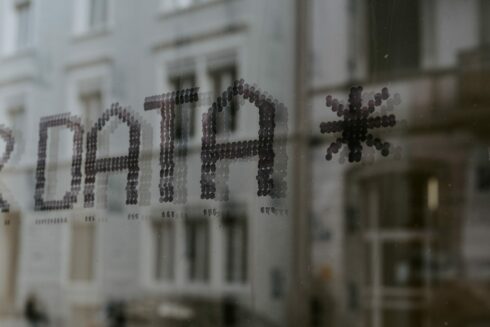
As we venture deeper into the realm of machine learning and Generative AI (GenAI), the emphasis on data quality becomes paramount. John Jeske, CTO for the Advanced Technology Innovation Group at KMS Technology, delves into data governance methodologies such as data lineage tracing and federated learning to ensure top-tier model performance.
“Data quality is the linchpin for model sustainability and stakeholder trust. In the modeling process, data quality makes long-term maintenance easier and it puts you in a position of building user confidence and confidence in the stakeholder community. The impact of ‘garbage in, garbage out’ is exacerbated in complex models, including large-scale language and generative algorithms,” says Jeske.
The Problem of GenAI Bias and Data Representativeness
Bad data quality inevitably culminates in skewed GenAI models, regardless of the model you choose for your use case. The pitfalls often arise from training data that misrepresents the organization’s scope, client base, or application spectrum.
“The real asset is the data itself, not ephemeral models or modeling architectures. With numerous modeling frameworks emerging in recent months, data’s consistent value as a monetizable asset becomes glaringly evident,” Jeske explains.
Jeff Scott, SVP, Software Services at KMS Technology, adds, “When AI-generated content deviates from expected outputs, it’s not a fault in the algorithm. Instead, it’s a reflection of inadequate or skewed training data.”
Rigorous Governance for Data Integrity
Best practices in data governance encompasses activities such as metadata management, data curation, and the deployment of automated quality checks. Examples include ensuring the origin of data, using certified datasets when acquiring data for training and modeling, and considering automated data quality tools. Though adding a layer of complexity, these tools are instrumental for achieving data integrity.
“To enhance data quality, we use tools that offer attributes like data validity, completeness checks, and temporal coherence. This facilitates reliable, consistent data, which is indispensable for robust AI models,” notes Jeske.
Accountability and Continuous Improvement in AI Development
Data is everyone’s problem and assigning responsibilities for data governance within the organization is a fundamental task.
It is paramount to ensure the functionality works as designed and that the data being trained is reasonable from a potential customer standpoint. Feedback reinforces learning, and is then accounted for the next time the model is trained, invoking continuous improvement until the point of trust.
“In our workflows, AI and ML models undergo rigorous internal testing before a public rollout. Our data engineering teams continuously receive feedback, allowing iterative refinement of the models to minimize bias and other anomalies,” states Scott.
Risk Management and Customer Trust
Data governance requires data stewardship from relevant areas of the business with subject matter experts continuously involved. This ensures responsibility that the data that flows through their teams and systems is appropriately groomed and consistent.
The risk associated with receiving inaccurate results from technology must be understood. An organization must assess its transparency from data sourcing and handling IP to overall data quality and integrity.
“Transparency is integral for customer trust. Data governance isn’t solely a technical endeavor; it also impacts a company’s reputation due to the risk transference from inaccurate AI predictions to the end-user,” Scott emphasizes.
In conclusion, as GenAI continues to evolve, mastering data governance becomes more critical. It’s not just about maintaining data quality, but also about understanding the intricate relationships that this data has with the AI models that leverage it. This insight is vital for technological advancement, the health of the business, and to maintain the trust of both stakeholders and the broader public.
Find out more in our podcast episode with John Jeske that goes more in-depth.