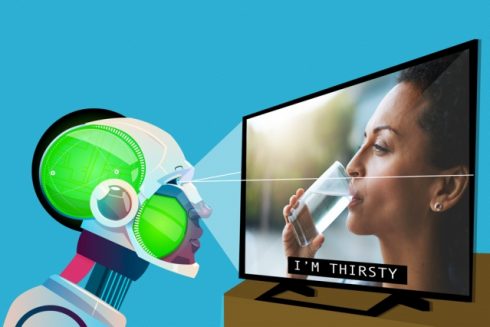
Children learn language by observing the things around them and connecting the dots between what they are seeing and hearing. By learning language like this, they are able to establish a language’s word order, such as where subjects and verbs belong in a sentence.
In machine learning, languages are learned by training systems on sentences annotated by humans that describe the structure and meaning of words. Gathering that annotation data can be time-consuming and practically impossible for less common languages. Furthermore, not all humans agree on annotations, and annotations might not accurately reflect how people naturally speak.
This week, MIT researchers presented a paper describing a new parser that learns the way a child does. The parser observes captioned videos and associates words with recorded objects and actions.
When provided a new sentence, the parser can then use what it learned about the language structure to predict a sentence’s meaning without the accompanying video.
This approach is “weakly supervised,” meaning that it requires limited training data. According to the researchers, the approach could expand data types and reduce the effort required for training parsers.
The researchers also believe that this parser could be used to make interactions between humans and personal robots more natural. “People talk to each other in partial sentences, run-on thoughts, and jumbled language. You want a robot in your home that will adapt to their particular way of speaking… and still figure out what they mean,” said Andrei Barbu, co-author of the paper and a researcher in the Computer Science and Artificial Intelligence Laboratory (CSAIL) and the Center for Brains, Minds, and Machines (CBMM) within MIT’s McGovern Institute.
In the future, the researchers plan to look into modeling interactions as opposed to just passive observations, the researchers explained.