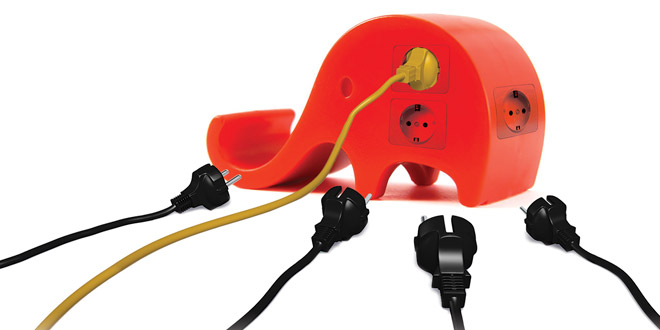
Even if it’s not where they end up, Hadoop can be a great starting platform for a data-driven software company. That’s what San Francisco- and Taipei-based Fliptop found in 2009 when they launched a social media identity matching engine, ultimately employed by such companies as MailChimp, Dell, Toyota, Oracle and Nordstrom. Based on Amazon Web Services’ implementation of Hadoop, Elastic Map Reduce, Fliptop’s initial approach used Ruby on Rails, Scala, Java, Apache Solr for search and Drools for business rules. But when market signals began to point elsewhere, experience with prior startups acquired by Bertelsmann and Symantec meant that founder Doug Camplejohn followed them.
“In 2012, we realized that while big companies were spending millions of dollars to build data science teams and custom data warehouses utilizing lots of expensive tools, most mid-market companies could not. So we pivoted. We built a suite of SaaS applications that leveraged internal and external data and data science for everyone—not just the data jocks,” Camplejohn said. Building a solution for predictive lead scoring, however, was a different kind of big data problem—one that Hadoop and Amazon’s Elastic Map Reduce couldn’t solve.
“When we were more a social data business, we were doing a lot of batch processing with Elastic Map Reduce. Large brands would give us a list of several 100 million email addresses, and we’d find social media profiles associated with those,” said Dan Chiao, Fliptop vice president of engineering. “Now, with predictive analytics, we have a large batch processing job to build an initial machine learning model, sucking in existing data in the CRM system. But the ongoing task is to score incoming leads as they come into Marketo. For that we do a lot of real-time processing on Apache Storm.”
Fliptop’s new technology stack is centered on Scala for its concise functional syntax, Java libraries, deployment architecture and Scala-specific libraries such as Dispatch and the Lift Web Framework. For streaming intake and normalization of massive data, they chose Storm, which had recently been open-sourced to Apache by Twitter. Finally, for their expanding data sources and continually morphing schemas, they chose the open-source MongoDB, which has “the most sophisticated query syntax of the NoSQL options,” according to Chiao. The solution was hosted on AWS and Azure.
The journey Fliptop has taken in the last five years isn’t unusual, as ISVs attempt to build on the Hadoop platform, mining the Big Data ecosystem for gems that make data science viable even without Hadoop expertise. With all the “data washing” hype now blurring the differences among business intelligence, predictive analytics and traditional databases, it’s time to gaze clearly upon this new “data operating system.”
When Hadoop doesn’t fit
What are you trying to gather and what will you do with it? Those are the first questions any data science project should ask itself. If you’re predicting utility power consumption, you may be dealing with smart meters that generate interval data, along with sensors that check voltage. Retailers may offer personalization based on facial recognition and biometrics, a la Minority Report. Or healthcare systems may look for fraudulent behavior in the form of anomalous data. Whether it’s transaction, historical, or master data will determine how you store and query it. How often you need to get to the data has implications for storage design and costs. Finally, whether you need to process it in real time or in batch mode determines much of your particular Big Data stack.
“I think of Hadoop as an operating system and not a database. It’s a database because it provides you with the ability to store and query data. But if you look at the architecture, it’s like an OS, because operating systems are pluggable. Today we have Pig, then Spark comes along. Like an OS, it allows us to develop on top of it,” said Eli Collins, chief technologist for Cloudera, the market-leading provider of Hadoop distributions and services.
And while major vendors from Oracle to Microsoft to IBM and Intel are incorporating Hadoop distributions from Apache, Cloudera and HortonWorks into their own big data offerings, it’s important to note that Hadoop isn’t one-size-fits-all. At the recent Dreamforce conference in San Francisco, plenty of vendors in the Salesforce ecosystem touted the data science behind their apps, but only some, like Fliptop’s early incarnation, used Hadoop as a data store. Popular alternatives include HBase, Cassandra and MongoDB, for varying reasons.
