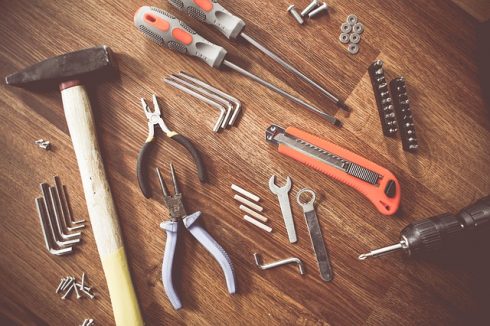
Testing has always required tools to be effective. However, the tools continue to evolve with many of them becoming faster, more intelligent and easier to use. Continuous testing (CT) necessarily recognizes the importance of testing throughout the software delivery life cycle. However, given the rapid pace of CT, tests need to run in parallel with the help of automation. However, that does not mean that all tests must be automated.
RELATED CONTENT: Who owns continuous testing
Continuous testing at every step
Why continuous testing is so important
The nature of “tools” is evolving in the modern contexts of data analytics, AI and machine learning. Nearly all types of tools, testing or otherwise, include analytics now. However, tool-specific analytics only provide a narrow form of value when used in a siloed fashion. CT is part of an end-to-end continuous process and so success metrics need to be viewed in that context as well.
AI and machine learning are the latest wave of tool capabilities which some vendors overstate. Those capabilities are seen as enablers of predictive defect prevention, better code coverage and test coverage, more effective testing strategies and more effective testing strategy execution. It takes some basic knowledge of AI and machine learning to separate tools that actually include those features versus other tools that only sound as if they provide those features.
CT-related tools
Diego Lo Giudice, VP and principal analyst at www.forrester.com Forrester Research outlined some of the testing tools needed for a CT effort have been included below. The list is merely representative as opposed to exhaustive:
- Planning – JIRA
- Version Control – GitHub
- CI – Jenkins
- Unit testing – JUnit, Microsoft unit test framework, NUnit, Parasoft C/C++test,
- Functional testing –Micro Focus UFT, TestCraft
- API testing –Parasoft SOAtest, SoapUI
- UI testing – Applitools, Ranorex Studio
- Test suites – Smart Bear Zephyr, Telerik Test Studio
- Automated testing (including automated test suites) – Appvance, IBM Rational Functional Tester, LEAPWORK, Sauce Labs, Selenium, SmartBear TestComplete, SOASTA TouchTest, Micro Focus Borland Silk Test
- CT – Tricentis Tosca
Analytics and AI can help
Test metrics isn’t a new concept, although with the data analytics capabilities modern tools include, there’s a lot more than can be measured and optimized beyond code coverage.
“You need to understand your code coverage as well as your test coverage. You need to understand what percentage of your APIs are actually tested and whether they’re completely tested or not because those APIs are being used in other applications as well,” said Theresa Lanowitz, founder and head analyst at market research firm www.vokeinc.com Voke. “The confidence level is important.”
Rex Blackpresident of testing training and consulting firm RBCS, said some of his clients have been perplexed by results that should indicate success when code quality still isn’t what it should be.
“One thing that happens is sometimes [there is] a unidimensional view of coverage, and I’ve seen this with clients where they say, ‘How could we possibly be missing defects when we’re achieving 100% statement coverage?’ and I have to explain you’re testing the code that’s there, but how about the code that should be there and isn’t?” said Black.
Similarly, there may be a big screen dashboard indicating that all automated tests have passed, so the assumption is all is good, but the dashboard is only reporting on the tests that have run, not what should be covered but hasn’t been covered.
Forrester analyst DIego Lo Giudice referenced a “continuous testing mobius loop” in which data is generated at each step that can be used to do “smarter things” like deciding what to test next.
“If you start using AI or machine learning, you can start to predict where the software may be more buggy or less buggy, or you can predict bugs and prevent them rather than finding the bugs and validating or verifying them,” said Lo Giudice. “On the other hand, it’s part of a broader picture that we call ‘value stream management’ where each type of stakeholder in the whole end-to-end delivery process has a view.”
Quality is one of the views. Other views enable a product owner to understand the costs of achieving a quality level, what has been released into production, how long it took and that the value it’s generating.
Sean Kenefick, VP, analyst at www.gartner.com Gartner said he’s currently working on a project that involves looking at AI and its relationship to software testing. Importantly, there are two ways to view that topic. One is testing systems that incorporate AI. The other is using AI as part of the testing effort.
“I think AIs are going to have quite a significant impact on automated testing because they’re going to allow us to solve some really thorny problems,” said Kenefick. “Some of my clients are in the video game space where, unlike an insurance package or a banking package that have right answers determined by banking regulations or GAAP, games are disconnected from the real world.”
For example, in a game, players may have wings that enable them to fly. Moreover, they may be able to fly at a speed that would dismember a physical body in the real world. Traditional automation tools have done a poor job of bridging the gap between fictional scenarios and the real-life expectations of humans, such as hair flapping in the wind. AI enhancement could help.
Automated testing is ripe for AI enhancement from a number of perspectives such as identifying tests that should be automated and suggesting new types of tests, but again, even with AI it may not be possible or even wise to automate all types of tests.
“We can automate functional testing at multiple levels to some extent, though there are some validation tests which may not be so easy to automate,” said Black. “At some point, we’ll have AIs that can do ethical hacker kinds of tests, but we don’t have that now, so if you want to do a penetration test, you need a human ethical hacker to do that.”
Localization testing tools are using machine learning to test translations. They’re not perfect yet, nor is Google Translate which is available via an API, so a human is still needed in the loop. Humans also need to be involved in accessibility testing and portability testing to some extent.
“You need to start with what do we need to test and if you let the test automation determine what your test coverage is going to be, that’s likely to make you sad,” said Black. “I made that mistake when I was an inexperienced test manager and it’s not a good thing. Identify all the things you need to cover and then figure out the best way to cover those things.”
Drive higher value with a test strategy
The inclusion of analytics, AI, and machine learning in tools is arguably “cool,” but the outcomes they help enable and the effectiveness of testing generally can be improved by having an overarching test strategy.
“This is a very big problem. Software engineering is notoriously fad-driven and hype-driven,” said RBCS’s Black. “I’ve worked with a number of clients who were heavily into automation and if you look at what they were doing, there was no strategy. I ask questions that are pretty obvious like, ‘What are the objectives you’re trying to accomplish? For each one of those objectives, show me how you’re measuring testing and efficiency, show me the business case for your automation. What’s your ROI? Without a business case, it’s not a tool, it’s a toy.”
There is a temptation to go to tools first and think later, when the reverse yields better results. Starting with tools results in a test strategy by default rather than test strategy by design.
“I think many people would say [that having a testing strategy] is an old-school approach, but it’s the right approach because if you’re just going with tool A or tool B, you’re trusting your approach to that tool and that tool may not provide what you need,” said Voke’s Lanowitz. “I think you need to take a step back and decide what you’re going to do.”
Ultimately, the testing strategy and execution need to tie together in a way that aligns with business objectives, but more fundamentally organizations have to cultivate a culture of quality in the first place if they want their CT efforts to be stable.
“Understand that nothing we’re doing can be 100%. What we’re really doing is trying to minimize our risk as much as possible and mitigate for things we weren’t expecting,” said RBCS’s Black. “Continuous delivery is a process that lives forever. As long as the product is alive, we’re testing it.