data science will be reluctant to do anything non-Hadoop, but they didn’t really write MapReduce code. Their use case was to pull out data then run models against it.
“That process is gone with Spark now, given the APIs are much easier, and with Python bindings it’s much easier to use,” said Gunarathne. “In terms of the data science side of the things, it’s much improved and easier. But if you look at traditional data engineering side, people who used to write MapReduce code, even that is much easier. Using Resilient Distributed Datasets and DataFrames is much easier.”
The future
Spark’s future may be bright, but as a fast-moving open-source project, some enterprises may be frightened away by the speed with which it is evolving.
Gunarathne said that this rapid development is actually a deterrent for some enterprises. “Spark has a lot of traction. They quoted that they…are the most active project at Apache, but from a production point of view, that’s not a plus. That means the codebase is still fast-moving. For the conservative people who want stability, they’re sticking with Hadoop unless they have a time-sensitive pipeline.”
But Databricks’ Stoica said there are a great many improvements to the platform in their pipeline and in the community’s. “It’s a very fast-growing system, and there are growing pains. I don’t think there is any fundamental challenge, but there are growing pains. We want to push availability, we want to push on performance. As we started growing, we’ve added more and more security features. This has been an obvious direction. We are going to push these to the extreme,” he said.
One of the other major focuses for the future of Spark will be Project Tungsten: the effort to bring Spark closer to bare metal and to drastically improve CPU and memory performance. Recently, these have been the bottlenecks for Spark jobs processing on clusters, and Tungsten seeks to solve them.
“Tungsten will address a great deal of things on performance in terms of scale,” said Stoica. “Spark is Scala, and Scala is running in a JVM. When you read data, you deserialize data and you have these Java objects. It’s very memory-inefficient when you read some more complex data structure and deserialize it. Memory can grow several times in size. Also having small objects doesn’t help with garbage collection in Java.
“With Tungsten, because we have the data and we know the schema, we can keep it in binary format in-memory. We can access that directly because it knows the schema, and this means much less memory usage and better scale. We don’t need to flood the JVM with a lot of small objects, which means much lower overhead for garbage collection.”
So while Spark has a lot of growing ahead, it’s already making life easier for data scientists everywhere. Here’s hoping future improvements bring those benefits to the entire development team.
What’s New in Spark 1.6
Parquet: For the release of version 1.6, the Apache Spark community and Databricks focused largely on performance improvements that could be implemented across Spark.
One such speedup comes from the increased performance for processing data in the Apache Parquet format. This will accelerate the performance of Apache Spark when working with Hadoop systems.
Apache Parquet is a columnar storage format that works with any of the Hadoop projects. The 1.6 release of Apache Spark introduces a new Parquet reader, bypassing the existing parquet-mr record assembly routines, which had previously been eating up a lot of processing cycles. The change promises an almost 50% improvement in speed.
Memory: Apache Spark 1.6 also includes better memory management. Previously, when commencing processing, Spark would divide available memory in two and work with it as such. Now, the memory manager in Spark can automatically tune the size of different memory regions. The runtime will now grow and shrink regions according to their specific needs.
The state management API in Spark Streaming has been redesigned in this release. Version 1.6 is the first to include the new mapWithState API, which scales linearly with the number of updates, rather than the total number of records. This allows it to track the deltas rather than constantly rescanning the full dataset.
Type-safe DataFrames: Speaking of datasets, version 1.6 includes the new DataSet API, which allows for compile-time type safety within DataFrames. The existing DataFrames API, using the DataSet API, now supports static typing and user functions that run directly on Scala or Java types.
For data scientists, Spark 1.6 has improved its machine-learning pipeline. The Pipeline API offers functionality to save and reload pipelines in persistent storage. Spark 1.6 also increases algorithm coverage in machine learning; this adds support for univariate and bivariate statistics, bisecting k-means clustering, online hypothesis testing, survival analysis, and non-standard JSON data.