First, there’s size. Many data problems aren’t a question of volume, but more of velocity, variety or veracity. While a Hadoop cluster easily scales up to contend with massive amounts of unstructured data, MongoDB is a NoSQL alternative that functions well on several terabytes of data, but may run into limitations after that point. Another option is Apache HBase, whose flexible data model is good for quickly nabbing small stats within large columnar data sets. And many are fond of the lesser-known Cassandra, another NoSQL, fault-tolerant database written in Java, popular for storing huge machine-to-machine data sets or transaction logging. Accumulo is an interesting option offering cell-level security. There are other flavors, each with pros and cons: Couchbase, Riak and Redis, to name a few.
“One technology people use today is NoSQL databases, because NoSQL is designed to be highly scalable. Companies like Uber, in last two years or so, built an architecture with a huge, huge set of NoSQL databases because of their access to mobile content. On the back-end is Cassandra, then there’s Teradata. In the middle is Talend, tying it all together,” said Ciaran Dynes, Dublin, Ireland-based vice president of product at Talend, a French master data management company and enterprise service bus provider.
Cost considerations
Hadoop’s success comes from the power to massively distribute compute clusters on commodity hardware, while storage costs in the cloud have simultaneously plummeted.
“The economic argument for Hadoop is, in the old data warehousing days, $40,000 per terabyte of data was the traditional cost. Now we’re talking about $1,000 per terabyte for Hadoop. That’s a 40x savings, if you ignore the fact that data is growing. So with growing data, you may need something alongside your Teradata data warehouse. You may be legally required to archive information. Hadoop is almost at the same price point as it would have been for the old archive technology, but Hadoop is a data processing platform whereas tape isn’t. Hadoop gets you best of both worlds, and maybe some data scientist helps you find some lost or hidden gems,” said Dynes.
But there are still costs associated with Hadoop, depending on how it is set up, tuned and supported. Microsoft’s HDInsight, comprising Hortonworks Hadoop ported to the 64-bit version of Windows Server 2012 R2 and supporting the .NET Framework, also offers Azure Blob storage for unstructured data. Blob storage has advantages over the Hadoop Distributed File System (HDFS): it’s accessible, via REST APIs, to more applications and other HDInsight clusters; it’s an archive to the HDFS data lake (as redundant as that sounds;) it’s a cheaper storage container than the Hadoop compute cluster and cuts data loading costs; it has automatic elastic scaling that can be less complex than HDFS node provisioning; and it’s geo-replicated, if need be (though that’s more expensive.)
And what about personnel? Hortonworks argues that the Hadoop learning curve is nontrivial—and, these days, non-essential: “New world companies that are data-driven from day one may have a team of 20 guys using Hadoop and managing their own Hadoop cluster. They don’t realize, ‘Hey I don’t need to do this anymore. The Hadoop thing isn’t the differentiator, it’s what I’m doing with it that’s the differentiator,” said Jim Walker, director of product marketing at Hortonworks, which sells enterprise Hadoop support while proudly proclaiming its status as the 100 percent open-source provider of Hadoop distributions.
It’s in the box
Oracle makes the same argument with its Oracle Big Data Appliance X3-2, based on the Sun X3-2L servers, a commodity X86 server for distributed computing. “Many people ask ‘Why should any organization buy a Hadoop appliance?’ While this is a valid question at first glance, the question should really be ‘Why would anyone want to build a Hadoop cluster themselves?’ Building a Hadoop cluster for anything beyond a small cluster is not trivial,” according to the Oracle Big Data Handbook (Oracle Press, September 2013.) The process of building a large Hadoop cluster is closer to erecting a skyscraper, the book contends—thus best left to experts. Economically, too, Oracle makes the argument that its Big Data Appliance list price of $450,000 plus $54,000 per year of maintenance is ultimately cheaper than building an 18-node cluster on your own.
Like Oracle, IBM is no newcomer to database technology. InfoSphere BigInsights melds its expertise in relational databases, SPSS advanced analytics, grid computing, modeling and distributed data crunching with Hadoop. The ubiquity of IBM and Oracle solutions isn’t a legacy: it’s a gold mine. Historic data expertise can’t be downplayed as enterprises seek to define new types of enterprise data hubs via Hadoop, especially since valuable data, once discovered, is likely to be propagated back into the enterprise data warehouse.
Twenty years ago, Oracle’s decision support systems or data warehouses “became engines for terabyte-sized data warehouses. Today Oracle-based data warehouses have grown into the petabytes thanks to many further improvements in the Oracle Database and the introduction of engineered systems such as the Oracle Exadata Database Machine,” according to the Oracle Big Data Handbook. Why does this matter? Because, “Over time, the functionality mix will increasingly overlap in each as more Hadoop capabilities appear in relational databases and more relational database capabilities appear in Hadoop,” the handbook says. Thus the performance enhancements and redundancy of traditional data warehouses may be useful in hybrid applications, which are likely to be the norm.
Who asks the questions?
The explosion of interest in data science isn’t limited to quants. “There are different end-user communities for Hadoop. A data scientist or analyst might want to use SAS, R, Python—those are for writing programs. There’s another community from the DBA world, which is using SQL or a business intelligence tool that uses SQL data sources. Then there’s the ETL community, which has traditionally done transforms via graphical tools. They’re using third-party drag-and-drop—reporting software is in there too. Finally, the platform has gotten easier for non-analysts to use, and you can use free-text search to inspect the data. Splunk is the leader there; their product, Hunk, can run directly on Hadoop. For each of these end-user communities, there’s a different set of tools,” said Collins.
As the technology stack has evolved to meet the demands of Hadoop users, the concept of storing everything has grown commonplace. That’s due in part, according to Hortonworks, to new abilities to retrieve and make sense of data coming from industrial sensors, web traffic and other sources. In Hadoop 2.0, YARN replaces the classic Hadoop MapReduce framework. It’s a new application management framework—the Hadoop operating system—and it improves scalability and cluster performance.
“We saw a major shift on October 23 last year with the introduction of YARN. That’s why you heard the terms ‘data lake,’ ‘data reservoir,’” said Hortonworks’ Walker. As a result of YARN, Hadoop has become an architectural center serving many needs, Walker explained. “Spark, Hive—all these things live in the Hadoop world because of the technology of YARN. If my applications need SQL access, I’ll use Hive. If I’m doing machine learning and data science, I’ll use Spark,” Walker continued.
Python pulls ahead
Today, every element of the Apache Hadoop stack—Ambari for provisioning clusters, Avro for data serialization, Cassandra for scalable multi-master databases, Chukwa for data collection, HBase for large table storage, Hive for adhoc querying, Mahout for machine learning and recommendation engines, Pig for programming parallel computation, Spark for ETL and stream processing, Tez for programming data tasks, and ZooKeeper, for coordinating distributed applications—has several contenders vying for users and tackling precise niches.
Take Mahout, a library of scalable machine-learning algorithms named after the Indian term for an elephant rider. Microsoft Azure users, among others, will find this pre-installed on HDInsight 3.1 clusters. But it may not be production-ready, depending on your needs.
“We tested Mahout, but we didn’t get good results,” said Fliptop’s Chiao. “It had to do with the fact that it only had skeleton implementations of some sophisticated machine learning techniques we wanted to use, like Random Forest and Adaboost.” In its stead, Fliptop chose Python-based scikit-learn, a mature, actively developed, sophisticated and production-grade machine learning library that has been around since 2006 and, Fliptop decided, had comparable performance to R.
“This is a great time to be a data scientist or data engineer who relies on Python or R,” blogged Ben Lorica, chief data scientist at O’Reilly Media. Python and R code can run on a variety of increasingly scalable execution engines such as GraphLab, wise.io, Adatao, H20, Skytree and Revolution R. Speculation swirled in September 2014 when Cloudera acquired Datapad, a company that comes with co-founder Wes McKinney, creator of the Python-based Pandas data analysis library.
“I don’t think of Python as tooling. But there’s been a ton of interest in the Python community around integrating with Hadoop—there’s a large community there. We published an overview of 19 Python frameworks built around Hadoop a few months ago, and that was our number one or two most popular blog post ever,” said Collins.
Security still a step-child
New features and sparkly storytelling are all the rage, but it seems, at least among users, that security still gets short shrift. An integration vendor recently explained, under cover of anonymity, that security had not even been a concern for his company, which made tools to pass data from IoT devices such as thermostats and sprinkler systems to cloud data stores: “That’s what we depend on Hadoop and Amazon to do,” the vendor said.
Thankfully, Hortonworks and others are acting quickly to fill this gap in the stack. XA Secure, which Hortonworks purchased in May 2014, was the source for code subsequently contributed to the Apache Software Foundation and now incubating under the name Apache Argus. “It’s a UI to create a single holistic security policy across all engines,” explained Walker. “It needs to reach down into YARN and HDFS. So we’re trying to get ahead of that—we made the first security acquisition in this space.”
For its part, in June 2014, Cloudera acquired Gazzang for enterprise-grade data encryption and key management. Last year, the company delivered Apache Sentry, which is incubating. Another security project is Project Rhino—an open source effort founded by Intel in 2013 to provide perimeter security, entitlements and access control and data protection.
“We now have a 30-person security center of excellence team as part of our partnership with Intel. If we don’t solve the security problems, we don’t get the benefits of the data. One thing is new here, with the data hub methodology: You can’t onboard new users if you don’t solve the security problem,” said Collins.
Not surprisingly, relational database market leader Oracle has incorporated Sentry, LDAP-based authorization and support for Kerberos authentication in its Big Data Appliance. Oracle also alerts admins to suspicious activities happening in Hadoop via its Audit Vault and Database Firewall.
Ironically, some early adopters in retail and finance haven’t feared the lack of data-level security protocols—they’ve simply built it themselves. Square, the mobile credit card payment processing company, stores some customer data in Hadoop, but wiggles out the security and privacy quandaries by redacting the data. However, other data isn’t so harmless, and must be protected. After looking at multiple options, including a separate, secure Hadoop cluster, Square crafted an encryption model for its data stored in protobufs format using an online crypto service, maintaining keys separate from data, controlling cell-level access, using security to cover the incoming data as well as that being stored. Though the end product was successful, “The lack of data-level security is keeping relevant projects off of Hadoop,” blogged Ben Spivey, a solutions architect at Cloudera, and Joey Echeverria, a Cloudera software engineer.
Flint, meet steel
Heating up the Hadoop community are real-time data processing options such as the nascent Spark, which emerged from the famed UC Berkeley Amp Lab, home of the Berkeley Data Analytics Stack. Akka, Finagle and Storm, which run on the JVM and work with Java and Scala, are bringing the power of concurrent programming to the masses. Google is continually innovating new tools, such as Dataflow, intended to supplant the obtuse MapReduce model. Interestingly, the entire Hadoop ecosystem points to mainstream success of parallel programming in a world of exponentially increasing data and shrinking silicon real estate. With Hadoop as the new data OS, the abstraction layer continually rises. The wisdom of clusters wins.
Still, moderation is key. “Don’t let the digital obscure the analog,” warned Peter Coffee in his keynote at the 2014 Dreamforce conference in San Francisco. “All modes of information, those are part of the new business too: The expression on someone’s face, the feel of someone’s handshake.” Patterns in Big Data only emerge because there are these networks of interaction, and those networks can only arise because people voluntarily connect, he argued.
“If you parachuted a bunch of consultants with clipboards into the Philippines and said ‘We’re going to build you a disaster preparedness system for $5 million that no one trusts and no one uses’—that sounds like the old IT, doesn’t it? The new IT is to take the behavior people enthusiastically and voluntarily engage in and use it to find the emergent opportunities.”
Your big data project: What could go wrong?
Answer: Plenty.
In 2012, researchers Danah Boyd and Kate Crawford published the paper “Critical Questions for Big Data: Provocations for a Cultural, Technological, and Scholarly Phenomenon.” Among their warnings are the following:
1.“Big Data changes the definition of knowledge.”
2.“Claims to objectivity and accuracy are misleading.” Subjective interpretation and “storytelling” as well as data loss and noise, will always affect results.
3.“Bigger data are not always better data.” The more data
there is, the greater the need for quality sources and statistical rigor.
4.“Taken out of context, Big Data loses its meaning.”
5.“Just because it is accessible does not make it ethical.” Privacy protection is also imperfect, as anonymized records can be reconstituted.
6.“Limited access to Big Data creates new digital divides.”
But wait, there’s much more where that came from. What about asking the wrong questions? Data scientists may be born quants, but they’re unfamiliar with the business domain—which is more important than knowing Hadoop. And what about asking questions companies don’t really want to know the answers to, such as Twitter sentiment analysis that might require compliance reporting of side effects to the FDA?
Speaking of governance, that, along with privacy, metadata management and security, presents a real risk to enterprise big data efforts. Privacy regulations to be cognizant of include:
• The USA Patriot Act’s KYC (Know Your Customer) provision
• The Gramm-Leach-Bliley Act for protecting consumers’ personal financial information
• The UK Financial Services Act
• DNC compliance
• Consumer Proprietary Network Information (CPNI)
compliance
• HIPPA
• The European Union Data Protection Directive
• The Japanese Protection for Personal Information Act
• The Federal Trade Commission, 16 CFR Part 314—safeguarding customer information
And then there are the usual problems associated with any IT effort: skill, scope and context.
A recent survey conducted by Infochimps found that “Lack of Business Context Around the Data” (51 percent), “Lack of Expertise to Connect the Dots” (51 percent), and “Inaccurate Scope” (58 percent) were the top reasons big data projects fail.